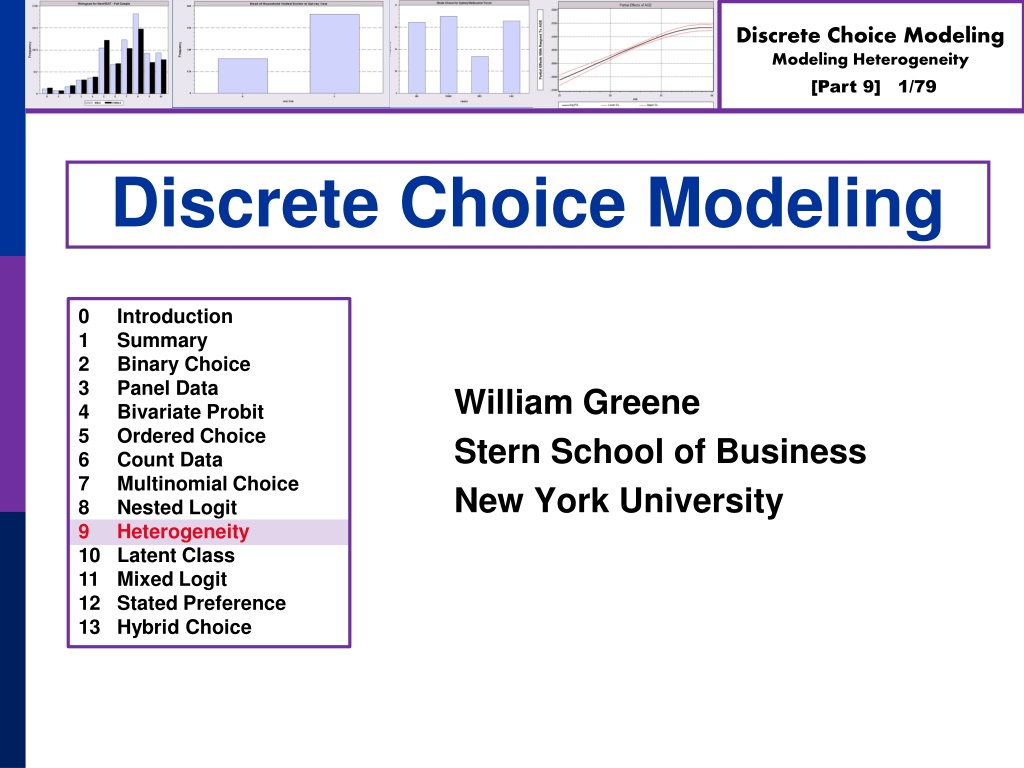
Understanding Heterogeneity in Discrete Choice Modeling
Explore the nuances of heterogeneity in discrete choice modeling, including types of heterogeneity, challenges with the MNL model, ways to accommodate heterogeneity, and limitations of current modeling approaches. Discover how differences across choice makers impact decision strategies and preference structures in modeling.
Download Presentation

Please find below an Image/Link to download the presentation.
The content on the website is provided AS IS for your information and personal use only. It may not be sold, licensed, or shared on other websites without obtaining consent from the author. If you encounter any issues during the download, it is possible that the publisher has removed the file from their server.
You are allowed to download the files provided on this website for personal or commercial use, subject to the condition that they are used lawfully. All files are the property of their respective owners.
The content on the website is provided AS IS for your information and personal use only. It may not be sold, licensed, or shared on other websites without obtaining consent from the author.
E N D
Presentation Transcript
Discrete Choice Modeling Modeling Heterogeneity [Part 9] 1/79 Discrete Choice Modeling 0 1 2 3 4 5 6 7 8 9 10 Latent Class 11 Mixed Logit 12 Stated Preference 13 Hybrid Choice Introduction Summary Binary Choice Panel Data Bivariate Probit Ordered Choice Count Data Multinomial Choice Nested Logit Heterogeneity William Greene Stern School of Business New York University
Discrete Choice Modeling Modeling Heterogeneity [Part 9] 2/79 What s Wrong with the MNL Model? Insufficiently heterogeneous: economists are often more interested in aggregate effects and regard heterogeneity as a statistical nuisance parameter problem which must be addressed but not emphasized. Econometricians frequently employ methods which do not allow for the estimation of individual level parameters. (Allenby and Rossi, Journal of Econometrics, 1999)
Discrete Choice Modeling Modeling Heterogeneity [Part 9] 3/79 Several Types of Heterogeneity Differences across choice makers Observable: Usually demographics such as age, sex Unobservable: Usually modeled as random effects Choice strategy: How consumers make decisions. (E.g., omitted attributes) Preference Structure: Model frameworks such as latent class structures Preferences:Model parameters Discrete variation latent class Continuous variation mixed models Discrete-Continuous variation
Discrete Choice Modeling Modeling Heterogeneity [Part 9] 4/79 Heterogeneity in Choice Strategy Consumers avoid complexity Lexicographic preferences eliminate certain choices choice set may be endogenously determined Simplification strategies may eliminate certain attributes Information processing strategy is a source of heterogeneity in the model.
Discrete Choice Modeling Modeling Heterogeneity [Part 9] 5/79 Accommodating Heterogeneity Observed? Enter in the model in familiar (and unfamiliar) ways. Unobserved? Takes the form of randomness in the model.
Discrete Choice Modeling Modeling Heterogeneity [Part 9] 6/79 Heterogeneity and the MNL Model exp( + ' x ) j itj P[choice j|i,t]= J(i) exp( + ' x ) j itj j=1 Limitations of the MNL Model: IID IIA Fundamental tastes are the same across all individuals How to adjust the model to allow variation across individuals? Full random variation Latent grouping allow some variation
Discrete Choice Modeling Modeling Heterogeneity [Part 9] 7/79 Observable Heterogeneity in Utility Levels j U = + ' x + z + ijt j itj it ijt z j exp( + 'x + ) j itj it Prob[choice j|i,t]= J (i) exp( + 'x + z ) t j itj it j=1 Choice, e.g., among brands of cars xitj = attributes: price, features zit = observable characteristics: age, sex, income
Discrete Choice Modeling Modeling Heterogeneity [Part 9] 8/79 Observable Heterogeneity in Preference Weights Hierarchical model - Interaction terms U = + = + Parameter heterogeneity is observable. Each parameter = + i j x + z + ijt j itj it ijt h i i k h i,k k i x i j exp( + + z ) j itj it Prob[choice j|i ,t]= J (i) i exp( + x + z ) t j itj it j=1
Discrete Choice Modeling Modeling Heterogeneity [Part 9] 9/79 Heteroscedasticity in the MNL Model Motivation: Scaling in utility functions If ignored, distorts coefficients Random utility basis Uij = j + xij + zi+ j ij i =1, ,N; j = 1, ,J(i) F( ij) = Exp(-Exp(- ij)) now scaled Extensions: Relaxes IIA Allows heteroscedasticity across choices and across individuals
Discrete Choice Modeling Modeling Heterogeneity [Part 9] 10/79 Quantifiable Heterogeneity in Scaling j U = + 'x + z + ijt j itj w it ijt j 2 j 2 1 2 Var[ ]= exp( ), = /6 ijt i wi = observable characteristics: age, sex, income, etc.
Discrete Choice Modeling Modeling Heterogeneity [Part 9] 11/79 Modeling Unobserved Heterogeneity Latent class Discrete approximation Mixed logit Continuous Many extensions and blends of LC and RP
Discrete Choice Modeling Modeling Heterogeneity [Part 9] 12/79 LATENT CLASS MODELS
Discrete Choice Modeling Modeling Heterogeneity [Part 9] 13/79 The Finite Mixture Model An unknown parametric model governs an outcome y F(y|x, ) This is the model We approximate F(y|x, ) with a weighted sum of specified (e.g., normal) densities: F(y|x, ) j j G(y|x, ) This is a search for functional form. With a sufficient number of (normal) components, we can approximate any density to any desired degree of accuracy. (McLachlan and Peel (2000)) There is no mixing process at work
Discrete Choice Modeling Modeling Heterogeneity [Part 9] 14/79 Density? Note significant mass below zero. Not a gamma or lognormal or any other familiar density.
Discrete Choice Modeling Modeling Heterogeneity [Part 9] 15/79 ML Mixture of Two Normal Densities Maximum Likelihood Estimates Class 1 Class 2 Estimate Std. Error Estimate Std. error 7.05737 .77151 3.25966 .09824 3.79628 .25395 1.81941 .10858 .28547 .05953 .71453 .05953 y - 1 2 1000 i j LogL = log j i=1 j=1 j j 1 y-7.05737 3.79628 1 y-3.25966 1.81941 F(y)=.28547 +.71453 3.79628 1.81941
Discrete Choice Modeling Modeling Heterogeneity [Part 9] 16/79 Mixing probabilities .715 and .285
Discrete Choice Modeling Modeling Heterogeneity [Part 9] 17/79 The actual process is a mix of chi squared(5) and normal(3,2) with mixing probabilities .7 and .3. 2.5 1.5 .5 exp( .5 ) (2.5) 1 2 3 y y y = + ( ) f y .7 .3 2
Discrete Choice Modeling Modeling Heterogeneity [Part 9] 18/79 Approximation Actual Distribution
Discrete Choice Modeling Modeling Heterogeneity [Part 9] 19/79 Latent Classes Population contains a mixture of individuals of different types Common form of the generating mechanism within the classes Observed outcome y is governed by the common process F(y|x, j) Classes are distinguished by the parameters, j.
Discrete Choice Modeling Modeling Heterogeneity [Part 9] 20/79
Discrete Choice Modeling Modeling Heterogeneity [Part 9] 21/79 The Latent Class Model Parametric Model: F(y|x, ) E.g., y ~ N[x , 2], y ~ Poisson[ =exp(x )], etc. Density F(y|x, ) j j F(y|x, j), = [ 1, 2, , J, 1, 2, , J] j j = 1 Generating mechanism for an individual drawn at random from the mixed population is F(y|x, ). Class probabilities relate to a stable process governing the mixture of types in the population
Discrete Choice Modeling Modeling Heterogeneity [Part 9] 22/79
Discrete Choice Modeling Modeling Heterogeneity [Part 9] 23/79
Discrete Choice Modeling Modeling Heterogeneity [Part 9] 24/79 RANDOM PARAMETER MODELS
Discrete Choice Modeling Modeling Heterogeneity [Part 9] 25/79 A Recast Random Effects Model + + , ~ = = + i i u + x [0, ] U u u N it it i it i u T = observations on individual i For each period, Joint probability for T observations is i = 1[ 0] (given u ) y U i it it i N T = + x Prob( , ,...| ) ( = + i ) y y u F y i 1 2 , i i i it i it = 1 t Write = , ~ [0,1], u v v v u u i i i i N T = + + x log | ,... L v log ( ( ) ) v F y v i 1 , u N it i it = = 1 i i t It is not possible to maximize log | ,... the unobserved random effects embedded in . because of i L v v 1 N
Discrete Choice Modeling Modeling Heterogeneity [Part 9] 26/79 A Computable Log Likelihood v The unobserved heterogeneity is averaged out of l og | L = ( ) T N + | ] v x [log log ( , ) E L F y f d i = v it i it i i = 1 i 1 t i = + Maximize this function with respect to , , How to compute the in ( 1 ) Analytically? No, no formula exists. (2) Approximately, using Gauss-Hermite quadrature (3) Approximately using Monte Carlo simulation . ( ) u i v u i tegral?
Discrete Choice Modeling Modeling Heterogeneity [Part 9] 27/79 Simulation ( ) T N = + i logL log F(y , x ) d i it it i i = i 1 = t 1 2 2 - 1 2 N = log g( ) exp d i i i = i 1 N This The expected value of the function of by dra w in g a R r ndom draws v from the population N[0,1] and averaging the R functio ns of equal s log E [ g ( ) ] i = i 1 can be a p proxima ted i i r = t 1 + v . We maximi ze ir u ir 1 R iT N R = + + logL log F (y ,( v ) x ) S it u ir it = i 1 = r 1 = (We did this in part 4 for th e random effects pro bit model.)
Discrete Choice Modeling Modeling Heterogeneity [Part 9] 28/79 Random Effects Model: Simulation ---------------------------------------------------------------------- Random Coefficients Probit Model Dependent variable DOCTOR (Quadrature Based) Log likelihood function -16296.68110 (-16290.72192) Restricted log likelihood -17701.08500 Chi squared [ 1 d.f.] 2808.80780 Simulation based on 50 Halton draws --------+------------------------------------------------- Variable| Coefficient Standard Error b/St.Er. P[|Z|>z] --------+------------------------------------------------- |Nonrandom parameters AGE| .02226*** .00081 27.365 .0000 ( .02232) EDUC| -.03285*** .00391 -8.407 .0000 (-.03307) HHNINC| .00673 .05105 .132 .8952 ( .00660) |Means for random parameters Constant| -.11873** .05950 -1.995 .0460 (-.11819) |Scale parameters for dists. of random parameters Constant| .90453*** .01128 80.180 .0000 --------+------------------------------------------------------------- Implied from these estimates is .904542/(1+.904532) = .449998.
Discrete Choice Modeling Modeling Heterogeneity [Part 9] 29/79 The Entire Parameter Vector is Random i = u x + , , U i t it it = + u [ , 0 ~ ( ,..., )] N diag i i 1 i K Joint probability for observations is T i T i = , x Prob( , ,...| ) ( ) y y u F y i = 1 2 i i i it v it 1 t = + For convenience, write = , ~ [0,1], v u v N v ik k ik ik ik k k ik iT N i = , x log | ,.. L v . log ( ) v F y 1 N it it i i = = 1 t v It is not possible to maximize log the unobserved random effects embedded in . | ,... because of L 1 N i
Discrete Choice Modeling Modeling Heterogeneity [Part 9] 30/79 Estimating the RPL Model Estimation: 1 2it = 2 + zi + vi,t (1) Estimate structural parameters (2) Estimate individual specific utility parameters (3) Estimate elasticities, etc. Uncorrelated: is diagonal Autocorrelated: vi,t = Rvi,t-1 + ui,t
Discrete Choice Modeling Modeling Heterogeneity [Part 9] 31/79 Classical Estimation Platform: The Likelihood Marginal: f( Population Mean=E[ |data, ) i |data, ] i = f( | )d i i i i = = a subvector of = Argmax L( ,i=1,...,N|data, ) i Estimator = Expected value over all possible realizations of i. I.e., over all possible samples.
Discrete Choice Modeling Modeling Heterogeneity [Part 9] 32/79 Simulation Based Estimation Choice probability = P[data | ( 1, 2, , ,R,vi,t)] Need to integrate out the unobserved random term E{P[data | ( 1, 2, , ,R,vi,t)]} = P[ |vi,t]f(vi,t)dvi,t Integration is done by simulation Draw values of v and compute then probabilities Average many draws Maximize the sum of the logs of the averages (See Train[Cambridge, 2003] on simulation methods.) v
Discrete Choice Modeling Modeling Heterogeneity [Part 9] 33/79 Maximum Simulated Likelihood True log likelihood T L ( |data )= f(data | ) i i i i i i t=1 T L ( |data )= f(data | )f( | )d i i i i i i i t=1 i N logL = log L ( |data )f( | )d i i i i i i=1 i Simulated log likelihood 1 R N R logL = log L ( |data, ) S i iR i i=1 r=1 =argmax(logL ) S
Discrete Choice Modeling Modeling Heterogeneity [Part 9] 34/79
Discrete Choice Modeling Modeling Heterogeneity [Part 9] 35/79 u When Var[ ] is not a diagonal matrix. How to estimate a positive definite matrix, . ~ N[ , ] = with ~ N[ , ] Convenient Refinement: = elements of matrix with free positive eleme = = M I i u 0 i Cholesky Decomposi u Lv t n i 0 o : I LL where is upper triangular L = v i i i = LL MS MS ( M ) where the diagonal equal 1, and is the diagonal S )( nts. (Cholesky values) u MSv i i returns the original uncorrelated case. We used the Cholesky decomposition in developing the Krinsky and Robb method for standard errors for partial effects, in Part 3.
Discrete Choice Modeling Modeling Heterogeneity [Part 9] 36/79 S M
Discrete Choice Modeling Modeling Heterogeneity [Part 9] 37/79 MSS M
Discrete Choice Modeling Modeling Heterogeneity [Part 9] 38/79 Modeling Parameter Heterogeneity of the parameters Individual heterogeneity in the means + , i = z u X z + u i i i E[ Heterogeneity in the variances | ] i i of the parameters i = Var[u |data] Estimation by maximum simulated likelihood exp( h ) i,k i k k
Discrete Choice Modeling Modeling Heterogeneity [Part 9] 39/79 A Hierarchical Probit Model Uit = 1i + 2iAgeit + 3iEducit + 4iIncomeit + it. 1i= 1+ 11 Femalei + 12 Marriedi + u1i 2i= 2+ 21 Femalei + 22 Marriedi + u2i 3i= 3+ 31 Femalei + 32 Marriedi + u3i 4i= 4+ 41 Femalei + 42 Marriedi + u4i Yit = 1[Uit > 0] All random variables normally distributed.
Discrete Choice Modeling Modeling Heterogeneity [Part 9] 40/79
Discrete Choice Modeling Modeling Heterogeneity [Part 9] 41/79 Simulating Conditional Means for Individual Parameters ( ( ) ) 1 R T R + + Lw Lw x ( ) (2 1)( ) y i = , , i r it i r it = 1 r 1 t ( E = y X | , ) 1 R i i i T R + Lw x (2 1)( ) y i = , it i r it = 1 r 1 t 1 R R = Weight ir ir = 1 r Posterior estimates of E[parameters(i) | Data(i)]
Discrete Choice Modeling Modeling Heterogeneity [Part 9] 42/79
Discrete Choice Modeling Modeling Heterogeneity [Part 9] 43/79
Discrete Choice Modeling Modeling Heterogeneity [Part 9] 44/79
Discrete Choice Modeling Modeling Heterogeneity [Part 9] 45/79 Individual Coefficients
Discrete Choice Modeling Modeling Heterogeneity [Part 9] 46/79 Programs differ on the models fitted, the algorithms, the paradigm, and the extensions provided to the simplest RPM, i = +wi. WinBUGS: MCMC User specifies the model constructs the Gibbs Sampler/Metropolis Hastings MLWin: Linear and some nonlinear logit, Poisson, etc. Uses MCMC for MLE (noninformative priors) SAS: Proc Mixed. Classical Uses primarily a kind of GLS/GMM (method of moments algorithm for loglinear models) Stata: Classical Several loglinear models GLAMM. Mixing done by quadrature. Maximum simulated likelihood for multinomial choice (Arne Hole, user provided) LIMDEP/NLOGIT Classical Mixing done by Monte Carlo integration maximum simulated likelihood Numerous linear, nonlinear, loglinear models Ken Train s Gauss Code, miscellaneous freelance R and Matlab code Monte Carlo integration Mixed Logit (mixed multinomial logit) model only (but free!) Biogeme Multinomial choice models Many experimental models (developer s hobby)
Discrete Choice Modeling Modeling Heterogeneity [Part 9] 47/79 SCALING IN CHOICE MODELS
Discrete Choice Modeling Modeling Heterogeneity [Part 9] 48/79 Using Degenerate Branches to Reveal Scaling LIMB Travel Fly BRANCH Rail Drive GrndPblc TWIG Air Train Car Bus
Discrete Choice Modeling Modeling Heterogeneity [Part 9] 49/79 Scaling in Transport Modes ----------------------------------------------------------- FIML Nested Multinomial Logit Model Dependent variable MODE Log likelihood function -182.42834 The model has 2 levels. Nested Logit form:IVparms=Taub|l,r,Sl|r & Fr.No normalizations imposed a priori Number of obs.= 210, skipped 0 obs --------+-------------------------------------------------- Variable| Coefficient Standard Error b/St.Er. P[|Z|>z] --------+-------------------------------------------------- |Attributes in the Utility Functions (beta) GC| .09622** .03875 2.483 .0130 TTME| -.08331*** .02697 -3.089 .0020 INVT| -.01888*** .00684 -2.760 .0058 INVC| -.10904*** .03677 -2.966 .0030 A_AIR| 4.50827*** 1.33062 3.388 .0007 A_TRAIN| 3.35580*** .90490 3.708 .0002 A_BUS| 3.11885** 1.33138 2.343 .0192 |IV parameters, tau(b|l,r),sigma(l|r),phi(r) FLY| 1.65512** .79212 2.089 .0367 RAIL| .92758*** .11822 7.846 .0000 LOCLMASS| 1.00787*** .15131 6.661 .0000 DRIVE| 1.00000 ......(Fixed Parameter)...... --------+-------------------------------------------------- NLOGIT ; Lhs=mode ; Rhs=gc,ttme,invt,invc,one ; Choices=air,train,bus,car ; Tree=Fly(Air), Rail(train), LoclMass(bus), Drive(Car) ; ivset:(drive)=[1]$
Discrete Choice Modeling Modeling Heterogeneity [Part 9] 50/79 A Model with Choice Heteroscedasticity = U( i ,t,j) + 'x + ' + z j itj j it j i,t,j F( IID after scaling by a choice specific scale parameter P[choice = j| , ,i,t] = Prob[U x z )) ) =exp(-exp(- i,t,j i,t,j U k = 1,...,J(i,t ) ], itj it i,t,j exp( + ' i,t,k exp ( + 'x + z ' )/ j itj j it j = J(i,t) x + z ' )/ j itj j it j j=1 Normalization required as only ratios can be estimated; =1 for one of the alternativ (Remember the integrability problem - scale is not identified.) es j