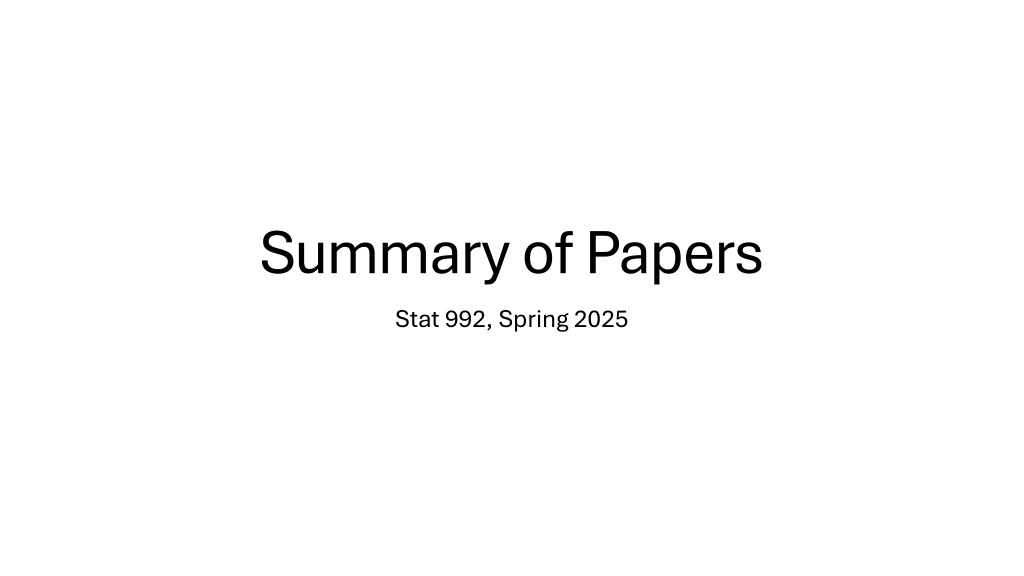
Causal Inference Research Areas and Projects Overview
Explore a comprehensive overview of research areas in causal inference, including unmeasured confounding, network analysis, psychometrics, transfer learning, and more. Delve into notable papers and projects, such as sensitivity analysis in genomic experiments and causal inference with latent variables. Engage with cutting-edge topics at the intersection of causal inference and diverse fields to enrich understanding and applications.
Download Presentation

Please find below an Image/Link to download the presentation.
The content on the website is provided AS IS for your information and personal use only. It may not be sold, licensed, or shared on other websites without obtaining consent from the author. If you encounter any issues during the download, it is possible that the publisher has removed the file from their server.
You are allowed to download the files provided on this website for personal or commercial use, subject to the condition that they are used lawfully. All files are the property of their respective owners.
The content on the website is provided AS IS for your information and personal use only. It may not be sold, licensed, or shared on other websites without obtaining consent from the author.
E N D
Presentation Transcript
Summary of Papers Stat 992, Spring 2025
Overview of Todays Lecture I ll provide a one-slide summary of the papers that represent research areas that I am currently working on I ve also listed my 4th and 5thyear Ph.D. students who are working in each area. In general, I would be happy to work on various topics in or related to causal inference (e.g., sampling, missing data, etc.) These days, I m broadly interested in fusing ideas from causal inference and other fields in ways that enrich both fields (i.e., Causal Inference + X) Design and analysis of Perturb-seq experiments in genomics Network analysis, spatial point processes, and study of dependence Psychometrics and latent variable modeling Transfer learning, optimization, optimal transport regression, etc.
Papers by Topic/Project Unmeasured confounding, sensitivity analysis, and application to genome-wide, Perturb-Seq experiments Rosenbaum (1987) The Role of a Second Control Group in an Observational Study. Stat. Sci. Zhao, Small, Bhattacharya (2019) Sensitivity analysis for inverse probability weighting estimators via the percentile bootstrap. JRSS:B. Du, Zeng, Kennedy, Wasserman, Roeder (2024) Causal Inference for Genomic Data with Multiple Heterogeneous Outcomes. arXiv. Talk to Jingqi Duan* (5thyear), Kwangmoon Park* (5thyear), Zhongxuan Sun* (2ndyear), Elaine Chiu (4thyear), and Xinran Miao (4thyear) to learn more. *: either advised by or jointly advised with Prof. Sunduz Keles
Papers by Topic/Project Causal inference with network/spatial/dependent data and applications to geosciences Li, Wager (2022) Random graph asymptotics for treatment effect estimation under network interference. AoS. Son, Reich, Schliep, Yang, Gill. Spatial causal inference in the presence of preferential sampling to study the impacts of marine protected areas. arXiv. Talk to Xindi Lin (4thyear) to learn more. Generalizability, transfer learning and causal inference Dahabreh, Robertson, Steingrimsson, Stuart, Hern n (2020) Extending inferences from a randomized trial to a new target population. Stat. Med. Li, Luedtke (2023) Efficient estimation under data fusion. Biometrika. Talk to Xinran Miao (4thyear) to learn more.
Papers by Topic/Project Causal inference with outcomes generated from latent variables and applications to psychometrics Stoetzer, Zhou, Steenbergen (2024) Causal inference with latent outcomes. American Journal of Political Science. Causal inference with surrogate (i.e., intermediate) outcomes Kallus, Mao (2024) On the role of surrogates in the efficient estimation of treatment effects with limited outcome data. arXiv. Other projects Causal inference with a continuous treatment and sensitivity analysis (ask Elaine Chiu) Optimal matching, randomization inference, and sampling from a finite population. Multi-sample instrumental variables and Mendelian randomization
Rosenbaum (1987) The Role of a Second Control Group in an Observational Study. Stat. Sci. Problem: study the average treatment effect (ATE) in the presence of unmeasured confounding. Solution: consider two types of control groups, both of which are not exposed to treatment. Example: the effect of AP programs in high school on college achievement Treated group: students who are enrolled in the AP program Control group A: students who are are offered the AP program, but declined to enroll Control group B: students who are not offered the AP program Use the two control groups to Test whether the treatment can be adjusted by measured variables only (X-adjustable) Bound the ATE
Zhao et al. (2019) Sensitivity analysis for inverse probability weighting... JRSS:B. Problem: conduct sensitivity analysis of the ATE in the presence of unmeasured confounding Solution: a marginal sensitivity model that compare the observed propensity score P(A=1|X) to the counterfactual propensity score P(A = 1|X,Y(a)) Consider all models of P(A=1 | X,Y(a)) that are \Gamma distance away (in odds ratio scale) from the observed, parametric propensity score P(A=1 | X). Conduct bootstrap and for each bootstrap sample, obtain estimates of the bounds of the ATE via IPW estimator and linear programming Obtain percentile confidence intervals based on the estimated bounds above Theory shows that this bootstrapped CI is asymptotically valid.
Du et al. (2024) Causal Inference for Genomic Data with Multiple Heterogeneous Outcomes. Problem: estimate ATEs across hundreds of outcomes Solution: estimators based on the efficient influence function (EIF) of the standardized estimands Use Gaussian multiplier bootstrap (Chernozhukov et al. 2013) to control for false discovery rates in multiple testing Applications to Perturb-seq experiments where genes are randomized to treatment or control via CRISPR technology and multiple outcomes (gene expression data) are measured.
Li, Wager (2022) Random graph asymptotics for treatment effect interference. AoS. Problem: Estimate network causal effects under a randomized experiment Solution: propose estimators of network causal effects and study their asymptotic properties with random graphs Interference graph is randomly generated from a graphon, a type of random graph. Requires assumptions about stratified inference Other approaches to asymptotics under general interference: (a) Stein s method, (b) psi-dependence, etc.
Son et al. Spatial causal inference in the presence of preferential sampling... arXiv. Problem: estimate treatment effects when there is spatial confounding and non-i.i.d. sampling Solution: a linear outcome model with an inhomogeneous Poisson point process model for non-i.i.d. sampling Spatial confounding induced by a Gaussian process Bayesian estimator This is a growing area of causal inference with applications to environmental sciences; lots of interesting theoretical, methodological, and applied questions.
Dahabreh et al. (2020) Extending inferences from a randomized trial Stat Med. Problem: Estimate the treatment effect in a new population based on the estimates from a randomized trial in the study population. Solution: a framework to transport ATEs between populations Let S = {0,1} denote the sample indicator where S=1 denotes the new population and S=0 denotes the study population Let X denote the covariates of study units. Transportability/exchangeability: P(Y(1), Y(0) | S, X) = P(Y(1), Y(0) | X) Estimation methods: outcome regression, inverse probability weighting, and doubly robust estimators
Li, Luedtke (2023) Efficient estimation under data fusion. Biometrika. Problem: Estimate smooth, low-dimensional parameters (e.g., means, ATEs, etc.) using data from multiple sources Solution: a general (in some sense, unified?) derivation of the the semiparametric efficiency lower bound of said parameters when there are datasets from multiple sources Characterizing situations when multiple datasets are useful for improving efficiency Construction of nonparametric estimators that achieve the efficiency lower bound Many examples to illustrate their general approach Technically precise derivations (see Alex Luedtke s other works); you do need to know a bit of ideas from empirical process theory and TMLE.
Stoetzer et al. (2024) Causal inference with latent outcomes. American Journal of Political Science. Problem: identify and estimate the treatment effect on a latent outcome (LTEs) Example 1: the effect of different types of newspaper articles on legitimacy (a latent concept) Example 2: the effect of door-to-door canvassing on prejudicial attitudes against immigrants (a latent concept) Solution: lays out the identification strategy for LTEs Proposes simple estimators for LTEs (plug-in, regression estimators) Discussion about LTE estimation and item response theory models While not the first paper in this area, I think this field, in general, has broader and important applications beyond political science (e.g., educational psychology, statistical genomics).
Kallus, Mao (2024) On the role of surrogates in the efficient estimation of. arXiv. Problem: estimate treatment effects on an outcome in the presence of a surrogate outcome (i.e., intermediate outcome) Example 1: estimate the effect of education policy about pre-K on long-term income potential using short-term income (e.g., surrogates) Example 2: estimate the effect of new cancer therapy on long-term remission based on intermediate immune-related biomarkers (e.g., surrogates) Solution: study how surrogates affect the efficiency of estimating treatment effects Derivation of semiparametrically efficient estimators under different scenarios involving the surrogates and the outcome. Some interesting application of semiparametric efficiency theory to approximate certain, finite-sample efficiency properties. Some interesting estimation techniques for nuisance parameters.
General Advice for Reading Causal Papers 1. What is the causal estimand or the model that defines the estimand? Is the estimand theoretically interesting (e.g., non-smooth, infinite-dimensional parameters or finite-dimensional parameters that are not easy to identify/estimate)? Is it practically interesting (e.g., formalizes a practical question in a way that s statistically interesting or impactful for practice) 2. What is the model for the data? How is the data sampled? Parametric/semiparametric/nonparametric model What kind of restrictions are on the model? i.i.d. sampling, randomization inference, dependence, multiple datasets, etc. 3. What assumptions are needed (or not needed) to identify (or bound) the causal estimand? Are these assumptions clearly stated, explained well, and reasonable? Are some assumptions satisfied the design of the study (e.g., RCTs)? (For sensitivity analysis and bounds): is the sensitivity model reasonable and/or well- explained? Are the assumptions used to tighten bounds too unrealistic?
General Advice for Reading Causal Papers 4. (For method papers): What kind of statistical properties are shown about the new estimator/test/method? For new estimators, did they prove (a) consistency and whenever relevant, (b) asymptotic Normality? Which theorems/assumptions did they use from math stats and are they reasonable? For new tests, did they prove (a) size control? Also, did they prove or numerically demonstrate statistical power? Did the paper compare the new method to regression or a simpler/na ve method in real data? 5. (For theory papers): Is the theoretical object/phenomena interesting? Are the theoretical results useful to understand the statistical fundamentals of the problem (e.g., efficiency lower bound, minimax rates, difficulty of the estimation problem) (For asymptotics): is the asymptotic sequence a good approximation to finite sample behavior? Which assumptions are technical / non-essential (e.g., moment assumptions) and which assumptions are essential ? Which proof techniques are useful for your own work? 6. (For papers that have data): Is the data interesting or useful for the paper? Is the applied problem from the data framed in a way that s statistically interesting and useful? Does the method address a real problem from the data? 7. Based on the answers from questions 1-7, how does the paper advance the field or stand out from other works?
Advice for Future Students of Causal Inference This is my own (soapbox, biased, etc.) opinion based on working in this field for 10-ish years. I think the field has solved the standard causal inference problem in standard data settings. A single, cross-sectional dataset with i.i.d.sampling. Strong ignorability with non-vanishing overlap and SUTVA. All observables measured (no missingness) Binary or discrete (non-ordered) treatment Binary or continuous outcome (except causal odds ratio) Pre-treatment covariates (low-dimensional) Optimal (i.e., efficient), semi/nonparametric estimation of the ATE, ATT, CATE (to a large extent), and optimal policy Standard sensitivity analysis of ATE and ATT when strong ignorability is violated. The problem is mostly solved under finite-sample, randomization inference. For estimation and inference, the modern trend in i.i.d. settings is to use (a) efficient influence functions (EIFs) and (b) machine learning methods via cross-fitting. While efficient and nonparametric, it s not the most accessible approach to practitioners. Method/theory and some applied papers in statistics journals encourage some discussions on this. Thankfully, it s now reasonably easy to learn this topic as a 2ndyear Ph.D. student (e.g., Edward Kennedy s summary papers); background in empirical processes is useful.
Advice for Future Students of Causal Inference After my recent foray into statistical genomics, I m more convinced that a major (practical) problem in causal inference is Understanding the real-world performance of new methods Whether new methods dramatically improve how PIs make scientific conclusions compared to regression Lack of rigorous, real-world validations I also think the interesting causal problems are Either Studying non-standard causal estimands from standard samples Studying standard causal estimandsfrom non-standard samples Studying non-standard causal estimands from non-standard samples They should all be theoretically, methodologically, and/or practically interesting. Developing new methods to use multiple datasets or auxiliary variables (e.g., identification, efficient estimation, bias from unmeasured confounding, etc.) Fusing ideas/techniques from causal inference (e.g., covariate balance, sensitivity analysis, missing data, EIFs) and other fields (e.g., IRT models, randomized algorithms)