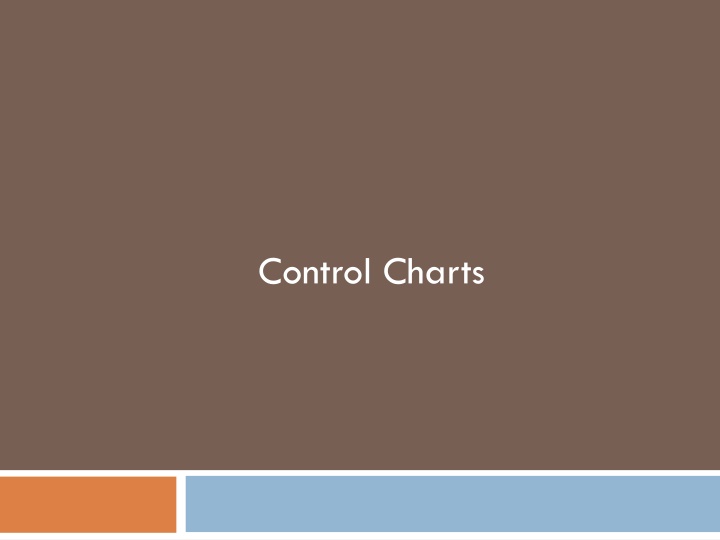
Understanding Control Charts for Quality Control
Learn about control charts for attributes, population distribution, setting control limits, and practical examples like the p-chart. Control charts help monitor processes to ensure quality and detect variations in categorical variables.
Download Presentation

Please find below an Image/Link to download the presentation.
The content on the website is provided AS IS for your information and personal use only. It may not be sold, licensed, or shared on other websites without obtaining consent from the author. If you encounter any issues during the download, it is possible that the publisher has removed the file from their server.
You are allowed to download the files provided on this website for personal or commercial use, subject to the condition that they are used lawfully. All files are the property of their respective owners.
The content on the website is provided AS IS for your information and personal use only. It may not be sold, licensed, or shared on other websites without obtaining consent from the author.
E N D
Presentation Transcript
Control Charts for Attributes For variables that are categorical Good/bad, yes/no, acceptable/unacceptable Measurement is typically counting defectives Charts may measure Percent defective (p-chart) Number of defects (c-chart)
Control Limits for p-Charts Population will be a binomial distribution, but applying the Central Limit Theorem allows us to assume a normal distribution for the sample statistics UCLp= p + z p^ p (1 - p) n p= ^ LCLp= p z p^ where p = mean fraction defective in the sample z = number of standard deviations p= standard deviation of the sampling distribution n = sample size ^
p-Chart - Example Clerks at Mosier Data systems key in thousands of insurance records each day for a variety of client firms. The CEO wants to set control limits to include 99.73% of the random variation in the data entry process when it is in control. Sample of the work of 20 clerks are gathered and shown in the following table (Next slide). Mosier carefully examined 100 records entered by each clerk and counts the number of errors. She also computes the fraction defective in each sample. Calculate the control limits .
p-Chart Example Sample Number 1 2 3 4 5 6 7 8 9 10 Number of Errors 6 5 0 1 4 2 5 3 3 2 Sample Number 11 12 13 14 15 16 17 18 19 20 Number of Errors 6 1 8 7 5 4 11 3 0 4
p-Chart Example Sample Number 1 2 3 4 5 6 7 8 9 10 Number of Errors 6 5 0 1 4 2 5 3 3 2 Fraction Defective .06 .05 .00 .01 .04 .02 .05 .03 .03 .02 Sample Number 11 12 13 14 15 16 17 18 19 20 Number of Errors Fraction Defective .06 .01 .08 .07 .05 .04 .11 .03 .00 .04 6 1 8 7 5 4 11 3 0 4 Total = 80
p-Chart Example Sample Number 1 2 3 4 5 6 7 8 9 10 Number of Errors 6 5 0 1 4 2 5 3 3 2 Fraction Defective .06 .05 .00 .01 .04 .02 .05 .03 .03 .02 Sample Number 11 12 13 14 15 16 17 18 19 20 Number of Errors Fraction Defective .06 .01 .08 .07 .05 .04 .11 .03 .00 .04 6 1 8 7 5 4 11 3 0 4 Total = 80 (.04)(1 - .04) 100 80 p= = .02 p = = .04 ^ (100)(20)
p-Chart - Example UCLp= p + z p= .04 + 3(.02) = .10 ^ LCLp= p z p= .04 - 3(.02) = 0 ^ .11 .10 .09 .08 .07 .06 .05 .04 .03 .02 .01 .00 UCLp= 0.10 Fraction defective p = 0.04 LCLp= 0.00 | | | | | | | | | | 20 18 16 14 12 10 8 6 4 2 Sample number
p-Chart - Example UCLp= p + z p= .04 + 3(.02) = .10 ^ LCLp= p z p= .04 - 3(.02) = 0 ^ Possible assignable causes present .11 .10 .09 .08 .07 .06 .05 .04 .03 .02 .01 .00 UCLp= 0.10 Fraction defective p = 0.04 LCLp= 0.00 | | | | | | | | | | 20 18 16 14 12 10 8 6 4 2 Sample number
Control Limits for c-Charts Population will be a Poisson distribution, but applying the Central Limit Theorem allows us to assume a normal distribution for the sample statistics UCLc= c + z c LCLc= c z c where c = mean number of defects per unit
c-Chart for Cab Company Red Top Cab company receives several complaints per day about the behavior of its drivers. Over a 9-day period (where days are the units of measure), the owner received the following number of calls from irate passengers: 3, 0, 8, 9, 6, 7, 4, 9, 8 for a total of 54 complaints. The owner wants to compute 99.73% control limits.
c-Chart for Cab Company c = 54 complaints/9 days = 6 complaints/day UCLc = c + 3 c = 6 + 3 6 = 13.35 UCLc= 13.35 Number defective 14 12 10 8 6 4 2 0 c = 6 LCLc = c - 3 c = 6 - 3 6 = 0 LCLc= 0 | 1 | 2 | 3 | 4 | 5 | 6 | 7 | 8 | 9 Day
Which Control Chart to Use Variables Data Using an x-Chart and R-Chart 1. Observations are variables 2. Collect 20 - 25 samples of n = 4, or n = 5, or more, each from a stable process and compute the mean for the x-chart and range for the R-chart 3. Track samples of n observations each.
Which Control Chart to Use Attribute Data Using the p-Chart 1. Observations are attributes that can be categorized as good or bad (or pass fail, or functional broken), that is, in two states. 2. We deal with fraction, proportion, or percent defectives. 3. There are several samples, with many observations in each. For example, 20 samples of n = 100 observations in each.
Which Control Chart to Use Attribute Data Using a c-Chart 1. Observations are attributes whose defects per unit of output can be counted. 2. We deal with the number counted, which is a small part of the possible occurrences. 3. Defects may be: number of blemishes on a desk; complaints in a day; crimes in a year; broken seats in a stadium; typos in a chapter of this text; or flaws in a bolt of cloth.