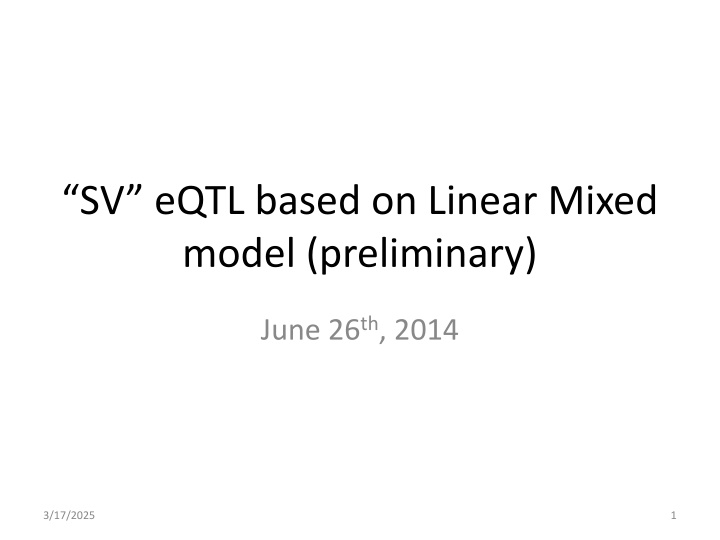
Unveiling Population Stratification in eQTL Studies
Explore the impact of population stratification in eQTL studies, including challenges, correction options, and the use of Linear Mixed Models to control for population-specific variations. Learn about the significance of genotype effects and the computational burden faced in analyzing diverse population structures.
Download Presentation

Please find below an Image/Link to download the presentation.
The content on the website is provided AS IS for your information and personal use only. It may not be sold, licensed, or shared on other websites without obtaining consent from the author. If you encounter any issues during the download, it is possible that the publisher has removed the file from their server.
You are allowed to download the files provided on this website for personal or commercial use, subject to the condition that they are used lawfully. All files are the property of their respective owners.
The content on the website is provided AS IS for your information and personal use only. It may not be sold, licensed, or shared on other websites without obtaining consent from the author.
E N D
Presentation Transcript
SV eQTL based on Linear Mixed model (preliminary) June 26th, 2014 3/17/2025 1
The main blame in GWAS : population stratification A simple example for spurious association Population B Population A Ref Allele Alt Allele High expression Low expression Then all the variations that is population specific would be under significant association! (up to 10000+) 3/17/2025 2
Stratification Correction Options Matched case and control association Not possible in eQTL studies Remove samples? hidden relatedness, sub-population structures, reduced statistical power PCA based association studies (after 2007) Not enough for complicated population structures Linear Mixed Models (pioneer paper on 2007, simplified but still under extensive computation) 3/17/2025 3
A Simple Linear Mixed Model y Log(gene expression) Parameters for eQTL studies x Genotype info z Indicator function for population y = bx +b1z1+b2z2+e Fixed coefficient to be estimated Random variables Assumptions: o Genotype effect is fixed across all populations o b1 and b2 are multivariable normally distributed, basically we are assuming different intercept for different population groups to control population difference o is the i.i.d. random variables for the error items o To test whether =0 or not e 3/17/2025 4
Challenges at first glance How many populations could we assume? Dimension of z equal to # of samples (Different from the Lobster eQTLs where just 2 groups are used) Computational Burden Large Matrix inversion during Newton-Raphson Method SVD decomposition for the matrix inversion steps with predefined relatedness matrix estimation Relatedness Matrix Computation use the common SNPs to calculate the matrix (tentative, suggested) 3/17/2025 5
The non SNV variants overview - length 0.5 0.4 count percent 0.3 0.2 0.1 0.0 1 2 3 4 5 6 7 8 9 10 20 70 120 170 500 1500 3500 5500 7500 9500 variant length region 2000 1500 count 1000 500 0 70 120 170 500 1500 2500 3500 4500 5500 6500 7500 8500 9500 variant length region 3/17/2025 6
The non SNV variants overview - missingness Histogram of impute[len <= 10] 4e+05 impute percent 2e+05 0e+00 0.0 0.2 0.4 0.6 0.8 1.0 impute[len <= 10] High Missingness != rare, since missingness = uncertein due to coverage or other issue Around half of the variants have imputation rate > 0.15, which are not believable for further association test (although can be used anyway) Somehow: the length>500 variants all with imputation rate = 0.128 (need to be checked up later) Longer variants are supposed to have larger number of missingness 3/17/2025 7
The non SNV variants overview rare alleles Larger SVs are usually rare (even after ignoring of missingness), how to do the association study? (collapsed tests?) 3/17/2025 8
Current Scheme Relatedness matrix Search all SNVs Require imputation rate < 0.7 (probably too high , would decrease) Require MAF >= 0.05, common SNP definition Centralized covariant matrix used SVs used Search all non-SNV variants Just use genotype for association Imputation rate filter: <0.70 MAF filter: >=0.05 Cis-element association: for each gene search the region within up and downstream 100kbp (and inside gene body and introns) BH correction for P values 3/17/2025 9
3/17/2025 10
Next Step Reliable SV calls (other than phase 1 data?) Missingness: no way to avoid, just remove some or directly use anything from imputation Rare variants: pooled regression within a whole region Repressor used? SV length as x? not a good option for longer SVs Functional impact score? Max/average/impact score, such as single base resolution Funseq scores? Model comparison: before and after the stratification correction 3/17/2025 11