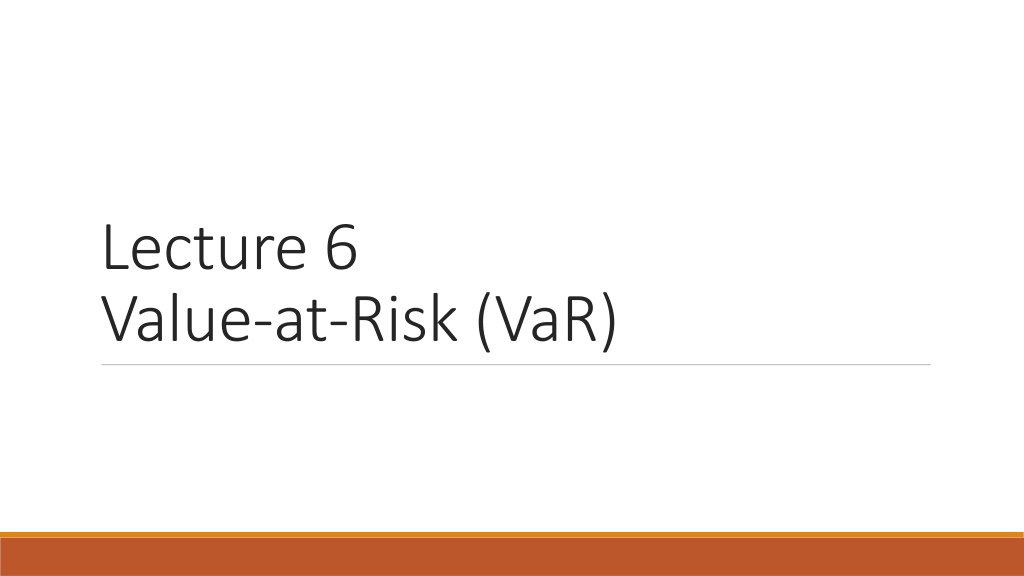
Understanding Value-at-Risk (VaR) and Market Risk
Explore the concept of Value-at-Risk (VaR) as a measure of market risk, focusing on the potential losses in trading and investment positions due to adverse market movements. Discover how VaR addresses risk aversion and sets confidence levels to determine acceptable loss thresholds.
Uploaded on | 0 Views
Download Presentation

Please find below an Image/Link to download the presentation.
The content on the website is provided AS IS for your information and personal use only. It may not be sold, licensed, or shared on other websites without obtaining consent from the author. If you encounter any issues during the download, it is possible that the publisher has removed the file from their server.
You are allowed to download the files provided on this website for personal or commercial use, subject to the condition that they are used lawfully. All files are the property of their respective owners.
The content on the website is provided AS IS for your information and personal use only. It may not be sold, licensed, or shared on other websites without obtaining consent from the author.
E N D
Presentation Transcript
Lecture 6 Value-at-Risk (VaR)
Todays content Market risk Value-at-Risk (VaR) Delta-normal approach Historical simulation Expected shortfall Stress testing Readings: oBessis (2015) chapters 11, 13, 14 oHull (2015) chapters 12 and 13 oRose and Hudgins (2013) chapter 15
Market Risk Market risk is the potential for changes in the market value of trading and investment positions Primary exposures include interest rates, currencies, equities (and other asset prices), and commodities High sensitivity to the business environments being operated in These depend on: Global GDP growth Efficient capital markets Low inflation High business and investor confidence Geopolitical conditions Business earnings
Market Risk Market risk can be defined as the risk of losses in on- and off-balance sheet positions arising from adverse movements in market prices (European Banking Authority) Market risk includes: Price risk, interest rate risk, FX risk, etc. Most common measure of market risk is volatility What is the problem with volatility?
Value-at-Risk as measure of market risk Risk = possibility of a loss Volatility does not only capture losses but also gains (symmetric) Value-at-Risk (VaR) = risk measure focusing only on losses Developed by JP Morgan in reaction to huge losses in financial scandals Within the context of market risk, VaR can be defined as the maximal loss of a financial position during a given time period for a given probability Measure of loss associated with a rare (or extraordinary) event under normal market conditions VaR can be interpreted as the reasonable amount that can be lost within a given time horizon What is reasonable will depend on the risk aversion of the user The more risk averse, the smaller the probability that the loss will be exceeded
A loss needs to be defined relative to a benchmark Common benchmarks are zero return (absolute loss) and expected return (relative loss) Here we focus on the relative loss The choice of confidence level will depend on the risk aversion The more risk averse an investor is, the higher the confidence level chosen For regulatory purposes, use 99% confidence level (Basel Committee s quantitative criteria) Example: A bank estimates its portfolio s daily average VaR = $100 billion over a 10-day interval with a 99% level of confidence If this VaR estimate is correct, the loss in portfolio value will not exceed $100 billion with a probability of 99% over the next 10-day period (or loss will exceed $100 billion with probability less than 1%)
VaR: a simple example Consider a $100 million portfolio of medium-term bonds Suppose a confidence level of 95% (95% of possible market events is defined as normal ) What is the maximum loss under normal market conditions over any particular month? Let s look at historical monthly returns on medium-term bonds: Lowest: -6.5% Highest: 12% Graph the frequency distribution of returns
At the 95% confidence level, lowest monthly return = -1.7% there are 26 months out of 516 for which monthly returns < -1.7% there is a 5% chance that next monthly return < -1.7% VaR = $100 million * 1.7% = $1.7 million 95% of the time, the portfolio s loss will be no more than $1.7 million 5% of the time, the portfolio s loss will be greater than $1.7 million If you increase your confidence level (more risk averse), you increase the VaR
VaR computation for a single asset VaR is the difference between the expected value of the asset and the lowest value of the asset at time t with probability : VaR = Vm Vq= V0(1 + Rm) V0(1 + Rq) = V0(Rm Rq) V0 current market value of asset Vm expected value of asset at time t Vq lowest value that the asset can record, for a given confidence level Rm average asset return for horizon t Rq lowest asset return corresponding to confidence level
Probability distribution and percentile P[X x( )]= Percentile x( )
The lowest return Rq is determined from the distribution of returns: ??? ? ?? P(? < Rq) = If returns are normally distributed: Rq = Rm VaR = V0(Rm Rq) = V0(Rm Rm + ) VaR = V0 loss probability asset volatility
Normal distribution and VaR For 99% confidence level = 2.33 For 95% confidence level = 1.65
Example Long position = $10 million Confidence level = 95% Probability that the loss VaR = 5% Standard deviation of daily returns = 0.53% Daily VaR = V0 = $10 million * 1.65 * 0.53% = $87,450 10-day VaR = Daily VaR * 10 = $276,541.18
VaR computation for multiple assets Most commonly used approaches to measure portfolio VaR: Variance-covariance method (delta-normal) Historical simulation method Monte Carlo simulation method
Variance-covariance (delta-normal) method Portfolio return is normally distributed as it is the linear combination of risky assets, therefore need: Historical volatility of each asset Correlation between each pair of assets Position on each asset (risk factor) Need to calculate: 1. Individual VaR of each asset 2. Portfolio VaR, using the portfolio risk formula
Portfolio VaR in the case of 2 assets: 2+ ???? 2+ 2??????????? ????= ???? ???? Value-at-risk of asset A ???? Value-at-risk of asset B ??? Correlation between A and B This is equivalent to: 2?? 2+ ?? 2?? 2+ 2??????? ????= ?? ? ??= ?? ? ??
Example: Calculate the 10-day ????, ???? and ???? for a 99% confidence level assuming a correlation of 0.5 between A and B. What if ? = 0? What if ? = -1?
????= $5,000 x 2.33 x 1% x 10 = $368.41 ????= $10,000 x 2.33 x 2% x 10 = $1,473.62 ????= 368.412+ 1,473.622+ 2 368.41 1,473.62 0.5 = $1,688.25 If ? = 0.5 ????= 368.412+ 1,473.622 = $1,518.97 If ? = 0 ????= 368.412+ 1,473.622+ 2 368.41 1,473.62 ( 1) = $1,105.21 If ? = -1
Application with 5 assets EXCEL WORKSHOP #1 EXCEL WORKSHOP #1
Duration and VaR Value-at-Risk is directly linked to the concept of duration in situations where a portfolio is exposed to interest rate risk only Duration measures the sensitivity of the bond price to changes in yield: So as duration increases, the interest rate risk is higher
Example: In the simple example above ($100 million portfolio of medium-term bonds), at 95% confidence level, portfolio VaR = $1.7 million over one month Typical duration for a 5-year note is 4.5 years Assume now that current yield = 5% From historical data: worst increase in yield over a month = 0.40% (at 95%) Worst loss (or VaR) is given by: VaR = Duration x portfolio value x i/(1+i) where i = worst yield increase VaR = 4.5 x $100m x (0.004/1.05) = $1.7 million
Historical simulation Based on assumption that history will repeat itself Going back in time (e.g. over the last 5 years) and applying current weights to a time-series of historical asset returns Calculated returns do not represent an actual portfolio Reconstruct the history of a hypothetical portfolio using the current position Need: A time-series of actual movements for each risk factor Positions on risk factors
Example 1: Portfolio of two assets with following current market values: V0 = $70 and W0 = $30 P0 = $100 Need to "re-value" these assets based on their historical price movements. Observe the market prices of these two assets for the past 100 trading days:
The 100 sets of daily change in the portfolio value, Pn can be ranked from the day with the worst performance to the day with the best performance: To find the Value-at-Risk with 95% confidence level: 95th percentile (one tailed) 5th worst performance = -$9
Example 2: Instead of taking P0 as base price, it makes more sense to take latest price Pn Obtain data for the last 500 days Today = 500; Tomorrow = 501 Generate 500 alternative scenarios that could happen between today and tomorrow Compute values for tomorrow if their % change between today and tomorrow are the same as they were between Day i-1 and Day i for 1<i<500
Day Var 1 Var 2 etc Var N 0 0.1132 65.37 20.33 1 0.1159 64.91 20.78 2 21.44 0.1162 65.02 499 25.75 0.1323 61.99 500 0.1343 62.10 25.85 Today
= = today value market variable Day scenario assumes n v ith i i value of market variables tomorrow v i v n v 1 i : 1st Market Variable 1st scenario: 500, 25.85; n v = = EX n = = = 20.33; 20.78 v v 500 0 1 20.78 20.33 25.85 =26.42
Scenario Number Market variable 1 Market variable 2 Market variable N Portfolio value ($m) Ch. In value ($m) 0.21 1 26.42 0.1375 61.66 23.71 2 26.67 0.1346 62.21 23.12 499 25.88 0.1354 ... 61.87 23.63 500 25.95 0.1363 62.21 22.87 Under Scenario Number 1: Value of portfolio tomorrow (t=501) = $23.71m Value of portfolio today (t=500) = $23.50m Change in value of portfolio between today and tomorrow = $23.71-$23.50 = +$210000
Compute $ change in the value of the portfolio between today and tomorrow for each of the 500 scenarios Gives: Probability distribution for daily changes in the value of the portfolio Rank changes in value 5th worst loss (out of 500) 1-day 99% VaR Can follow same procedure to update daily VaR (i.e. always last 500 days of data)
Application with 5 assets EXCEL WORKSHOP #2 EXCEL WORKSHOP #2
Monte Carlo simulation Two steps: 1. Specify a stochastic process for financial variables as well as process parameters Choice of distributions and parameters such as risk and correlations can be derived from historical data 2. Simulate fictitious price paths for all variables of interest At each horizon considered, portfolio is marked-to-market using full valuation Each of these pseudo realizations is then used to compile a distribution of returns, from which a VaR figure can be measured Need for each risk factor: specification of a stochastic process (i.e., distribution and parameters) valuation models for all assets in the portfolio positions on various securities
Comparison of Approaches Model building (delta-normal) approach: Advantages: results produced quickly can be used with volatility updating schemes (e.g: GARCH) Disadvantage: Assumes market variables have a multivariate normal distribution. (e.g: daily changes not normal usually) Historical simulation approach: Advantages: historical data determine the joint prob. distribution of the market variables Disadvantage: Computationally slow Monte Carlo simulation: Slow company complete portfolio revalued many times different instruments
VaR in practice VaR has been traditionally used by regulators for setting capital requirements for market risk, but also credit risk and operational risk JP Morgan RiskMetrics Allows users to compute a portfolio VaR based on a 95% confidence level over a daily or monthly horizon Deutsche Bank Risk Office (formerly RAROC 2020) Provides VaR estimates at the 99% confidence level over an annual horizon, using Monte Carlo method RAROC = Risk-adjusted return on capital
Current modelling practices In a survey of 13 leading banks in Europe and North America, McKinsey (2012) show that: 50% of banks run at least 2 different iterations of VaR, 1 for regulatory reporting and 1 for internal management All calculate VaR on a 1-day holding period 62% of them extrapolate the 10-day VaR (required by regulators) from the 1-day VaR Banks that calculate VaR for internal use do so with shorter holding periods (< 10 days) and sometimes lower confidence interval (< 99%) Most banks use historical analysis to determine whether relative or absolute returns best fit a given asset class
Limitations and challenges of VaR VaR estimates and internal modeling are not perfect Inaccurate VaR estimates can expose a bank to excessive risk so that its capital position may turn out not to be large enough to cover actual losses the bank faces The portfolios of the largest banks are so complex with thousands of risk factors it may be impossible to consistently forecast VaR accurately VaR does not measure event risk (e.g., market crash) portfolio stress tests are recommended to supplement VaR VaR does not readily capture liquidity differences among instruments Promote backtesting Even if an individual bank is a good forecaster, there may still be trouble due to systemic risk
Expected Shortfall Regulators are switching from VaR to expected shortfall (ES) for calculating market risk Also called conditional VaR one step further Expected loss during time T conditional on the loss being greater than the Xth percentile of the loss distribution Example: X = 99; T = 10 days; VaR = 50 million ES = average amount lost over a 10-day period assuming that the loss is greater than 50 million
VaR versus ES: case of 2 portfolios Is VaR a coherent measure of risk? Risk measure for 2 portfolios after they have been merged should be no greater than the sum of their risk measures before they were merged Example: Two independent portfolios (1-year horizon, confidence level = 97.5% tail of 2.5%) For each: Loss of 10m with p=0.02 and loss of 1m with p=0.98 VaR = 1m for each portfolio If combined: Loss of 20m with p=0.0004 (=0.02 0.02), loss of 11m with p=0.0392 (=2 0.02 0.98) and loss of 2m with p=0.9604 (=0.98 0.98) VaR = 11m for combined portfolio VaR (P1+P2) > VaR (P1) + VaR (P2)
Expected shortfall: For each portfolio: in the tail of the loss distribution, 2.5% = 2% chance of losing 10m and 0.5% chance of losing 1m Conditional probabilities: Loss of 10m with p=0.8 and loss of 1m with p=0.2 ES = 10m 0.8 + 1m 0.2 = 8.2m for each portfolio If combined: in the tail of the loss distribution, 2.5% = 0.04% chance of losing 20m and 2.46% chance of losing 11m Conditional probabilities: Loss of 20m with p=0.016 and loss of 11m with p=0.984 ES = 20m 0.016 + 11m 0.984 = 11.144m for combined portfolio ES (P1+P2) < ES (P1) + ES (P2)
BIS standards for ES (2016) ES must be computed on a daily basisfor the bank-wide internal model for regulatory capital purposes In calculating the expected shortfall, a 97.5th percentileconfidence level is to be used ES measure must be calibrated to a period ofstress Must replicate an expected shortfall charge that would be generated on the bank s current portfolio if the relevant risk factors were experiencing a period of stress No particular type of expected shortfall model is prescribed So long as model used captures all the risks run by the bank, and conforms to each of the capital requirements, banks can use either historical simulation, Monte Carlo simulation, or other appropriate analytical methods
Other alternatives VAR-iations: Marginal VaR Incremental VaR Extreme VaR Stressed VaR Other tools for managing market risk: Sensitivity/scenario analyses
Stress testing and back testing Back Testing: Testing how the VaR estimates would have performed in the past Stress Testing: Estimating how the portfolio would have performed under some of the most Extreme market moves seen in last 8 to 25 years EX: Extreme movement in US equity prices: set % change in all market variables equal to October 19th 1987 S&P500 moved 22.3 s.d s EX: Yield curve shifts 100, 200 basis points
Regulatory stress testing In Europe: http://www.eba.europa.eu/risk-analysis-and-data/eu-wide-stress-testing The European Banking Authority s stress test scenario includes key macroeconomic components, such as: Negative GDP growth Abrupt reversal of compressed global risk premia Shadow banking sector that becomes increasingly stressed Shock in residential and commercial real estate prices Foreign exchange risk in central and eastern Europe Misconduct risk In the USA: Comprehensive Capital Analysis and Review (CCAR) by the Fed
BIS standards for stress testing (2016) Banks that use internal model approach for meeting market risk capital requirements must have in place a rigorous and comprehensive stress testing program Stress testing to identify events or influences that could greatly impact banks Key component of a bank s assessment of its capital position Stress scenarios need to cover a range of factors that can create extraordinary losses or gains in trading portfolios, or make the control of risk in those portfolios very difficult Factors include low-probability events in all major types of risk (market, credit, and operational risks) Stress tests should be both of a quantitative and qualitative nature, incorporating both market risk and liquidity aspects of market disturbances The results of stress testing should be routinely communicated to senior management and, periodically, to the bank s board of directors