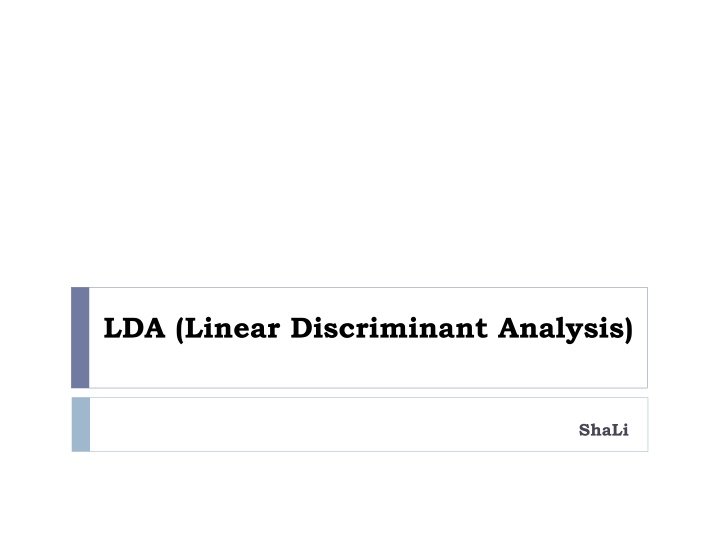
Understanding LDA for Data Analysis
Dive into Linear Discriminant Analysis (LDA) to comprehend its role in data analysis. Learn about its limitations, differences from PCA, and how it supports classification tasks. Explore the idea behind LDA and its application in handling non-Gaussian distributions for improved data classification.
Download Presentation

Please find below an Image/Link to download the presentation.
The content on the website is provided AS IS for your information and personal use only. It may not be sold, licensed, or shared on other websites without obtaining consent from the author. If you encounter any issues during the download, it is possible that the publisher has removed the file from their server.
You are allowed to download the files provided on this website for personal or commercial use, subject to the condition that they are used lawfully. All files are the property of their respective owners.
The content on the website is provided AS IS for your information and personal use only. It may not be sold, licensed, or shared on other websites without obtaining consent from the author.
E N D
Presentation Transcript
Limitation of PCA The direction of maximum variance is not always good for classification
Limitation of PCA The direction of maximum variance is not always good for classification
Limitation of PCA The direction of maximum variance is not always good for classification
Limitation of PCA The direction of maximum variance is not always good for classification
Limitation of PCA There are better direction that support classification tasks. LDA tries to find the best direction to separate classes
Idea of LDA 1x 2 1 w m 2 2 2 S m 1 2 1 S 2x
Idea of LDA Find the w that m maximizes m 1 2 minimizes S + 2 1 2 2 S 1x 2 1 w m 2 2 2 S 1 m 2 1 S 2 x
Limitations of LDA If the distributions are significantly non Gaussian, the LDA projections may not preserve complex structure in the data needed for classification LDA will also fail if discriminatory information is not in the mean but in the variance of the data
LDA for two class and k=1 1x Compute the means of classes: 2 1 N 1 1 x i x i = = , x x w 1 2 i i N R R 1 2 m 1 2 2 2 2S m 1 2 1S Projected class means: 1 w N i 2x 1 = = = = T T T T , m x w m w x w 1 1 2 2 i i N x R x R 1 2 1 2 i Difference between projected class means: ( 2 1 2 = w m m T ) 1 10
LDA for two class and k=1 = = 2 1 2 1 2 2 2 2 , Scatter of projected data in 1 dim space s s = = = 2 1 2 T T T T ( ) ( )( ) s w 1 x m w x x w w S w 1 1 1 1 i i i class x x R 1 i i = = = 2 2 2 T T T T ( ) ( )( ) s w 2 x m w x x w w S w 2 2 2 2 i i i class x x R 2 i i 1x 2 1 w m 2 2 2S m 1 2 1S 2x 11
Objective function 1x Find the w that 2 m maximizes m 1 2 1 minimizes w S + 2 1 2 2 S 2m 2 2S 1m LDA does this by 2 1S 2x maximizing : 2 ( ) m m sqr. mean difference = = ( ) 2 1 r w Between class scatter + 2 1 2 2 s s 12
Objective functionNumerator 2 ( ) m m = ( ) 2 1 r w We can rewrite: + 2 1 2 2 s s m = 2 2 T T ( ) m ( ) w w 2 1 2 1 = T T ( )( ) w w 1 2 1 2 S B = T w S w B Between class scatter 13
Objective functionDenominator 2 ( ) m m = ( ) 2 1 r w We can rewrite: + 2 1 2 2 s s 2 1 s = 2 T ( ) w 1 x m 1 i x class i = T T ( )( ) w x 1 x w 1 1 i i class x i 1 S = T w S w 1 s + 2 1 2 2 s = + = T T ( ) w S S w w S w 1 2 W Within class scatter 14
Objective function Putting all together: r 2 T T ( ) m m w S + w w S w = = = 2 1 B B ( ) w + 2 1 2 2 T T ) s s w S S w w S w 1 2 W Where = T ( S )( ) S 2 + 1 2 1 B = S S 1 2 w Maximize r(w) by setting the first derivative of w to zero: w w = 1 ( ) S 2 1 15
Extension to K>1 w = 1 ( ) w S For K=1 2 1 w = 1 Scatter matrix S S For K>1 B = eigenvecto k top r of Scatter matrix w Transform data onto the new subspace: Y = X W n k n d d k
Prediction as a classifier Classification rule: x in Class2 if y(x)>0, else x in Class1, where 1 ) ( 1 m m x w x y + = T ( ) 2 2 1x 2 1 w m 2 m 1 1 m + ( ) m 1 2 2 17 2 x
Comparison of PCA and LDA PCA: Perform dimensionality reduction while preserving as much of the Variance in the high dimensional space as possible. LDA: Perform dimensionality reduction while preserving as much of the class discriminatory information as possible. PCA is the standard choice for unsupervised problems(no labels) LDA exploits class labels to find a subspace so that separates the classes as good as possible
PCA and LDA example Data: Springleaf customer information 2 classes Original dimension: d=1934 Reduced dimension: k=1 Seriously overlap m1 and m2 are close Var1 and Var2 are large
PCA and LDA example Data: Iris 3 classes Original dimension: d=4 Reduced dimension: k=2 PCA LDA
PCA and LDA example Data: coffee bean recognition 5 classes Original dimension: d=60 Reduced dimension: k=3