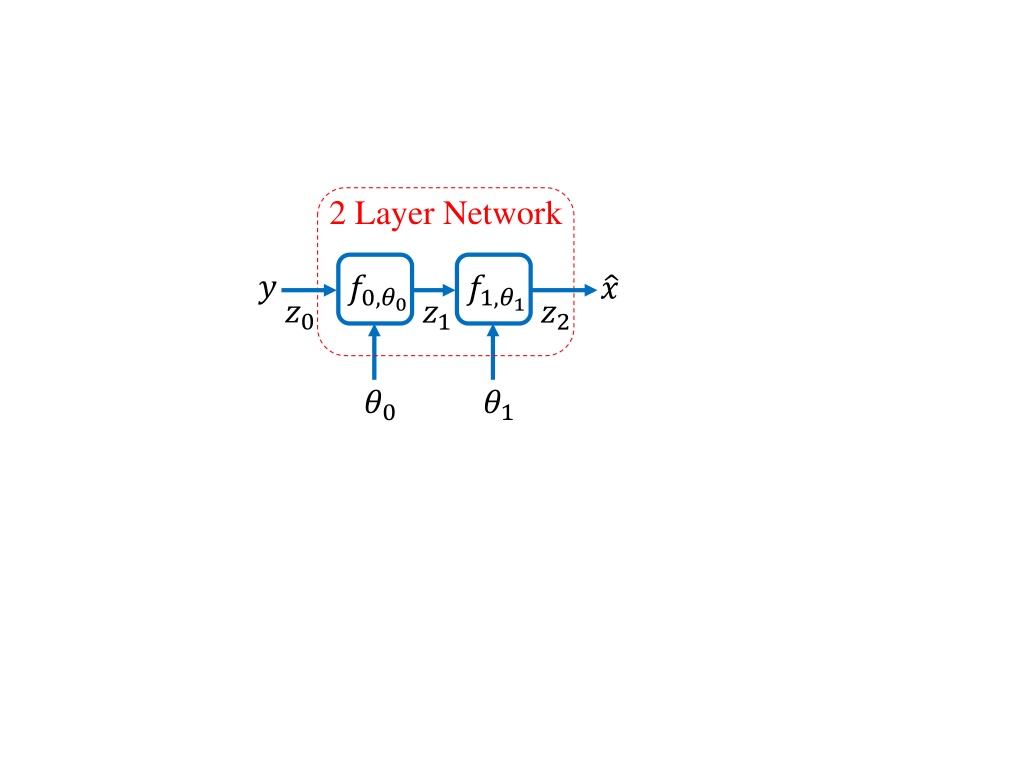
Neural Network Optimization and Loss Functions
Explore the concepts of neural network optimization, forward and back propagation, loss functions, overfitting, and solutions to parameter overfitting. Understand the importance of model order, training data, and testing loss for accurate machine learning system performance.
Download Presentation

Please find below an Image/Link to download the presentation.
The content on the website is provided AS IS for your information and personal use only. It may not be sold, licensed, or shared on other websites without obtaining consent from the author. If you encounter any issues during the download, it is possible that the publisher has removed the file from their server.
You are allowed to download the files provided on this website for personal or commercial use, subject to the condition that they are used lawfully. All files are the property of their respective owners.
The content on the website is provided AS IS for your information and personal use only. It may not be sold, licensed, or shared on other websites without obtaining consent from the author.
E N D
Presentation Transcript
2 Layer Network ? ?0,?0 ?1,?1 ? ?0 ?1 ?2 ?0 ?1
Forward Gradient Propagation ??1 ??2 ??0 ?0 ?1 + + ? ? ?1 ?0 ??0 ??1
Back Propagation ? ? ?? ?1 ?0 ? ? ?0 ?1 ?0,? ?1,? 2 ?? ???? error vector
?? - validation loss Loss ?? - training loss Iteration #
Loss Function Convergence Loss vs. iterations of gradient-based optimization ?? - validation loss generalization loss Loss ?? - training loss Iteration # best stopping point Notice: As training continues, the model is overfit to the data Best to stop training when ?? is at a minimum Model order is too high, but early termination of training can help fix problem
Loss vs. Model Order vs. # Training Pairs Loss vs. model order ?? - validation loss Loss ?? - training loss ? = Model Order best model order/capacity Loss vs. # of training pairs More training data is always better, but slower. Loss ? = # Training Pairs
What are ?? and ?? telling you? Model order/model capacity may be too low Loss ?? - validation loss Small ?? - training loss Iteration # Model order/model capacity may be too high Loss ?? - validation loss Large ?? - training loss Iteration #
Never Test on Training Data! Never report training loss, ??, as your ML system accuracy! But this is terrible Loss This is great ? = # Training Pairs This is like doing a homework problem after you have seen the solution. The network has memorized the answers. Don t even report validation loss, ??, as your ML system accuracy. This is also biased by the fact that your tuned model order parameters. Only report testing loss, ??, as your ML system accuracy. This data is sequestered to ensure it is an unbiased estimate of loss.
Solutions to Parameter Overfitting 1.Early termination 2.Regularization ?2 and ?1 weight regularization Loss function is modified to be ? ? = ? ? + ? ? ? ? larger less overfitting 3.Dropout Method: Next slide
The Dropout Method* Drop nodes with probably ? 0.2 Scale all node outputs by ?: To compute loss for validation and test During inference During Training: Done independently for each batch During Validation, Testing, and Inference *Nitish Srivastava, Geoffrey Hinton, Alex Krizhevsky, Ilya Sutskever, Ruslan Salakhutdinov, Dropout: A Simple Way to Prevent Neural Networks from Overfitting , vol. 15, no. 56, pp. 1929 1958, 2014.