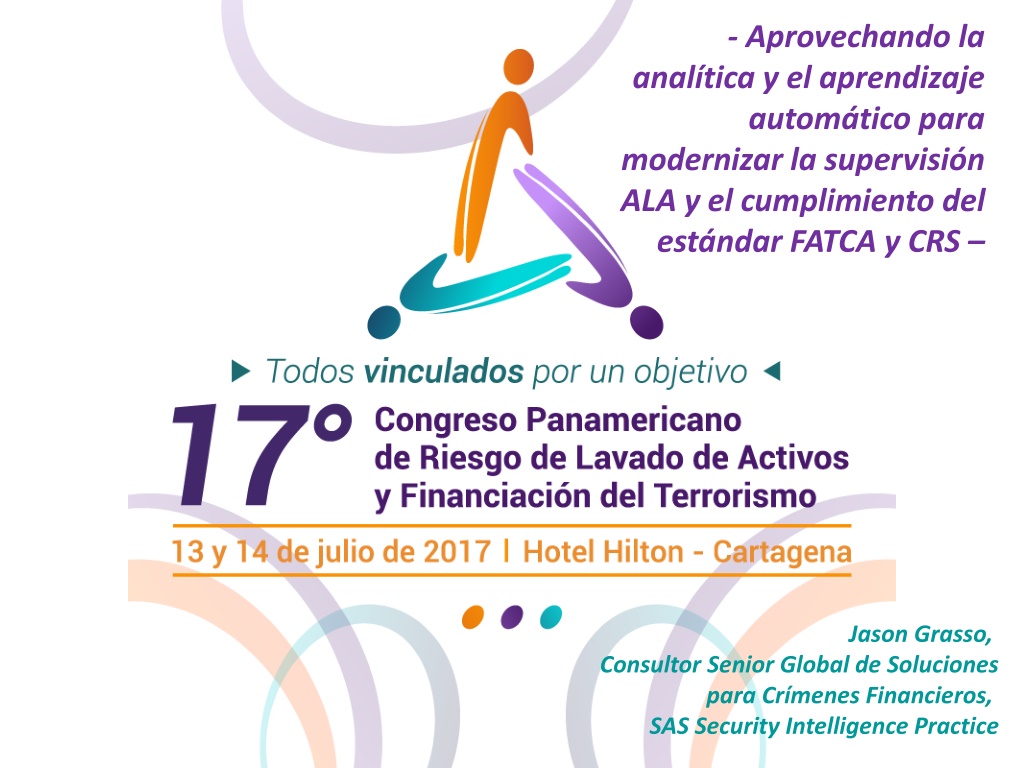
Modernizing Supervision for Compliance with FATCA and CRS
Leveraging analytics and machine learning to modernize supervision and ensure compliance with FATCA and CRS standards. Explore key challenges, best practices, and strategies in the realm of financial crime risk management. Discover how a hybrid approach to financial crimes analytics can enhance anomaly detection and predictive analytics for a holistic customer view.
Download Presentation

Please find below an Image/Link to download the presentation.
The content on the website is provided AS IS for your information and personal use only. It may not be sold, licensed, or shared on other websites without obtaining consent from the author. If you encounter any issues during the download, it is possible that the publisher has removed the file from their server.
You are allowed to download the files provided on this website for personal or commercial use, subject to the condition that they are used lawfully. All files are the property of their respective owners.
The content on the website is provided AS IS for your information and personal use only. It may not be sold, licensed, or shared on other websites without obtaining consent from the author.
E N D
Presentation Transcript
- Aprovechando la anal tica y el aprendizaje autom tico para modernizar la supervisi n ALA y el cumplimiento del est ndar FATCA y CRS Jason Grasso, Consultor Senior Global de Soluciones para Cr menes Financieros, SAS Security Intelligence Practice
CONVERGENCE OF FINANCIAL CRIME AML, CDD, FATCA, CRS Chartis Finanical Crime Risk Management Systems 2016
DATA SHARING ACROSS FINANCIAL INTELLIGENCE UNITS There is no best view determine who the ultimate data owner(s) will be Prioritize data sources to maximize lift Reporting layer can be a quick win Transactional, Demographics, Device/IP, and Third Party
GLOBAL MONITORING STRATGIES BEST PRACTICES Holistic Customer Monitoring Analytics Early Adopters 2016/2017 Model 2013 Validation 2011 Rules Monitoring 2000s
HYBRID APPROACH TO FINANCIAL CRIMES ANALYTICS ANOMALY DETECTION SOCIAL NETWORK ANALYSIS PREDICTIVE ANALYTICS 2 3 4 5 1 RULES TEXT MINING Suitable for Known Patterns Boolean logic Easy to deploy Prone to high false positive rates Suitable for Unknown Patterns Behavioral analysis Profile analysis Peer based anomaly detection, i.e. segmentation Suitable for complex patterns Numerous variables Supervised learning where target variable is known Neural networks Decision trees Regression Suitable for associative link patterns Demographic links Transactional links Hidden relationships Geographic analysis Necessary for unstructured data Contextual analysis Sentiment analysis Social media Simple thresholds, e.g. large wires , high risk geographies ; cash structuring Small business monitoring; commercial ACH activity; online session analysis Credit/debit card fraud; P2P payments fraud; funds availability models 1st Party, bust-out fraud; organized crime rings Insider threat; sanctions compliance; electronic communications Known Unknown Unknowable
USE CASE ENTITY RESOLUTION AND DATA QUALITY Designed to integrate brokerage and consumer data Fuzzy matching techniques Identified duplicate SSNs and EINs and other data elements Consolidated entity records to view holistic customer profile Leverage resolution and aggregation results across your Financial Crimes applications
USE CASE - CUSTOMER SEGMENTATION Notice that the majority of the alerts are being generated for the largest customers. However these groups of customers represent a relatively small percent of the total population and have the lowest productivity rate experience. Prior Alerts Using the Old Segmentation Model Current Percent of Population N/A N/A Analyst Reviewed Alerts 3,709 1,044 4,753 Segment Alert Percentage N/A N/A Number Productive Productivity Rate Scenarios 1. Personal 2. Commercial Grand Total 164 69 233 4.4% 6.6% 4.9% Prior Alerts Mapped onto New Segmentation Model Current Percent of Population Analyst Reviewed Alerts Segment Alert Percentage Number Productive Productivity Rate Scenarios Personal 1. Low Activity 2. Consumer (Small) 3. Consumer (Medium) 4. Consumer (Large) 5. High Risk (Small / Medium) 8. High Risk (Large) 9. Unclassified (Personal) Total Commercial 2% 38% 24% 8% 23% 5% 0% 100% 22 10 338 3,083 18 222 16 3,709 1% 0% 9% 83% 0% 6% 0% 100% 2 3 9.1% 30.0% 8.6% 3.5% 27.8% 8.1% 0.0% 4.4% 29 107 5 18 0 164 10.Business (Small / Medium) 11.Business (Large) 12.Money Service Businesses 13.Non-Profit Organizations 14.Unclassified (Commercial) Total Grand Total 76% 9% 7% 8% 0% 100% 18 952 41 26 7 1,044 4,753 2% 91% 4% 2% 1% 100% 5 27.8% 5.5% 19.5% 15.4% 0.0% 6.6% 4.9% 52 8 4 0 69 233
USE CASE - POST CUSTOMER SEGMENTATION Alerts Generated Under New Segmentation Model Current Percent of Population Analyst Reviewed Alerts Segment Alert Percentage Number Productive Productivity Rate Scenarios Personal 1. Low Activity 2. Consumer (Small) 3. Consumer (Medium) 4. Consumer (Large) 5. High Risk (Small / Medium) 8. High Risk (Large) 9. Unclassified (Personal) Total Commercial 2% 38% 24% 8% 23% 5% 0% 100% 80 2% 40% 25% 2% 23% 7% 1% 100% 6 7.5% 26.4% 8.3% 8.8% 28.3% 6.1% 17.5% 20.1% 1,601 1,001 80 921 280 40 4,003 423 83 7 261 17 7 804 The revised segmentation model produced a 4 fold increase in the scenario s productivity rate (from 4.9% to 20.5%). 10.Business (Small / Medium) 11.Business (Large) 12.Money Service Businesses 13.Non-Profit Organizations 14.Unclassified (Commercial) Total Grand Total 76% 9% 7% 8% 0% 100% 901 66 199 159 4 1,329 5,332 68% 5% 15% 12% 0% 100% 216 2 42 31 0 291 1,095 24.0% 3.0% 21.1% 19.5% 0.0% 21.9% 20.5%
USE CASE - FUNNEL ACCOUNT ANALYSIS, BIG DATA VISUALIZATION
MACHINE LEARNING AND INDUSTRY TRENDS Machine Learning Method of data analysis that automates analytical model building. Using algorithms that iteratively learn from data, machine Method of data analysis that automates analytical model building. Using algorithms that iteratively learn from data, machine learning allows computers to find hidden insights without being explicitly programmed where to look. Machine learning allows computers to find hidden insights without being explicitly programmed where to look. Industry Trends Build models quickly & cheaply fail fast Myth that it solves data quality issues These methods have been used in fraud for decades Potential to auto-decision escalation of items to investigation
CONCLUSION BIG DATA FOR FINANCIAL CRIMES Proven to improve accuracy of detection and reduce false positives In-memory visualization tools are critical to understand emerging patterns and trends in your book of business Assimilate data scientists into your Financial Intelligence Units, security departments, etc. Break down silos of fraud, compliance, security use data sharing to provide contextual awareness Ability to know your customers/counterparties is a competitive advantage in a digital world