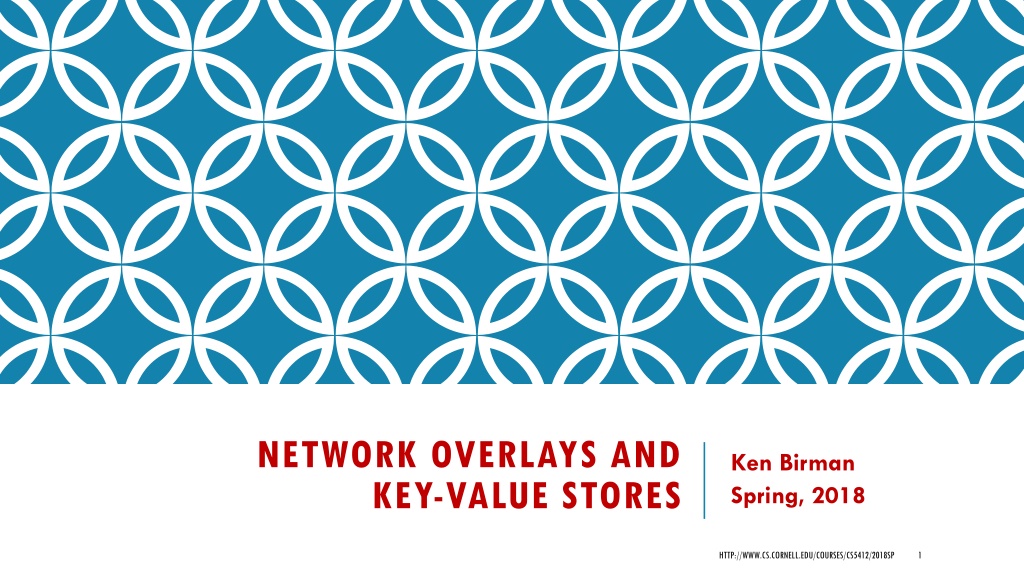
Key-Value Stores and Overlay Networks Overview
Explore the concepts of key-value stores, overlay networks, and distributed hash tables in the context of database scaling. Delve into topics such as transactions, replication, consistency, and more from lectures by Ken Birman at Cornell University. Understand the importance and functionality of overlay networks in spreading DHTs across multiple machines in various network environments.
Download Presentation

Please find below an Image/Link to download the presentation.
The content on the website is provided AS IS for your information and personal use only. It may not be sold, licensed, or shared on other websites without obtaining consent from the author. If you encounter any issues during the download, it is possible that the publisher has removed the file from their server.
You are allowed to download the files provided on this website for personal or commercial use, subject to the condition that they are used lawfully. All files are the property of their respective owners.
The content on the website is provided AS IS for your information and personal use only. It may not be sold, licensed, or shared on other websites without obtaining consent from the author.
E N D
Presentation Transcript
NETWORK OVERLAYS AND KEY-VALUE STORES Ken Birman Spring, 2018 HTTP://WWW.CS.CORNELL.EDU/COURSES/CS5412/2018SP 1
A 5-LECTURE ROADMAP! Lecture 1: Transactions Lecture 3: Lecture 3: Transactions on DHTs Transactions on DHTs Lecture 2: DHTs Lecture 2: DHTs Lecture 4: FaRM RDMA DHT Lecture 5: HeRD and FASST (Old topic) RDMA (Old topic) RDMA HTTP://WWW.CS.CORNELL.EDU/COURSES/CS5412/2018SP 2
RECAP In lecture 1, we saw the big picture, and learned about caching, and CAP This led us down a long path: to understand consistency in terms of , to see how this leads to consistency in a file system. Then we looked at consistent data replication, and then the transactional ACID model. Today, we revert to lecture 1 and look more carefully at the DHT concept. HTTP://WWW.CS.CORNELL.EDU/COURSES/CS5412/2018SP 3
WHEN WE SCALE DATABASES, WE GET BIG KEY-VALUE DATA COLLECTIONS We saw this in lecture 1 We called it a distributed hash table. But we didn t spend enough time to look closely. How do these work? Can you run transactions on them? Answering these questions will actually require five lectures! HTTP://WWW.CS.CORNELL.EDU/COURSES/CS5412/2018SP 4
OVERLAY NETWORKS We use the term network overlay when one network (or a network-like data structure) is superimposed upon an underlying network A key-value store is one of many examples of a network overlay The case we ll focus on is the DHT concept mentioned earlier. Can we create a DHT that spreads over a large number of machines? We will consider two cases: WAN network and inside one datacenter Other network overlays (ones we won t discuss): BitTorrent, CDNs, virtually private networks, enterprise VLAN networks, specialized routing policies, tunnels . CS5412 SPRING 2014 (CLOUD COMPUTING: BIRMAN) 5
KEY-VALUE STORAGE: A DEEP DIVE Previously we encountered the DHT/Key-Value concept when discussing tier two caching in the cloud But where did this concept originate? How can it be used in wide-area settings? When people specialized it for the cloud, exactly how was it changed? What are limitations on key-value storage today? HTTP://WWW.CS.CORNELL.EDU/COURSES/CS5412/2018SP 6
TECHNICAL ISSUE What s the very best way for a massive collection of computers in the wide-area Internet (the WAN) to implement these two aspects Best way to do search? Best way to implement peer-to-peer downloads? DHT: We are searching for the value associated with some key Useful even within a single data center Values are often limited to small things (numbers, or URLs). One can definitely have a value that could be a large thing (photo, video ) but this requires tools optimized to store and fetch large chunks of data. CS5412 SPRING 2014 (CLOUD COMPUTING: BIRMAN) 7
CONTEXT We have a vast number of machines (millions) Goal is to support (key,value) operations Put(key,value) stores this value in association with key Get(key) finds the value currently bound to this key Some systems allow updates, some allow multiple bindings for a single key. We won t worry about those kinds of detail today CS5412 SPRING 2014 (CLOUD COMPUTING: BIRMAN) 8
P2P ENVIRONMENT Nodes come and go at will (possibly quite frequently---a few minutes) Nodes have heterogeneous capacities Bandwidth, processing, and storage Nodes may behave badly Promise to do something (store a file) and not do it (free-loaders) Attack the system CS5412 SPRING 2014 (CLOUD COMPUTING: BIRMAN) 9
BASICS OF ALL DHTS Goal is to build some structured overlay network with the following characteristics: Node IDs can be mapped to the hash key space Given a hash key as a destination address , you can route through the network to a given node Always route to the same node no matter where you start from 127 13 111 97 33 81 58 10 CS5412 SPRING 2014 (CLOUD COMPUTING: BIRMAN)
SIMPLE EXAMPLE (DOESNT SCALE) Circular number space 0 to 127 127 Routing rule is to move counter-clockwise until current node ID key, and last hop node ID < key 13 111 97 33 Example: key = 42 81 58 Obviously you will route to node 58 from no matter where you start CS5412 SPRING 2014 (CLOUD COMPUTING: BIRMAN) 11
BUILDING ANY DHT Newcomer always starts with at least one known member 127 13 111 97 33 81 58 24 CS5412 SPRING 2014 (CLOUD COMPUTING: BIRMAN) 12
BUILDING ANY DHT Newcomer always starts with at least one known member 127 13 111 Newcomer searches for self in the network hash key = newcomer s node ID Search results in a node in the vicinity where newcomer needs to be 97 33 81 58 24 CS5412 SPRING 2014 (CLOUD COMPUTING: BIRMAN) 13
BUILDING ANY DHT Newcomer always starts with at least one known member 127 13 111 Newcomer searches for self in the network hash key = newcomer s node ID Search results in a node in the vicinity where newcomer needs to be 24 97 33 81 58 Links are added/removed to satisfy properties of network CS5412 SPRING 2014 (CLOUD COMPUTING: BIRMAN) 14
BUILDING ANY DHT Newcomer always starts with at least one known member Newcomer searches for self in the network hash key = newcomer s node ID Search results in a node in the vicinity where newcomer needs to be Links are added/removed to satisfy properties of network Objects that now hash to new node are transferred to new node 127 13 111 24 97 33 81 58 CS5412 SPRING 2014 (CLOUD COMPUTING: BIRMAN) 15
INSERTION/LOOKUP FOR ANY DHT Hash name of object to produce key Well-known way to do this 127 13 111 Use key as destination address to route through network Routes to the target node 24 97 33 Insert object, or retrieve object, at the target node 81 58 foo.htm 93 CS5412 SPRING 2014 (CLOUD COMPUTING: BIRMAN) 16
PROPERTIES OF MOST DHTS Memory requirements grow (something like) logarithmically with N Unlike our any DHT , where routing is linear in N, real DHTs have worst possible routing path length (something like) logarithmic with N Cost of adding or removing a node grows (something like) logarithmically with N Has caching, replication, etc CS5412 SPRING 2014 (CLOUD COMPUTING: BIRMAN) 17
DHT ISSUES Resilience to failures Load Balance Heterogeneity Number of objects at each node Routing hot spots Lookup hot spots Locality (performance issue) Churn (performance and correctness issue) Security CS5412 SPRING 2014 (CLOUD COMPUTING: BIRMAN) 18
WERE GOING TO LOOK AT FOUR DHTS At varying levels of detail CAN (Content Addressable Network) ACIRI (now ICIR) Chord MIT Kelips Cornell Pastry Rice/Microsoft Cambridge CS5412 SPRING 2014 (CLOUD COMPUTING: BIRMAN) 19
THINGS WERE GOING TO LOOK AT What is the structure? How does routing work in the structure? How does it deal with node departures? How does it scale? How does it deal with locality? What are the security issues? CS5412 SPRING 2014 (CLOUD COMPUTING: BIRMAN) 20
CAN STRUCTURE IS A CARTESIAN COORDINATE SPACE IN A D DIMENSIONAL TORUS 1 CAN graphics care of Santashil PalChaudhuri, Rice Univ CS5412 SPRING 2014 (CLOUD COMPUTING: BIRMAN) 21
SIMPLE EXAMPLE IN TWO DIMENSIONS 1 2 CS5412 SPRING 2014 (CLOUD COMPUTING: BIRMAN) 22
NOTE: TORUS WRAPS ON TOP AND SIDES 3 1 2 CS5412 SPRING 2014 (CLOUD COMPUTING: BIRMAN) 23
EACH NODE IN CAN NETWORK OCCUPIES A SQUARE IN THE SPACE 3 1 4 2 CS5412 SPRING 2014 (CLOUD COMPUTING: BIRMAN) 24
WITH RELATIVELY UNIFORM SQUARE SIZES CS5412 SPRING 2014 (CLOUD COMPUTING: BIRMAN) 25
NEIGHBORS IN CAN NETWORK Neighbor is a node that: Overlaps d-1 dimensions Abuts along one dimension 26 CS5412 SPRING 2014 (CLOUD COMPUTING: BIRMAN)
ROUTE TO NEIGHBORS CLOSER TO TARGET Z4 Z3 Zn Z2 Z1 d-dimensional space (a,b) n zones Zone is space occupied by a square in one dimension Avg. route path length (d/4)(n 1/d) Number neighbors = O(d) (x,y) Tunable (vary d or n) Can factor proximity into route decision 27 CS5412 SPRING 2014 (CLOUD COMPUTING: BIRMAN)
CHORD USES A CIRCULAR ID SPACE Key ID Node ID K5, K10 N10 K100 N100 Circular ID Space K11, K30 N32 K65, K70 N80 K33, K40, K52 N60 Successor: node with next highest ID Chord slides care of Robert Morris, MIT CS5412 SPRING 2014 (CLOUD COMPUTING: BIRMAN) 28
BASIC LOOKUP N5 N10 N110 Where is key 50? N20 N99 Key 50 is At N60 N32 N40 N80 N60 Lookups find the ID s predecessor Correct if successors are correct CS5412 SPRING 2014 (CLOUD COMPUTING: BIRMAN) 29
SUCCESSOR LISTS ENSURE ROBUST LOOKUP 10, 20, 32 N5 20, 32, 40 N10 5, 10, 20 N110 32, 40, 60 N20 110, 5, 10 N99 40, 60, 80 N32 60, 80, 99 N40 99, 110, 5 N80 80, 99, 110 N60 Each node remembers r successors Lookup can skip over dead nodes to find blocks Periodic check of successor and predecessor links CS5412 SPRING 2014 (CLOUD COMPUTING: BIRMAN) 30
CHORD FINGER TABLE ACCELERATES LOOKUPS To build finger tables, new node searches for the key values for each finger To do it efficiently, new nodes obtain successor s finger table, and use as a hint to optimize the search 1/8 1/16 1/32 1/64 1/128 N80 CS5412 SPRING 2014 (CLOUD COMPUTING: BIRMAN) 31
CHORD LOOKUPS TAKE O(LOG N) HOPS N5 N10 K19 N110 N20 N99 N32 Lookup(K19) N80 N60 CS5412 SPRING 2014 (CLOUD COMPUTING: BIRMAN) 32
DRILL DOWN ON CHORD RELIABILITY Interested in maintaining a correct routing table (successors, predecessors, and fingers) Primary invariant: correctness of successor pointers Fingers, while important for performance, do not have to be exactly correct for routing to work Algorithm is to get closer to the target Successor nodes always do this CS5412 SPRING 2014 (CLOUD COMPUTING: BIRMAN) 33
MAINTAINING SUCCESSOR POINTERS Periodically run stabilize algorithm Finds successor s predecessor Repair if this isn t self This algorithm is also run at join Eventually routing will repair itself Fix_finger also periodically run For randomly selected finger CS5412 SPRING 2014 (CLOUD COMPUTING: BIRMAN) 34
INITIAL: 25 WANTS TO JOIN CORRECT RING (BETWEEN 20 AND 30) 20 20 20 25 25 30 30 25 30 25 finds successor, and tells successor (30) of itself 20 runs stabilize : 20 asks 30 for 30 s predecessor 30 returns 25 20 tells 25 of itself CS5412 SPRING 2014 (CLOUD COMPUTING: BIRMAN) 35
THIS TIME, 28 JOINS BEFORE 20 RUNS STABILIZE 20 20 20 28 25 28 25 30 25 30 28 30 28 finds successor, and tells successor (30) of itself 20 runs stabilize : 20 asks 30 for 30 s predecessor 30 returns 28 20 tells 28 of itself CS5412 SPRING 2014 (CLOUD COMPUTING: BIRMAN) 36
20 20 20 25 25 28 28 25 28 30 30 25 runs stabilize 30 20 runs stabilize CS5412 SPRING 2014 (CLOUD COMPUTING: BIRMAN) 37
CHORD SUMMARY Ring with a kind of binary-search Self-repairing and self-organizing Depends on having a good hash function; otherwise some nodes might end up with many (key,value) pairs and others with few of them CS5412 SPRING 2014 (CLOUD COMPUTING: BIRMAN) 38
CHORD CAN MALFUNCTION IF THE NETWORK PARTITIONS Transient Network Partition USA Europe 0 0 255 255 30 30 248 248 241 64 241 64 202 202 199 108 199 108 177 177 123 123 CS5412 SPRING 2014 (CLOUD COMPUTING: BIRMAN) 39
CHORD HAS NO SENSE OF INTEGRITY The system doesn t know it should be a ring... so it won t detect that it isn t a ring! MIT solution is to make this very unlikely using various tricks, and they work But an attacker might be able to force Chord into a partitioned state and if so, it would endure CS5412 SPRING 2014 (CLOUD COMPUTING: BIRMAN) 40
SO, WHO CARES? Chord lookups can fail and it suffers from high overheads when nodes churn Loads surge just when things are already disrupted quite often, because of loads And can t predict how long Chord might remain disrupted once it gets that way Worst case scenario: Chord can become inconsistent and stay that way CS5412 SPRING 2014 (CLOUD COMPUTING: BIRMAN) 41
MORE ISSUES Suppose my machine has a (key,value) pair and your machine, right in this room, needs it. Search could still take you to Zimbabwe, Lima, Moscow and Paris first! Chord paths lack locality hence can be very long, and failures that occur, if any, will disrupt the system CS5412 SPRING 2014 (CLOUD COMPUTING: BIRMAN) 42
KELIPS: BEST OF ALL (BUT NOBODY USES IT) Network partitioned into N affinity groups Hash of node ID determines which affinity group a node is in Each node knows: One or more nodes in each group All objects and nodes in own group But this knowledge is soft-state, spread through peer-to-peer gossip (epidemic multicast)! CS5412 SPRING 2016 (CLOUD COMPUTING: BIRMAN) 43
RATIONALE? Kelips has a completely predictable behavior under worst-case conditions It may do better but won t do worse Bounded message sizes and rates that never exceed what the administrator picks no matter how much churn occurs Main impact of disruption: Kelips may need longer before Get is guaranteed to return value from prior Put with the same key CS5412 SPRING 2016 (CLOUD COMPUTING: BIRMAN) 44
KELIPS 110 knows about other members 230, 30 Affinity Groups: peer membership thru consistent hash Affinity group view id hbeat rtt 30 234 90ms N 0 1 2 1 230 322 30ms 110 N 230 202 members per affinity group 30 Affinity group pointers CS5412 SPRING 2016 (CLOUD COMPUTING: BIRMAN) 45
KELIPS 202 is a contact for 110 in group 2 Affinity Groups: peer membership thru consistent hash Affinity group view id hbeat rtt 30 234 90ms N 0 1 2 1 230 322 30ms 110 N Contacts 230 202 members per affinity group group contactNode 30 2 202 Contact pointers CS5412 SPRING 2016 (CLOUD COMPUTING: BIRMAN) 46
KELIPS cnn.com maps to group 2. So 110 tells group 2 to route inquiries about cnn.com to it. Affinity Groups: peer membership thru consistent hash Affinity group view id hbeat rtt 30 234 90ms N 0 1 2 1 230 322 30ms 110 N Contacts 230 202 members per affinity group group contactNode 30 2 202 Resource Tuples Gossip protocol replicates data cheaply resource info cnn.com 110 CS5412 SPRING 2016 (CLOUD COMPUTING: BIRMAN) 47
HOW IT WORKS Kelips is entirely gossip based! Gossip about membership Gossip to replicate and repair data Gossip about last heard from time used to discard failed nodes Gossip channel uses fixed bandwidth fixed rate, packets of limited size CS5412 SPRING 2016 (CLOUD COMPUTING: BIRMAN) 48
GOSSIP 101 Suppose that I know something I m sitting next to Fred, and I tell him Now 2 of us know Later, he tells Mimi and I tell Anne Now 4 This is an example of a push epidemic Push-pull occurs if we exchange data CS5412 SPRING 2016 (CLOUD COMPUTING: BIRMAN) 49
GOSSIP SCALES VERY NICELY Participants loads independent of size Network load linear in system size Information spreads in log(system size) time 1.0 % infected 0.0 Time CS5412 SPRING 2016 (CLOUD COMPUTING: BIRMAN) 50