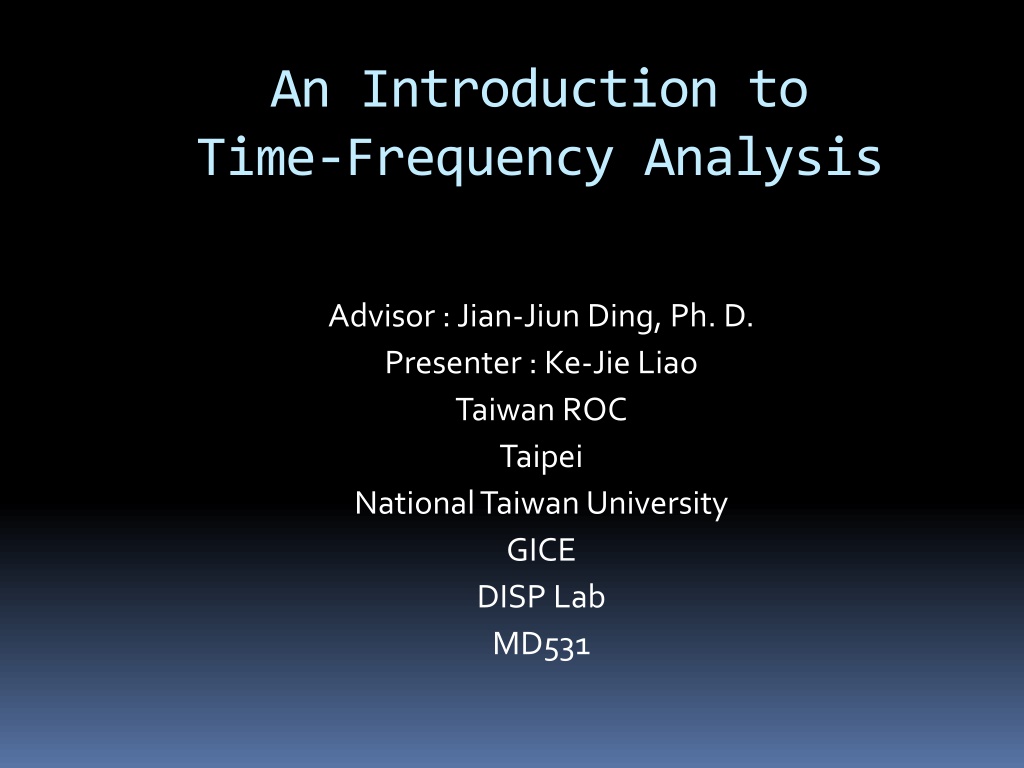
Exploring Time-Frequency Analysis Techniques
Uncover the intricacies of time-frequency analysis through topics like Short Time Fourier Transform, Fourier Transform variations, and applications in signal decomposition and filter design. Understand the significance of frequency in signal processing and the evolution from conventional Fourier transform methods to more advanced techniques. Explore the impact of window functions on controlling time and frequency resolution in analysis.
Download Presentation

Please find below an Image/Link to download the presentation.
The content on the website is provided AS IS for your information and personal use only. It may not be sold, licensed, or shared on other websites without obtaining consent from the author. If you encounter any issues during the download, it is possible that the publisher has removed the file from their server.
You are allowed to download the files provided on this website for personal or commercial use, subject to the condition that they are used lawfully. All files are the property of their respective owners.
The content on the website is provided AS IS for your information and personal use only. It may not be sold, licensed, or shared on other websites without obtaining consent from the author.
E N D
Presentation Transcript
An Introduction to Time-Frequency Analysis Advisor : Jian-Jiun Ding, Ph. D. Presenter : Ke-Jie Liao Taiwan ROC Taipei National Taiwan University GICE DISP Lab MD531
Outline Introduction STFT Rectangular STFT Gabor Transform Wigner Distribution Function Motions on the Time-Frequency Distribution FRFT LCT Applications on Time-Frequency Analysis Signal Decomposition and Filter Design Sampling Theory Modulation and Multiplexing 2
Introduction Frequency? Another way to consider things. Frequency related applications FDM Sampling Filter design , etc . 3
Introduction Conventional Fourier transform 1-D Totally losing time information Suitable for analyzing stationary signal ,i.e. frequency does not vary with time. [1] 4
Introduction Time-frequency analysis Mostly originated form FT Implemented using FFT [1] 5
Short Time Fourier Transform Modification of Fourier Transform Sliding window, mask function, weighting function Mathematical expression ( ) ( , ) ( ) X t f x w t w(t) = = ( )} 2 j f { ( ) e d F w t x Reversing Shifting FT 6
Short Time Fourier Transform Requirements of the mask function w(t) is an even function. i.e. w(t)=w(-t). max(w(t))=w(0),w(t1) when |t| is large. An example of window functions w(t2) if |t1|<|t2|. w t ( ) 0 t Window width K 7
Short Time Fourier Transform Requirements of the mask function w(t) is an even function. i.e. w(t)=w(-t). max(w(t))=w(0),w(t1) when |t| is large. An illustration of evenness of mask functions w(t2) if |t1|<|t2|. w t ( ) 0 Mask Signal t0 8
Short Time Fourier Transform Effect of window width K Controlling the time resolution and freq. resolution. Small K Better time resolution, but worse in freq. resolution Large K Better freq. resolution, but worse in time resolution 9
Short Time Fourier Transform The time-freq. area of STFTs are fixed f f K decreases t t 10
Rectangular STFT Rectangle as the mask function Uniform weighting Definition Forward 1 + t B = ( ) 2B 2 j f ( , ) X t f x e d t B Inverse = where 2 j ft ( ) x t ( , ) X t f e df + t B t t B 1 1 11
Rectangular STFT Examples of Rectangular STFTs cos(4 ) ,0 ( ) cos(2 ) ,10 cos( ) t f 2 ,0 1 ,10 0.5 10 20 10 t t t = = ( ) f t t 20 30 x t t t i ,20 30 t ,20 t f B=0.25 B=0.5 2 1 0 2 1 0 t t 30 0 10 20 30 0 10 20 12
Rectangular STFT Examples of Rectangular STFTs cos(4 ) ,0 ( ) cos(2 ) ,10 cos( ) t f 2 ,0 1 ,10 0.5 10 20 t 10 t t t t = = ( ) f t t 20 30 x t t i ,20 30 ,20 t f B=1 B=3 2 1 0 2 1 0 t t 30 0 10 20 30 0 10 20 13
Rectangular STFT Properties of rec-STFTs Linearity = + ( ) ( , ) H t f ( ) x t = ( ) y t h t + ( , ) Y t f ( , ) X t f Shifting t B + = 2 2 j f j f ( ) ( , ) f e x e d X t 0 0 0 t B Modulation t B + = 2 2 j f j f [ ( ) x ] ( , ) e e d X t f f 0 0 t B 14
Rectangular STFT Properties of rec-STFTs Integration + ( ), x v v B t v B = 2 j fv ( , ) X t f e df 0 , otherwise Power integration t B + = ( ) ( ) * * ( , ) X t f ( , ) Y t f df x y d t B Energy sum = * * ( , ) X t f ( , ) ( ) ( ) Y t f dfdt B x y d 15
Gabor Transform Gaussian as the mask function ( ) e w t = 2 t Mathematical expression 1.9143 + t 2 2 = ( ) ( ) ( ) 2 ( ) 2 t j f t j f ( , ) G t f e e x d e e x d x 1.9 143 t Since 2 | | 1.9143 a a 0.00001 e where GT s time-freq area is the minimal against other STFTs! 16
Gabor Transform Compared with rec-STFTs Window differences Resolution The GT has better clarity Complexity 17
Gabor Transform Compared with rec-STFTs Resolution GT has better clarity Example of y t 2 = t ( ) e f f The rec-STFT The GT 0 0 t t 0 0 18
Gabor Transform Compared with the rec-STFTs Window differences Resolution GT has better clarity Example of y t 2 = t ( ) e f The rec-STFT The GT 0 0 t t 0 0 19
Gabor Transform Properties of the GT Linearity = + ( ) ( ) ( , ) z G t f ( ) z x = y + ( , ) G t f ( , ) G t f x y Shifting = 2 j f t )( , ) t ( , ) f e G f G t t 0 0 x ( x t t 0 Modulation = ( , ) t ( , ) G f G t f f 0 x 2 j f t 0 ( ) x t e 20
Gabor Transform Properties of the GT Integration 2 2 t = 2 ( 1) j k tf k K=1-> recover original signal ( , ) G t f e ( ) x kt df e x Power integration Energy sum Power decayed 21
Gabor Transform Gaussian function centered at origin = 1 2 2 = = t t ( ) ( ) w t e w t e Generalization of the GT Definition 1.91 43 + t 0 2 2 = ( ) ( ) ( ) 2 ( ) 2 t j f t j f ( , ) G t f e e x d e e x d x 1.9143 t 22
Gabor Transform plays the same role as K,B.(window width) increases -> window width decreases decreases -> window width increases Examples : Synthesized cosine wave f f = = 0.1 1 2 2 1 1 0 0 t t 30 0 10 20 30 0 10 20 23
Gabor Transform plays the same role as K,B.(window width) increases -> window width decreases decreases -> window width increases Examples : Synthesized cosine wave f = 1.5 f = 5 2 2 1 1 0 0 t t 30 0 10 20 30 0 10 20 24
Wigner Distribution Function Definition W t f = + = + * 2 * j f ( , ) ( ) ( ) { ( ) ( ) } x t x t e d F x t x t x 2 2 2 2 Auto correlated -> FT Good mathematical properties Autocorrelation Higher clarity than GTs But also introduce cross term problem! 25
Wigner Distribution Function Cross term problem WDFs are not linear operations. ( ) ( ) h t g t = + ( ) s t = + 2 2 ( , ) | W t f | ( , ) | W t f | ( , ) W t f h g s + + + + * * * * 2 j f [ ( ) ( s t ) ( ) ( ) ] g t g t s t e d 2 2 2 2 + ( ) ( ) s t g t + * * s t * * ( ) ( ) g t 26
Wigner Distribution Function An example of cross term problem = f 1 1 5 2 t ( 4) 9 1 t t ( 4 ) t j 9 1 e t 10 2 ( ) x t = ( ) f t i 1( 2 2 2 ( 6 ) t j t + 1 9 e t 6) 1 9 t t f Without cross term With cross term 0 0 t t 0 0 27
Wigner Distribution Function Compared with the GT Higher clarity Higher complexity An example f + 4 4 j t j t e e = = ( ) x t cos(4 ) t 2 f WDF GT 0 0 t t 0 0 28
Wigner Distribution Function But clarity is not always better than GT Due to cross term problem Functions with phase degree higher than 2 = 3 ( ) x t exp( ( 5) 6 ) j t j t f f WDF GT 0 0 t t [1] 0 0 29
Wigner Distribution Function Properties of WDFs Shifting = )( , ) t f ( , ) f W W t t 0 x ( 0 x t t Modulation = )( , ) t f ( , ) W W t f f 0 x 2 j f t 0 e ( x t Energy property = = 2 2 ( , ) | ( )| x t | ( )| X f W t f dtdf dt df x 30
Wigner Distribution Function Properties of WDFs Recovery property Energy property Region property Multiplication Convolution Correlation ( , ) W t f is real x Moment Mean condition frequency and mean condition time 31
Motions on the Time- Frequency Distribution Operations on the time-frequency domain Horizontal Shifting (Shifting on along the time axis) f STFT GT 2 j ft , ( ( ) ) ( , ) f x t t S t t e 0 0 0 x WDF ( , ) f x t t W t t 0 0 x t Vertical Shifting (Shifting on along the freq. axis) f STFT GT 2 j f t , ( ) x t ( , ) e S t f f 0 0 x 2 j f t WDF ( ) x t ( , ) e W t f f 0 0 x t 32
Motions on the Time- Frequency Distribution Operations on the time-frequency domain Dilation 1 | | 1 | | a t t STFT GT , ( ) a ( , a ) x S af x a t t WDF ( ) a ( , a ) x W af x Case 1 : a>1 Case 2 : a<1 33
Motions on the Time- Frequency Distribution Operations on the time-frequency domain Shearing - Moving the side of signal on one direction Case 1 : ( ) ( ) y t e x t = f 2 j at Moving this side a>0 = ( , ) ( , ) y W t f ( , ( , x ) S t f S t f W t a t a t y x ) f t 2 Case 2 : t j = ( ) y t ( ) x t e a a>0 f = ( , ) ( , ) y W t f ( , ) , ) f a S t f S t W t f f a y x ( f Moving this side x t 34
Motions on the Time- Frequency Distribution Rotations on the time-frequency domain Clockwise 90 degrees Using FTs f = ( ) { ( )} FT x t X f ( , )| | ( , ) ( , ) W t f | ( , )| , ) , ) f t S t f S f t X x = = 2 j ft ( ( x G t f G W f t e X x X t 35
Motions on the Time- Frequency Distribution Rotations on the time-frequency domain Generalized rotation with any angles Using WDFs or GTs via the FRFT Definition of the FRFT 2 2 2 csc = = cot cot j u j ut j t ( ) [ ( )] 1 cot ( ) x t du X u O x t j e e e F Additive property + = { [ ( )]} O x t [ ( )] x t O O F F F 36
Motions on the Time- Frequency Distribution Rotations on the time-frequency domain [Theorem] The fractional Fourier transform (FRFT) with angle is equivalent to the clockwise rotation operation with angle for the WDF or GT. = = + + ( , ) ( , ) u v ( cos ( cos G u sin , sin sin , sin cos ) cos ) W G u v W u v v u u v v X x X x Old New New Old ' ' cos sin sin cos cos sin sin cos u u v u v u = = ' ' v v Clockwise rotation matrix Counterclockwise rotation matrix 37
Motions on the Time- Frequency Distribution Rotations on the time-frequency domain [Theorem] The fractional Fourier transform (FRFT) with angle is equivalent to the clockwise rotation operation with angle for the WDF or GT. Examples (Via GTs) 5 5 5 0 0 0 -5 -5 -5 -5 0 5 -5 0 = 5 -5 (c) G (t, ) F = 0 5 (a) G (t, ) f = (b) G (t, ) F [1] 38
Motions on the Time- Frequency Distribution Rotations on the time-frequency domain [Theorem] The fractional Fourier transform (FRFT) with angle is equivalent to the clockwise rotation operation with angle for the WDF or GT. Examples (Via GTs) 5 5 5 0 0 0 -5 -5 -5 -5 (d) G (t, ) F = 0 5 -5 0 5 -5 0 5 = (f) G (t, ) F = (e) G (t, ) F [1] 39
Motions on the Time- Frequency Distribution Twisting operations on the time-frequency domain LCT s Old New = + ( , ) u v ( , ) W W du bv + cu av ' u d b u v X x ( , , , ) a b c d = + = c a ' ( , ) ( , ) W au bv cu dv W u v v X x ( , , , ) a b c d Inverse exist since ad-bc=1 f f a c b d LCT t t 40
Applications on Time-Frequency Analysis Signal Decomposition and Filter Design A signal has several components -> separable in time -> separable in freq. -> separable in time-freq. f t t f 41
Applications on Time-Frequency Analysis Signal Decomposition and Filter Design An example f 1 0t t 1t 2 Rotation -> filtering in the FRFT domain 42
Applications on Time-Frequency Analysis Signal Decomposition and Filter Design An example [1] 43
Applications on Time-Frequency Analysis Signal Decomposition and Filter Design An example 2 2 2 + = + + 0.23 0.3 8.5 0.46 9.6 j t j t j t j t j t ( ) 0.5 0.5 0.5 n t e e e [1] 44
Applications on Time-Frequency Analysis Signal Decomposition and Filter Design An example 2 2 2 + = + + 0.23 0.3 8.5 0.46 9.6 j t j t j t j t j t ( ) 0.5 0.5 0.5 n t e e e [1] 45
Applications on Time-Frequency Analysis Signal Decomposition and Filter Design An example [1] 46
Applications on Time-Frequency Analysis Sampling Theory Nyquist theorem : , B Adaptive sampling 2 sf B [1] 47
Conclusions and Future work Comparison among STFT,GT,WDF rec-STFT GT WDF Complexity Clarity Time-frequency analysis apply to image processing? 48
References [1] Jian-Jiun Ding, Time frequency analysis and wavelet transform class note, the Department of Electrical Engineering, National Taiwan University (NTU), Taipei, Taiwan, 2007. [2] S. C. Pei and J. J. Ding, Relations between Gabor transforms and fractional Fourier transforms and their applications for signal processing , IEEE Trans. Signal Processing, vol.55,no. 10,pp.4839-4850. [3] S. Qianand D. Chen, Joint Time-Frequency Analysis: Methods and Applications, Prentice-Hall, 1996. [4] D. Gabor, Theory of communication , J. Inst. Elec. Eng., vol. 93, pp.429-457, Nov. 1946. [5] L. B. Almeida, The fractional Fourier transform and time-frequency representations, IEEE Trans. Signal Processing, vol. 42,no. 11, pp. 3084- 3091, Nov. 1994. [6] K. B. Wolf, Integral Transforms in Science and Engineering, Ch. 9: Canonical transforms, New York, Plenum Press, 1979. 49
References [7] X. G. Xia, On bandlimited signals with fractional Fourier transform, IEEE Signal Processing Letters, vol. 3, no. 3, pp. 72-74, March 1996. [8] L. Cohen, Time-Frequency Analysis, Prentice-Hall, New York, 1995. [9] T. A. C. M. Classen and W. F. G. Mecklenbrauker, The Wigner distribution a tool for time-frequency signal analysis; Part I, Philips J. Res., vol. 35, pp. 217-250, 1980. 50