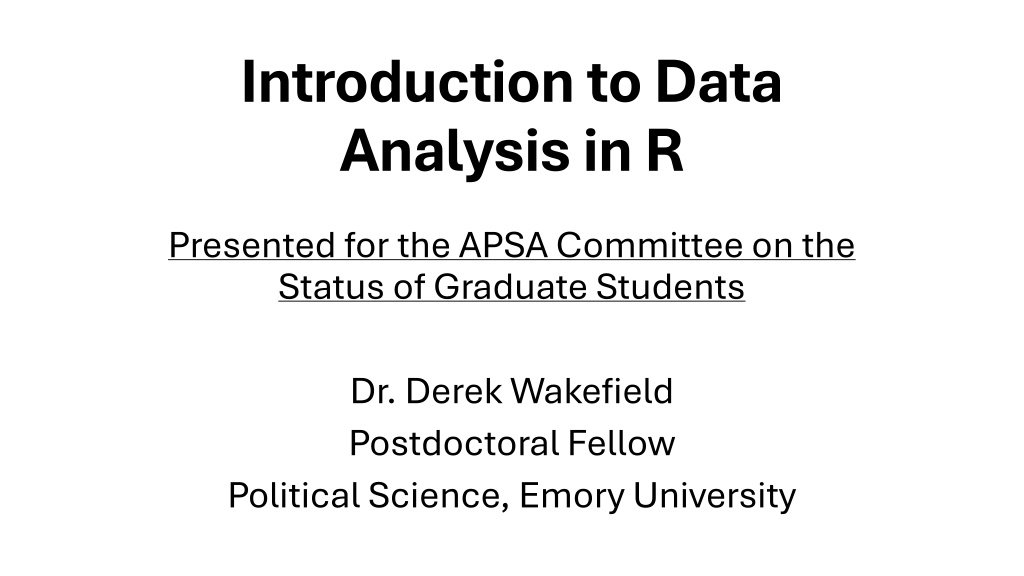
Data Analysis in R: Installation, Basic Concepts, and Commands
Explore the installation of R and RStudio, understand basic R concepts and commands, and learn how to perform arithmetic operations and work with data objects in R. Get started on your data analysis journey in R!
Download Presentation

Please find below an Image/Link to download the presentation.
The content on the website is provided AS IS for your information and personal use only. It may not be sold, licensed, or shared on other websites without obtaining consent from the author. If you encounter any issues during the download, it is possible that the publisher has removed the file from their server.
You are allowed to download the files provided on this website for personal or commercial use, subject to the condition that they are used lawfully. All files are the property of their respective owners.
The content on the website is provided AS IS for your information and personal use only. It may not be sold, licensed, or shared on other websites without obtaining consent from the author.
E N D
Presentation Transcript
Introduction to Data Analysis in R Presented for the APSA Committee on the Status of Graduate Students Dr. Derek Wakefield Postdoctoral Fellow Political Science, Emory University
Installing R and RStudio If you have not already, install R R and RStudio on your computer RStudio https://posit.co/download/rstudio-desktop/ Choose any mirror, closer ones will be faster Have this going in the background while I talk!
Basic R Concepts There are multiple windows open in the editor let s go over each one Active code Data objects Console and terminal Packages, plot viewer, and ?help
Basic R Concepts (continued) Look at top toolbar Change visual look of editor Change working directory Make a new R Notebook file and save it under a new name Need to begin every chunk with ```{r [put title here]} and end with ``` R Notebook is my preferred method of coding because it creates distinct chunks and puts code output beneath each chunk
Basic R Concepts (continued) At the start of every coding task, you will do the following: Identify, install, and load packages Use bottom-right pane to find packages, or use install.packages() Library( Library(rmarkdown rmarkdown) ) Library( Library(tidyverse tidyverse) ) Set working directory, store and load data Create new folder, keeps things cleaner (also look into R Projects) Create new folder, keeps things cleaner (also look into R Projects) Diagnose and clean issues with data before any analysis
Basic Commands in R R can do basic arithmetic with standard computer-math Addition: 1+1 Subtraction: 2-1 Multiplication: 3*2 Division: 4/2 Exponents: ^ Square root: sqrt(vector) Mean: mean(vector) Sum: sum(vector) R uses PEMDAS in order of operations What would this be: (4 + 6) / (2 * (4 2))^2
Basic Commands in R (continued) R uses data objects as the primary form of storing data Create a new single-object data by using <- (or =) smol_data smol_data < <- - 7 7 The object being created or edited is always on the left, and the data that is being stored comes from the right side Look in the data pane and you will now have a smol_data object there Type view( view(smol_data smol_data) )
Basic Commands in R (continued) You can then manipulate the data Try adding 1 to the data Try exponentiating the data by You can make vectors which are longer lists of variables using the c() command Vector1 < Vector1 <- -c(1, 2, 3, 4, 5) c(1, 2, 3, 4, 5) Vector2 < Vector2 <- -c(4,8,10) c(4,8,10) You can create a bigger dataframe (we will discuss something called `tibbles` in the future as well) big_df big_df < <- -data.frame data.frame( (smol_data smol_data, Vector1, Vector2) Why doesn t this work, but only including smol_data and Vector1 works? , Vector1, Vector2)
Basic Commands in R (continued) If you have questions about a given package, you can use ? followed by the package name to open a help panel First, create a new data object using the cars dataset cars_df cars_df < <- - cars cars Try using ?cars (a dataset that comes automatically with R) What is this dataset? What variables are stored? How large is the dataset? How many variables/observations?
The Data Viewer Panel Let s click on the cars_df This is not advisable for large datasets, which means 100+ variables and 10,000+ observations. In that case, use glimpse() cars_df dataframe and view what it is Can manipulate column orders Can view the list of variables and identify obvious issues
Visualizing Data in R Let s load another dataset: mtcars New_table New_table < <- - mtcars mtcars Let s make sure the Rmarkdown package is loaded Library( Library(rmarkdown rmarkdown) ) Library( Library(tidyverse tidyverse) ) Create a nicer-looking output: paged_tables paged_tables( (cars_df glimpse( glimpse(cars_df cars_df) ) cars_df) )
Visualizing Data in R Let s try a basic plot with base R Plot( data = Plot( data = mtcars mtcars, , cyl cyl ~ mpg) ~ mpg) 99% of the time, I m using ggplot (from tidyverse) for these tasks Ggplot Ggplot(data = [ (data = [dataframe_name dataframe_name], ], aes Geom_bar Geom_bar() + (or () + (or Geom_point Geom_point, , Geom_line Geom_line, , geom_raster title() + title() + etc etc etc. etc. aes(x = variable1, y = variable2)) + (x = variable1, y = variable2)) + geom_raster) ) GGPlot functions by adding layers cumulatively that inform R how to use the given variables We will learn much more at the end of the class on this
Visualizing Data in R Dipping our toes into dplyr a bit using the group_by and reframe commands to create diagnostic tables diag_df diag_df < <- - mtcars group_by group_by( (cyl reframe reframe(count = n () ) (count = n () ) mtcars %>% cyl) %>% ) %>% %>% This will take the mtcars dataframe, group by cyl, and get the average mpg for each cyl group. Try it with the HP variable
Visualizing Data in R Lastly, the way that we determine relationships between variables is through linear regressions The basic command for a linear model in R is lm() Lm Lm(data = (data = mtcars the number of cylinders in a car, and its horsepower, across the full dataset mtcars, hp ~ , hp ~ cyl cyl) ) estimates the relationship between We will learn more about this process in a future lecture
Answering Questions about mtcars Use the tools we have learned today and work with your nearby classmates to answer the following questions about the mtcars dataset: 1. How many individual car types are in the dataset? 2. What is the lowest value for mpg? 3. What is the median value for wt? 4. What are the options for the numbers of cyl? 5. Which of the variables are binary (only 0 or 1)? 6. What is the relationship between mpg and cyl? Is it significant? Please raise your hand if you need help
Some last RMarkdownthoughts RMarkdown can be used as a way to present your final results, although I tend to use R Notebooks and LaTeX These documents have to knit which means the entire document needs to run, which can sometimes be more difficult than having individual chunks that create usable outputs You can learn more in the RMarkdown documentation (?RMarkdown)
Your (Easy) Homework Look at the dataset of datasets and begin thinking about which of the final project options you want to take Under Course Modules, see Important Links document Anybody looking to do a solo-author original project (grad student 3rdyear paper, undergraduate honors thesis) Anybody looking to do a co-authored original project? Anybody looking to do a replication project?