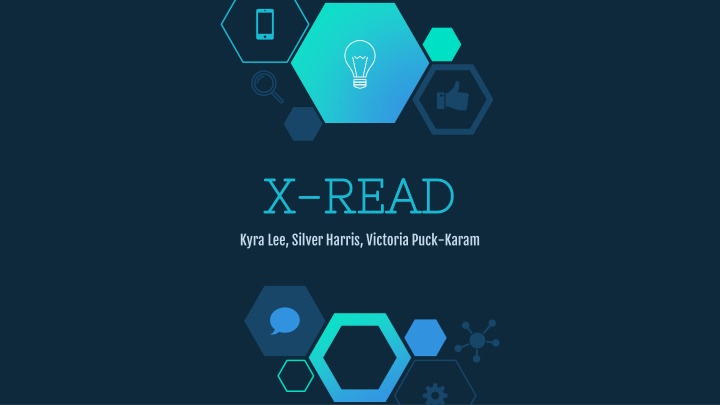
Cutting-Edge AI Training for Disease Recognition
Explore the detailed process of training an AI algorithm to identify various thoracic abnormalities in X-rays. Discover the challenges faced, accuracy rates, and future plans for enhancing the CNN's capabilities.
Download Presentation

Please find below an Image/Link to download the presentation.
The content on the website is provided AS IS for your information and personal use only. It may not be sold, licensed, or shared on other websites without obtaining consent from the author. If you encounter any issues during the download, it is possible that the publisher has removed the file from their server.
You are allowed to download the files provided on this website for personal or commercial use, subject to the condition that they are used lawfully. All files are the property of their respective owners.
The content on the website is provided AS IS for your information and personal use only. It may not be sold, licensed, or shared on other websites without obtaining consent from the author.
E N D
Presentation Transcript
X X- -READ READ Kyra Lee, Silver Harris, Victoria Puck-Karam
DISEASES CHOSEN DISEASES CHOSEN % of American % of American population affected (per population affected (per year) year) Name Name Definition Definition # of .pngs # of .pngs Atelectasis Partial or total collapse of the lung when alveoli deflate or are filled with alveolar fluid 11,559 >.06% Effusion Fluid buildup between tissues that line the lungs and the chest 13,317 >.06% Infiltration Diffusion or accumulation of foreign substances 19,894 7% Mass A build up of cells leading to the formation of a mass 5,782 .001-.3% Nodule A small, palpable mass usually on the epidermis 6,331 .5% 2
TRAINING PROCESS TRAINING PROCESS - - - Each disorder took 10 hours to train Used 10 layers of epochs to make the algorithm more accurate Saved trained models into .h5 files - Able to run trained algorithm remotely, will identify in under a minute Some disorders were harder to identify due to: - Lack of pictures to allocate to training and test - Monochrome nature of x-rays - Some disorders were more visibly obvious (mass) Average val_accuracy: Average val_accuracy: 65-70% Average val_loss: Average val_loss:75-85% - - - 3
WORST ACCURACY: WORST ACCURACY: EFFUSION EFFUSION
BEST ACCURACY: MASS BEST ACCURACY: MASS
AVERAGE ACCURACIES AND LOSSES AVERAGE ACCURACIES AND LOSSES
FUTURE PLANS FUTURE PLANS Create user friendly interface Create program that enhances contrast of X-ray RGB values to make the CNN more accurate Possibly invert colors to do such Use contrast limited adaptive histogram equalization Train remaining 9 cardiothoracic abnormalities Create program to recognize other x-rays Broken bones Fractures Delete the 60,000 photos off the computer to increase memory 7