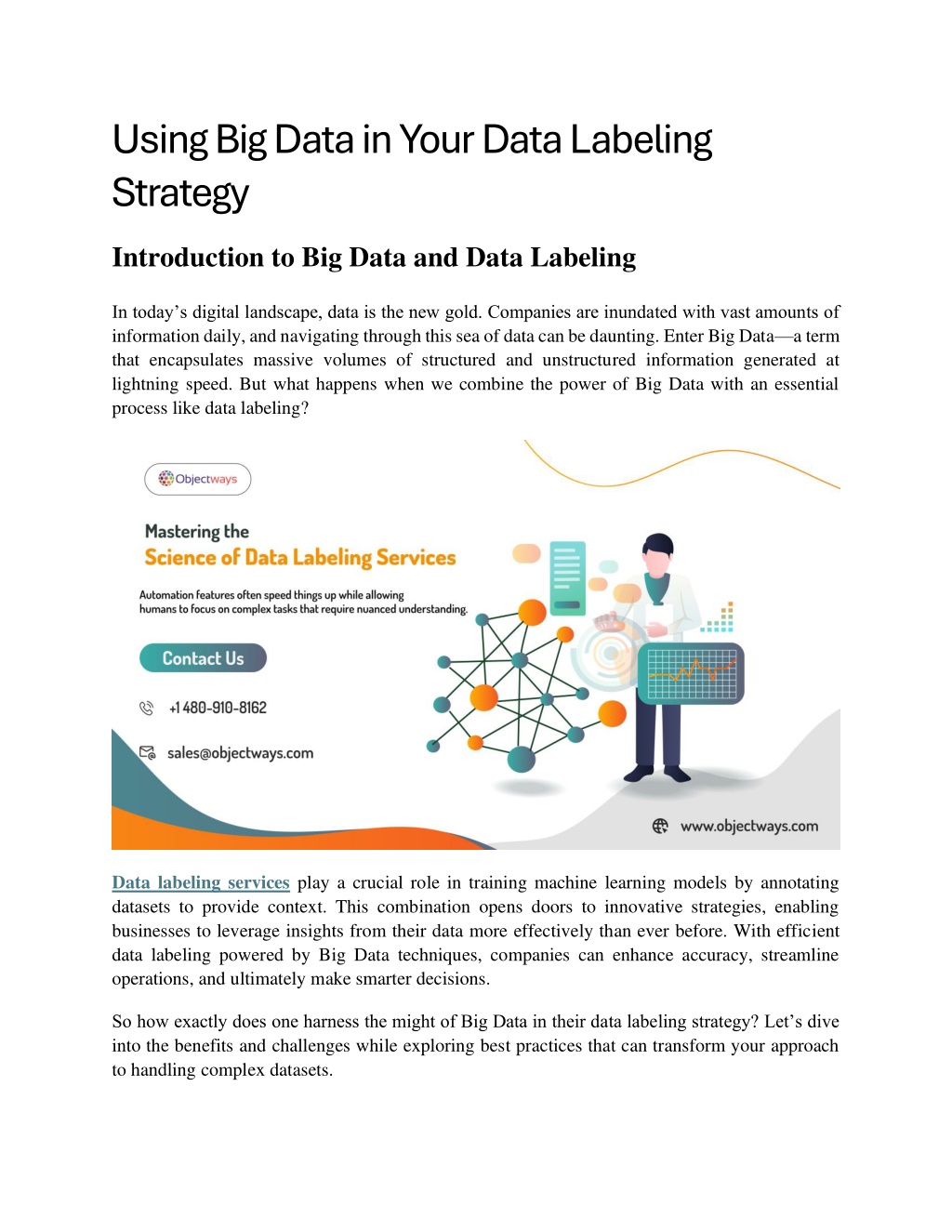
Using Big Data in Your Data Labeling Strategy
Data labeling services play a crucial role in training machine learning models by annotating datasets to provide context.
Download Presentation

Please find below an Image/Link to download the presentation.
The content on the website is provided AS IS for your information and personal use only. It may not be sold, licensed, or shared on other websites without obtaining consent from the author. Download presentation by click this link. If you encounter any issues during the download, it is possible that the publisher has removed the file from their server.
E N D
Presentation Transcript
Using Big Data in Your Data Labeling Strategy Introduction to Big Data and Data Labeling In today s digital landscape, data is the new gold. Companies are inundated with vast amounts of information daily, and navigating through this sea of data can be daunting. Enter Big Data a term that encapsulates massive volumes of structured and unstructured information generated at lightning speed. But what happens when we combine the power of Big Data with an essential process like data labeling? Data labeling services play a crucial role in training machine learning models by annotating datasets to provide context. This combination opens doors to innovative strategies, enabling businesses to leverage insights from their data more effectively than ever before. With efficient data labeling powered by Big Data techniques, companies can enhance accuracy, streamline operations, and ultimately make smarter decisions. So how exactly does one harness the might of Big Data in their data labeling strategy? Let s dive into the benefits and challenges while exploring best practices that can transform your approach to handling complex datasets.
Benefits of Incorporating Big Data in Your Data Labeling Strategy Incorporating Big Data into your data labeling strategy can drastically enhance accuracy. With vast amounts of information, algorithms refine and improve label precision. This leads to better machine learning models. Speed is another significant advantage. Big Data enables the processing of massive datasets quickly. Rapid labeling accelerates project timelines, allowing for faster deployment of AI solutions. Moreover, diversity in data sources enriches training sets. Incorporating varied data types helps create more robust models that perform well across different scenarios. Cost efficiency also emerges as a key benefit. Automating parts of the labeling process with insights from Big Data can reduce manual labor costs significantly. Leveraging analytics allows teams to identify trends and patterns easily. These insights inform strategic decisions that strengthen overall business goals while enhancing model performance. Challenges and Limitations of Using Big Data for Data Labeling Implementing Big Data in data labeling comes with its own set of challenges. One major hurdle is the sheer volume and complexity of data. Sifting through vast datasets can lead to confusion, making it hard to pinpoint relevant information. Data quality is another concern. Not all big data is clean or structured, which complicates the labeling process. Poor-quality data can result in inaccurate labels and undermine AI performance. Additionally, technical expertise is essential. Organizations may struggle if they lack trained personnel who understand both Big Data analytics and effective labeling strategies. Privacy regulations pose significant constraints. Companies must navigate complex legal frameworks that govern how data can be used, especially personal information. This makes compliance a critical factor in leveraging Big Data for accurate labeling outcomes.
Best Practices for Implementing Big Data in Your Data Labeling Process When integrating Big Data into your data labeling company process, start by defining clear objectives. Know what you want to achieve with your labeled data. This focus will streamline the entire workflow. Next, leverage automation tools. They can significantly reduce manual effort and increase efficiency. Automated solutions help in quickly processing vast datasets while maintaining accuracy. Collaboration is key; involve domain experts early on. Their insights ensure that labels are not only accurate but also contextually relevant. Regularly review and refine your processes based on feedback and results. An iterative approach allows for continuous improvement in labeling quality. Invest in training for your team to understand Big Data technologies better. A well- informed team can make smarter decisions throughout the data labeling journey, leading to more effective outcomes. Case Studies: Companies Successfully Utilizing Big Data in Their Data Labeling Strategies Amazon is a prime example of leveraging big data in its data labeling strategies. By analyzing customer behavior and preferences, they enhance product recommendations. This not only improves user experience but also refines their algorithms over time. Another noteworthy example is Tesla, which utilizes big data for autonomous vehicle training. Their fleet collects vast amounts of real-time driving data, allowing the company to label scenarios effectively. This process accelerates the development of self-driving capabilities. In healthcare, IBM Watson Health employs big data analytics for medical imaging tasks. They analyze millions of labeled images to train models that assist radiologists in diagnosing diseases with higher accuracy. These companies showcase how integrating big data into labeling processes can drive innovation and efficiency across various sectors. Each case highlights unique methods tailored to their specific industry needs while enhancing operational workflows significantly.
The Future of Big Data and its Impact on the Field of Data Labeling The future of Big Data is poised to revolutionize data labeling. As technology advances, we will see an exponential increase in the volume and complexity of data generated every day. Machine learning algorithms will become increasingly sophisticated, allowing for more accurate and efficient labeling processes. These tools can analyze vast datasets rapidly, identifying patterns that might elude human annotators. Collaboration between AI and human intelligence will enhance quality control in data labeling service. By leveraging insights from both machines and humans, businesses can ensure higher accuracy rates. Moreover, advancements in automation are set to reduce costs significantly. Companies can allocate resources more effectively while still meeting the demands of large-scale projects. As Big Data continues to evolve, it brings new opportunities for innovation within data labeling strategies. Organizations that adapt will have a competitive edge in harnessing this powerful resource for their needs. Conclusion The integration of big data into your data labeling strategy offers a multitude of advantages. By harnessing vast datasets, companies can enhance accuracy, efficiency, and scalability in their labeling processes. This not only leads to better model performance but also reduces time and costs associated with manual labeling. However, challenges remain. The complexity of managing large datasets can create hurdles that organizations must address. Ensuring data quality and maintaining privacy standards are crucial factors that cannot be overlooked. To successfully implement big data solutions in your data labeling efforts, adopting best practices is essential. It involves establishing clear protocols for dataset management and utilizing advanced technologies that support seamless integration. As more businesses adopt these strategies, the landscape of data labeling continues to evolve. Companies leveraging big data effectively are setting new benchmarks for success in this competitive field.
Looking ahead, the potential impact of big data on the domain of data labeling is immense. As technology advances further, we can expect even greater innovations that will transform how labels are created and utilized across industries enhancing everything from artificial intelligence models to machine learning applications. Embracing these changes ensures you stay at the forefront of technological advancements while optimizing your operations with top-tier Data Labeling Services tailored for today's demands. Reach out to us understand how we can assist with this process - sales@objectways.com