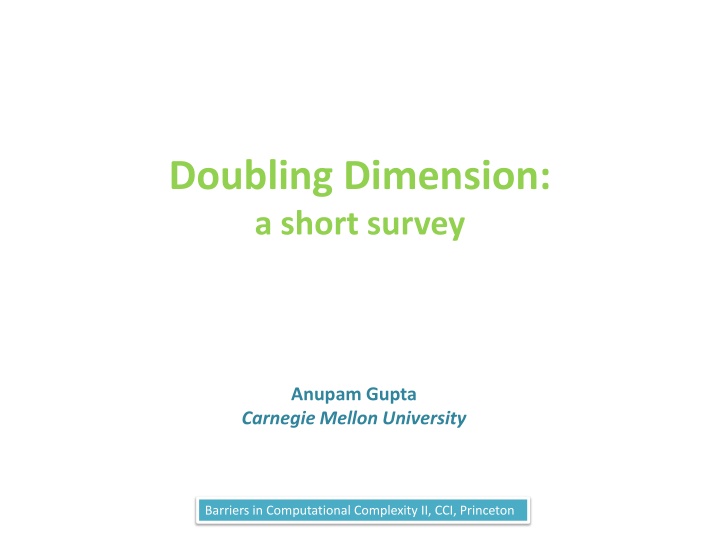
Understanding Doubling Dimension in Metrics
Learn about doubling dimension in metric spaces, how it generalizes geometric dimension, its useful properties, and what types of metrics are not considered doubling. Explore the concept's implications and applications in computational complexity research.
Download Presentation

Please find below an Image/Link to download the presentation.
The content on the website is provided AS IS for your information and personal use only. It may not be sold, licensed, or shared on other websites without obtaining consent from the author. If you encounter any issues during the download, it is possible that the publisher has removed the file from their server.
You are allowed to download the files provided on this website for personal or commercial use, subject to the condition that they are used lawfully. All files are the property of their respective owners.
The content on the website is provided AS IS for your information and personal use only. It may not be sold, licensed, or shared on other websites without obtaining consent from the author.
E N D
Presentation Transcript
Doubling Dimension: a short survey Anupam Gupta Carnegie Mellon University Barriers in Computational Complexity II, CCI, Princeton
Metric space M = (V, d) y z (finite) set V of points symmetric non-negative distances d(x,y) x triangle inequality d(x,y) d(x,z) + d(z,y)
doubling dimension Dimension dimD(M) is the smallest k such that every set S with diameter DS can be covered by 2k sets of diameter DS = 2dim_D = doubling constant D
doubling generalizes geometric dimension Take k-dim Euclidean space Rk Claim: dimD(Rk) (k) Easy to see for boxes 23 boxes to cover larger box in R3 Argument for spheres a bit more involved.
facts about doubling The notion of doubling dimension behaves smoothly under metric distortion definition closed under taking submetrics jargon: doubling = family of metrics with doubling dimension bounded by some absolute constant c independent of n.
useful property of doubling Suppose a metric (X,d) has doubling dimension k. If any subset S X of points has all inter-point distances lying between and then |S| (2 / )k Proof:recursively apply the definition
useful property of doubling Suppose a metric (X,d) has doubling dimension k. If any subset S X of points has all inter-point distances lying between and then |S| (2 / )k this 2-dim set has O( / )2 points
what is not a doubling metric? The equidistant metric Un on n points has dimension (log n) Hence low doubling dimension captures the fact that the metric does not have large (near)-equidistant metrics.
the picture thus far Doubling dimension k Euclidean dimension (k) Metrics with >> 2k nearly-equidistant points
btw, just to check Natural Q: Do all doubling metrics embed into 2 with distortion O(1)? No. The Laakso fractals require ( log n) distortion to embed into 2 with any number of dimensions. [GKL 03] In fact, the right behavior is ( dimD log n) [KLMN 04, ABN 05, JLM 09]
a substantial(?) generalization Doubling dimension k Euclidean dimension (k) Many geometric algorithms can be extended to doubling spaces Small-world networks Traveling Salesman Sparse Spanners Approx. inference Network Design Clustering problems Well-separated pair decomposition Data structures Learnability Near neighbor search Compact routing Distance labeling Network triangulation Sensor placements
example application Assign labels L(x) to each host x in a metric space Looking just at L(x) and L(y), can infer distance d(x,y) Results labels with (O(1)/ )dim log n bits estimates within (1 + ) factor y 010001 010001 Contrast with lower bound of n bit labels in general for any factor < 2 110001 110001 x f( , ) d(x,y)
another example [Arora 95] showed that TSP on Rk was (1+ )-approximable in time [Talwar 04] extended the first result to metrics with doubling dimension k Can we get the PTAS as well?
example in action: sparse spanners for doubling metrics
spanners Given a metric M = (V, d), a graph G = (V, E) is an (m, )-spanner if 1) number of edges in G is m 2) d(x,y) dG(x,y) (1 + ) d(x,y) A reasonable goal: = 0.1, m = O(n) Fact: For the equidistant metric Un, if < 1 then G = Kn
spanners for doubling metrics Theorem: Given any metric M, and any < , we can efficiently find an spanner G with stretch and number of edges m = n (1 + 1/ ) dimD(M) Hence, for doubling metrics, linear-sized spanners! Generalizes a similar theorem for Euclidean metrics.
standard tool: nets Nets: A set of points N is an r-net of a set S if d(u,v) r for any u,v 2 N For every w 2 S \ N, there is a u 2 N with d(u,w) < r r
standard tool: nets Nets: A set of points N is an r-net of a set S if d(u,v) r for any u,v 2 N For every w 2 S \ N, there is a u 2 N with d(u,w) < r Fact: If a metric has doubling dim k and N is an r-net ) B(x,2r) \ N has O(1)k points.
recursive nets 16 8 4 2 Suppose all the points were at least unit distance apart so you take a 2-net N1 of these points Now you can take a 4-net N2 of this net And so on
recursive nets N4 N3 N2 N1 N0 = V Nt is a 2t-net of the set Nt-1 Nt is a 2t+1-net of the set V (almost)
the spanner construction N4 N3 Connect each net point in Nt to other net points at distance at most O(1/ ) 2t N2 N1 N0 = V Nt is a 2t-net of the set Nt-1 Nt is a 2t+1-net of the set V (almost)
the number of edges Number of points in Nt within O(1/ ) 2t of some net point at most O(1/ )k Number of levels = O(log diameter) Number of nodes in net at each level n Hence, number of edges n log diameter O(1/ )k Can be improved to n O(1/ )k
spanners for doubling metrics Theorem: Given any metric M, and any < , we can efficiently find an (m, )-spanner G with number of edges m = n (1 + 1/ ) dimD(M) Hence, for doubling metrics, linear-sized spanners!
example in action: TSP for doubling metrics
plan of attack We have PTASs for TSP for points in constant-dimensional 2. If we could embed doubling metrics into that maintains distances to within (1+ ) (in expectation) constant-dimensional 2 we d be done. completely ridiculous strategy, but maybe we ll get somewhere.
embedding doubling trees into 2 Recall: embedding doubling metrics into 2 requires ( log n) distortion, regardless of dim n. however Theorem: if a doubling metric is also a tree metric, embeds into 2 with distortion O(1) and dimension O(1) poly( ) poly( )
embedding doubling metrics into doubling trees Bad news: 2-d grids require (log n) distortion to embed into distributions over trees Good news: All doubling metrics embed into distributions over doubling trees with distortion O(log n).
plan of attack We have PTASs for TSP for points in constant-dimensional 2. If we could embed doubling metrics into that maintains distances to within (1+ ) (in expectation) constant-dimensional 2 we d be done.
Aroras simpler TSP idea Given any TSP tour of length L in d-dim space find B = (log n/ )d portals in each cluster and show there exists a portal-respecting tour which increases length by L Now dynamic program to find best portal-resp tour runtime ~ (n log n) BB
Aroras simpler TSP idea Given any TSP tour of length L in d-dim space find B = (log n/ )d portals in each cluster and show there exists a portal-respecting tour which increases length by L OPT tour of length L* in original doubling metric embeds into O(1)-dim space with length L = O(log n)L* define portals, choosing = /O(log n) increase in length = L = L* and now find the best portal-respecting tour in original doubling metric!
recap for TSP embedded doubling metric randomly into doubling trees embedded those into constant-dimensional 2 use that to find clusters/portals and claim existence of (1+ ) OPT tour find best tour in original metric using dynamic programming. Talwar s algorithm does it better, dependence on dimD, not on
low dimensional embeddings (and dimensionality reduction)
dimensionality reduction If a Euclidean metric embeds into Rk for some dimension k with distortion O(1) the Euclidean metric has doubling dimension O(k) we want to efficiently find an Euclidean embedding into RO(k) with distortion O(1) We just saw: embed any metric with doubling dimension k into distribution over 2O(k)-dimensional 1 spaces with distortion O(log n)2O(k).
dimensionality reduction If a Euclidean metric embeds into Rk for some dimension k with distortion O(1) the Euclidean metric has doubling dimension O(k) we want to efficiently find an Euclidean embedding into RO(k) with distortion O(1) We just saw: embed any metric with doubling dimension k into distribution over 2O(k)-dimensional 1 spaces with distortion O(log n)2O(k).
dimensionality reduction If a Euclidean metric embeds into Rk for some dimension k with distortion O(1) the Euclidean metric has doubling dimension O(k) we want to efficiently find an Euclidean embedding into RO(k) with distortion O(1) We just saw: embed any metric with doubling dimension k into distribution over 2O(k)-dimensional 1 spaces with distortion O(log n)2O(k). O*(log n) Better: O(k) 2 space
a more general bound Example Theorem: Any metric with doubling dimension dimD embeds into Euclidean space with T dimensions with distortion dimD T log n (where T 2 [ dimD log log n, log n]) All these techniques are ultimately limited by fact that they embed all doubling metrics, and not just Euclidean ones.
special cases of interest Distortion Distortion on using O(log n) Euclidean dimensions on using O(dimD (M)) Euclidean dimensions General metrics Euclidean If the metric is doubling, this quantity is sqrt{log n}. This generalizes result we talked about in Lecture #2: any metric embeds into Euclidean space with O(log n) distortion In general, this is never more than O(log n). This is just the Johnson-Lindenstrauss lemma. Again generalizes the previous result.
weaken requirements? Low-dimensional projection preserving near-neighbors O(log dimD poly -1) dimension random projection [IN05?] (random projections also work for points on smooth manifolds) Give low-dim set of points approximating d(x,y)0.99 Again, can get similar dimensionality [GK10, BRS10]
one more useful tool.. Given a metric M, such that nearby vertices lie in different pieces want to partition it randomly into pieces of small diameter only with small probability. random metric decompositions
padded decompositions A metric (V,d) admits -padded decompositions, if for every , we can output a random partition V = V1] V2] ] Vk 1. each Vjhas diameter 2. Pr[ B(x, ) split ]
the facts Thm: Doubling metrics admit O(dimD)-padded decompositions Useful wherever padded decompositions are useful E.g.: can prove that all doubling metrics embed into 2 with distortion
last slide: some questions For specific metric space problems, can we match the performance for their geometric counterparts? Which problems admit algorithms whose performance can be parameterized using such a notion of dimension? Other notions of dimension that are algorithmically significant?