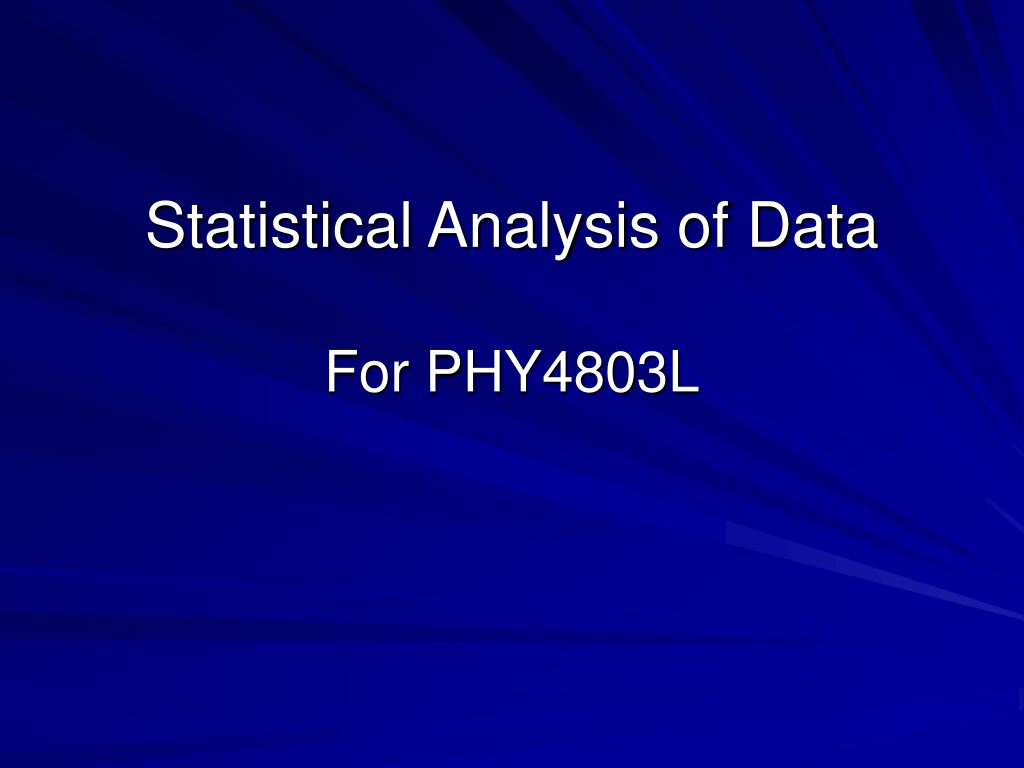
Statistical Analysis of Data in Experimental Physics
Explore the fundamental principles of statistical analysis in experimental physics, covering topics such as measurement, model, random variables, sampling, errors, and regression analysis. Understand the importance of uncertainties, significant figures, and systematic errors in experimental data interpretation. Enhance your knowledge of handling experimental data for accurate results and conclusions.
Download Presentation

Please find below an Image/Link to download the presentation.
The content on the website is provided AS IS for your information and personal use only. It may not be sold, licensed, or shared on other websites without obtaining consent from the author. If you encounter any issues during the download, it is possible that the publisher has removed the file from their server.
You are allowed to download the files provided on this website for personal or commercial use, subject to the condition that they are used lawfully. All files are the property of their respective owners.
The content on the website is provided AS IS for your information and personal use only. It may not be sold, licensed, or shared on other websites without obtaining consent from the author.
E N D
Presentation Transcript
Statistical Analysis of Data For PHY4803L
References Statistical Treatment of Experimental Data Hugh D. Young An Introduction to Error Analysis John R. Taylor Data Reduction and Error Analysis for the Physical Sciences Philip R. Bevington, D. Keith Robinson Statistical Analysis of Data in the Linear Regime Robert DeSerio
Overview of an Experiment Vacuum pump Thermocouple Sample Laser Theory Brownian motion Fermi surface Doppler shift physical model apparatus statistical analysis measurements data Gaussian Chi-square Regression Amplifiers Thermometry Random & systematic errors Communication Experiment
Main Topics Measurement Model Random variables Sampling, expectation values Independence and Correlation Propagation of errors Central limit theorem Principle of Maximum Likelihood Regression analysis Evaluating a fit Excel
Measurements 7.3 0.1 cm y ym units measurement value uncertainty 3.30 0.15 V Uncertainties should have one or two sig. figs. 10% rule. Least significant digit in a measurement (or derived quantity) should match least significant digit in its uncertainty. Include a trailing zero if necessary. Give (your best guess for) 1- uncertainty estimate (rms dev).
Significant figures 180,000 5000 1.80 x 105 500 180,000. 0.5 1.73 0.0376 0.04 1.7365 1.74 0.04 (1.7438 0.0004) x 10-4 1.7438 x 10-4 4 x 10-8
Measurement model Every measurement ym is a sample from some distribution ym = + y is distribution mean yis random error where: and Mean is associated with a theoretical quantity (measurand) ytrue = ytrue + ysys where: ytrueis what you are trying to determine (e.g. thermometer measures sample temperature.) ysys is systematic error. and
Systematic errors ysys is not random; is not from a distribution often depends on y offset (zeroing) errors --- constant ysys for all y scaling errors ---- ysys proportional to y is minimized (not eliminated) by calibrations Systematic errors become more important as y gets small Sometimes the cause of disagreement between theory and measurement Must be considered apart from random error
Discrete random variable Countable outcomes can be described by an integer n Probability distribution given by P( n ) P(n) is the actual probability of obtaining each n. Normalization: Some value of n must occur with a probability of 1 ?(?) = 1 Example: Poisson parameterized by its mean Possible values are integers n from 0 to ?(?) =? ??? all ? ?!
Continuous random variable Defined by probability density function (pdf) p(y) (note lower case p). Probability obtained by integration of pdf ?2 ?(?1< ? < ?2) = ?(?)?? ?1 Probability that y is between y and y+dy ??(?) = ?(?)?? Normalization ? ? ?? = 1 p(y) has units of probability per unit y
Continuous to discrete Size of least significant digit y ?+ ?/2 ?(?) = ?(? )?? ? ?/2 ?(?) ?
The normal or Gaussian distribution 2??2exp (? ?)2 1 ?(?) = ?2 ?2 2??2exp (? ?)2 1 ?(?1< ? < ?2) = ?? ?2 ?1 Parameters: ? = ? Brackets indicate expectation value ?2= (? ?)2 Very important !!! For Poisson: ?2= ?
?(? ??) = ?(? ?? < ? < ? + ??) ?+?? 2??2exp (? ?)2 1 = ?? 2?2 ? ?? ?? 2??2exp ?2 1 = 2?2??; ? = ? ? ?? ? 1 2?exp ? 2 ? =? ? ?? ; = 2 ? ? ?1 2?exp ? 2 ?? = 2 2 0 Z 1 2 3 P( z ) 68% 95% 99.7%
Sample average mean and std dev ?? ? = 1...? ? Sample (sample size N) ?(?) =1 ?(??) ? ?=1 Sample average (definition) Ex.: Sample mean ? ? =1 ? ?=1 ?? Sample variance ? ? 1 2=1 2= (?? ?)2 ?? ? 1 (?? ?)2 ?? ? ?=1 ?=1 ?? ? Sample std. dev. of the mean ? ?=
Law of Large Numbers As N average is not a random number anymore --- it is a well-defined constant. lim ? ?(?) = ?(?) CONSEQUENCE: discrete ?(?) = ?(??)?(??) all ? continuous ?(?) = ?(?)?(?)?? all ?
Central Limit Theorem yi i i = 1 N with N large With each yi from ANY distribution with mean i variance i2 yiwill be Gaussian with mean = i variance = i2
Stats Exercises Set 1 Probability distributions Ex. 1-3 (1 for Gaussian and Poisson distribution only) Ex. 4-7 Set 2 PML, expectation values, and Student-T Set 3 Correlation, POE & Regression Ex. 8-13
POE 2 ?? ?? 2= ??2 ??
Covariance matrix ?11 ?21 ?31 ?12 ?22 ?32 ?13 ?23 ?33 2= ?? variances 2= 2 ?? ?? ?? ? = ? covariances ???= (?? ??)(?? ??) ? ?
POE (multiple input variables) ? = ?(?1,?2,...) First order Taylor expansion ?? ??1 ?? ??2 ? = ??+ ?1 ?1 + ?2 ?2 + 2= (?? ??)2 ?? 2= (? ??)2 ?? Definitions: ???= (?? ??)(?? ??) 2 2 ?? ??1 ?? ??2 Result: 2+ 2+ ??2= ?1 ?2 + 2 ?? ??1 ?? ??2 ?12+
POE (multiple input and output) (also for the previous two cases) ?1= ?1(?1,?2, ) ?2= ?2(?1,?2, ) ?]?? = ??? [ ?? ??? 2] = [ ?? ?][?? 2] [ ?? ?]? [??
Regression model Data set: Prediction: Ex. There is an xi for each yi giving??= ?1 ??+ ?2 Where ?1 and ?2 are true theory parameters. Find estimates ?? of each ?? thus providing estimates ?? ?? Ex: ?? ??,?? ??= ??(?1,?2, ??) ? = 1...? fitof each ??: fit = ??(?1,?2, ??) fit= ?1 ??+ ?2
Regression: principle of maximum likelihood Determine theory parameters that make the data as likely as possible. Then, if the data are too unlikely with these parameters, they will only be less likely with any other values for the parameters. Parameters found this way are called maximum likelihood estimators or best estimates. But they are not the true values, they are still random variables.
Probability of data point ??: fit)2 2 2exp (?? ?? ?? P(??) = 2?? 2??? Probability of entire data set ??: ? fit)2 2 ?? exp (?? ?? P( ??) = 2?? 2 2??? ?=1 Maximize probability by minimizing chi-square: ? fit)2 (?? ?? ?2= 2 ?? ?=1
Regression ? ??2 ??? fit)2 (?? ?? ?2= = 0 2 ?? ?=1 ? fit) fit (?? ?? ?? ??? ??? 0 = 2 ?=1 fit ?]?? = ??? [ ?? ?]?[?? 0 = [ ?? 2] 1? ???? ??? Covariance matrix: ?]?[?? ?] 2] 1= [ ?? 2] 1[ ?? [?? Parameter solution: ? ????=[??2] [ ?? ?]?[?? 2] 1? ????
Parameter uncertainties fit= ?1(?1,?2, ) ?1 fit= ?2(?1,?2, ) ?2 ?]?[?? ?] 2] 1= [ ?? 2] 1[ ?? [?? fit ?]?? = ??? [ ?? ???
Chi-square ? (?? ??)2 ?? ?2= ? ?2= 2 2= 2? ??2 ?=0 ?2= ? ? ? fit)2 (?? ?? ?2= 2 2= 2(? ?) ?? ??2 ?=0
Propagation of Error Direct: ?1= ?1(?1,?2, ) ?2= ?2(?1,?2, ) 2] = [ ?? ?][?? 2] [ ?? ?]? [?? ?]?? = ??? [ ?? ??? fit= ?1(?1,?2, ) ?1 Fit: ?]?[?? ?] 2] 1= [ ?? 2] 1[ ?? [?? fit= ?2(?1,?2, ) ?2 fit ?]?? = ??? [ ?? ???
Go to Excel Regression for Non-linear regression example Be sure to mention: plotting issues hand fitting parameter renormalization delta chi-square = 1 rule data: error bars (no line) theory: line/no symbols
Systematic vs. Random Errors The bulls-eye figure Lower random error -- easier to distinguish small changes in the measurement quantity Higher random error Lower systematic error Higher systematic error
POE (multi variable) ?1= ?1(?1,?2, ) ?2= ?2(?1,?2, ) Direct: 2] = [ ?? ?][?? 2] [ ?? ?]? [?? ?]?? = ??? [ ?? ??? fit= ?1(?1,?2, ) fit= ?2(?1,?2, ) ?1 Fit: ?]?[?? ?] 2] 1= [ ?? 2] 1[ ?? [?? ?2 fit ?]?? = ??? [ ?? ???
?(? ??) = ?(? ?? < ? < ? + ??) ?+?? 2??2exp (? ?)2 1 = ?? 2?2 ? ?? ?? 2??2exp ?2 1 = 2?2??; ? = ? ? ?? ? 1 2?exp ? 2 ? =? ? ?? ; = 2 ? ? ?1 2?exp ? 2 ?? = 2 2 0 Z 1 2 3 P( z ) 68% 95% 99.7%