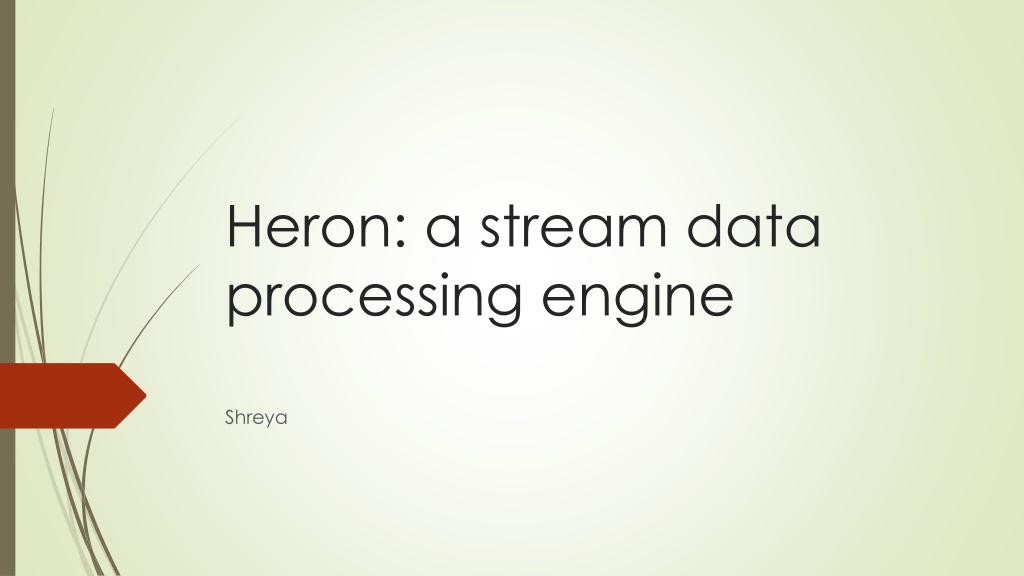
Real-Time Data Processing Engines: Heron and Storm
Heron and Storm are real-time data processing engines widely used for streaming data analytics. Heron provides efficient stream processing with its spouts and bolts architecture, while Storm, once a main platform for real-time analytics at Twitter, faced limitations such as scalability challenges and debugging issues. The complexities of scheduling and resource allocation in Storm led to the need for a more scalable and efficient solution. Despite its capabilities, Storm's architecture posed challenges in debugging and resource management, making it essential to explore newer options for real-time data processing.
Download Presentation

Please find below an Image/Link to download the presentation.
The content on the website is provided AS IS for your information and personal use only. It may not be sold, licensed, or shared on other websites without obtaining consent from the author. Download presentation by click this link. If you encounter any issues during the download, it is possible that the publisher has removed the file from their server.
E N D
Presentation Transcript
Heron: a stream data processing engine Shreya
Real Time Streaming by Storm Storm used to be the main platform for providing real time analytics at Twitter Storm topology is a directed graph of spouts and bolts Spouts: sources of input data/tuples (stream of tweets) Bolts: abstraction to represent computation on the stream. Like real-time active user counts (RTAC) Spout and bolts are run as tasks.
Storm Worker Multiple tasks are grouped into an executor Multiple executors are grouped into a worker Each worker runs as a JVM process. Worker processes are scheduled by the OS Each executor is mapped to two threads scheduled using preemptive priority based by the JVM. Each executor has a scheduler
Limitations of Storm As the scale and diversity of the data increased the Storm s limitations became apparent These many layers of scheduling makes it a much more complex problem and more difficult to predict performance Since each worker can run separate tasks, you could potentially have spouts and bolts from different sources depending on the same resources
Limitations of Storm Makes debugging difficult because when restarting it is possible to find the erroneous task scheduled with different tasks therefor difficult to find. Also if an entire worker process is killed it will hurt other running tasks. The resource allocation methods can lead to overprovisioning. Becomes more complicated as you increase the types of components being put in worker
Limitations of Storm As the number of bolts/ tasks increases in a worker, each worker tends to be connected to other workers. There are not enough ports in each worker to communicate: not scalable. A new engine was needed that could provide better scalability, sharing of cluster resources, and debuggability. Because the multiple components of the topology are bundled into one OS process, debugging is difficult.
Storm Nimbus Limitations Schedules, monitors, and distributes JARs. It also Manages counters for several topologies All these tasks make Nimbus the bottleneck Does not support resource isolation at granular level. Workers in different topologies on the same machine can interfere with each other. Solution: run entire topologies on one machine. -> Leads to waste of resources Uses Zookeeper to manage heartbeats from workers. Becomes the bottleneck for large numbers of topologies When Nimbus fails, users can t modify topologies and failures cannot be automatically detected.
Efficiency Reduced performance were often caused by Suboptimal Replays: tuple failure anywhere leads to failure of the whole tree Long Garbage Collection Cycles: High RAM usage for GC cycles results in high latencies and high tuple failures Queue contention: there is contention for transfer queues, Storm has dealt with this issues by overprovisioning
Heron Runs topologies (directed acyclic graph of spouts and bolts). Programmer specifies number of tasks for each spout and bolt, how data is partitioned across spout. Tuple Processing follows At most once: no tuple proessed more than once At least once: Each tuple is guaranteed to be processed at least once.
Architecture Deploy topologies to Aurora Scheduler using the Heron CL tool Aurora: generic service scheduler. Runs a framework on top of Mesos This scheduler can be replaced by a different one Each topology is an Aurora Job consisting of containers
Architecture The first container is the Toplogy Master The rest are Stream Manager, Metris Manger, and Heron Instances (one JVM) Containers are scheduled by Aurora Processes communicate with each other using protocol buffers
Topology Master Responsible for managing topologies and serves as point of contact for discovering status of the topology A topology can only have one TM Provides an endpoint for toplogy metrics.
Stream Manager Manage routing of tuples efficiently. Heron Instances connect to the local SM to send and receive tuples. There are O(k2) connections where k is the number of containers/SMs Stage by Stage Backpressure: propagate backpressure stage by stage until reaches the spouts High and low water mark Allows traceability by finding what triggered the backpressure
Heron Instance Each Heron Instance is a JVM process. Single threaded approach: maintains TCP comuniation to local SM. Waits for tuples to invoke user logic code. Output is buffered until threshold is met and all is delivered to SM. User can block because of read/writes, calling the sleep system call Two threaded Approach: Gateway thread and task execution thread
Heron Instance Gateway thread: controls communication and data movement in and out. Maintains TCP connections to SM and metrics manager. Task Execution thread: receives tuples and runs user codes. Sends output to gateway thread. GC: can be triggered before gateway sends out tuples => periodically check queue size
Start up Sequence Topology is submitted to Heron The scheduler allocates necessary resources and schedules containers in several machiens TM comes up on first container and makes itself discoverable using Zookeeper. SM on each container conults Zookeeper to discover TM When connections ae all set up, TM runs assignment algorithm to different components. Begins execution
Failure Scenarios Deaths of processes, failure of containers, failure of machines If TM process dies, the container restarts that process and recovers state from Zookeeper. standby TM becomes master TM and restarted master TM becomes standby If SM dies, it rediscoversss TM upon restart. When HI dies, it is restarted and gets copy of plan from SM
Heron Tracker/Heron UI Saves metadata and collects information on topology. Provides an API to create further tools The UI provides a visual representation of the topologies. Can view the acyclic graph See statistics on specific components. Heron Viz automatically creates a dashboard that provides health and resource monitoring.
Evaluation Tested against Word Count toplogy and RTAC toplogy. Considered at least once and at most once semantics Run on machines with 12 cores, 72 GB of RAM, and 500 GB of disk. Assumed no out of memory crashes or repetitive GC cycles.
Word Count Topology Spouts were could produce quickly Storm s convoluted queue structure led to more overhead. Heron demonstrates better throughput and efficiency Similar results for disabled acknowledgments and RTAC topologies
Summary Resource provisioning is abstracted from cluster manager, allowing isolation A single heron instance runs only a spout or bolt allowing better debugging Step by step backpressure makes clear which component is failing Heron is efficient because there is component level resource allocation A TM allows each topology to be managed independently and failures of one to not interfere with another