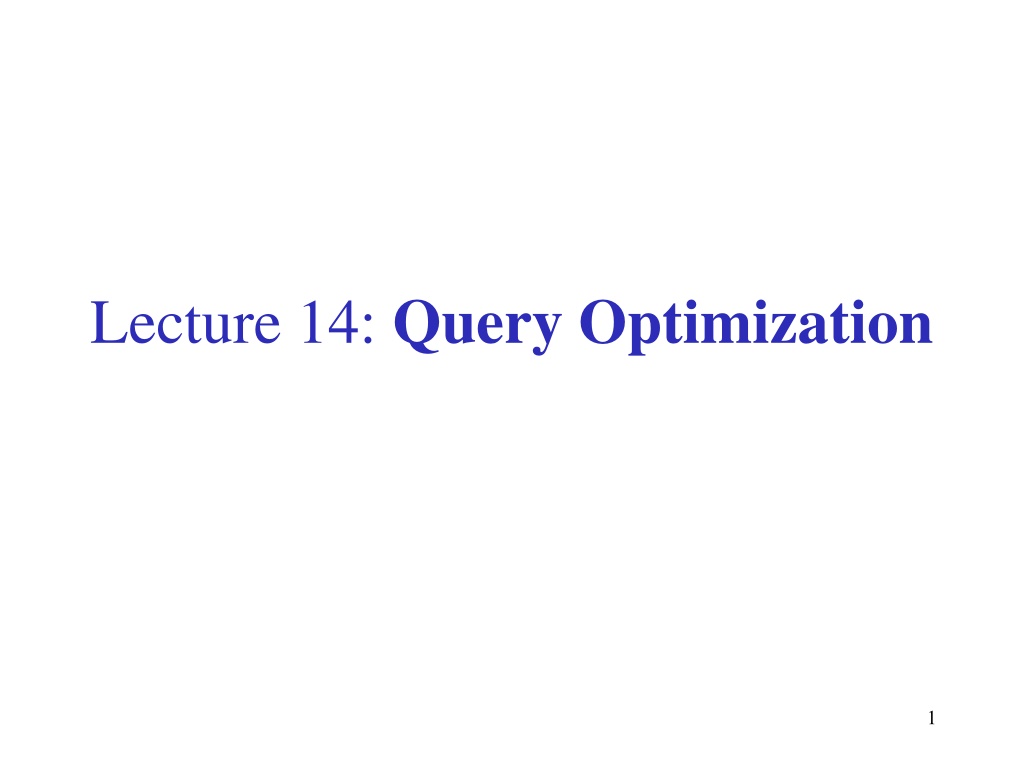
Query Optimization and Algebraic Laws Overview
Explore the process of query optimization and the application of algebraic laws in database management. Understand query rewriting, cost estimation, and the algebraic laws governing database operations. Learn about scan operations, joins, and laws involving selections and projections. Dive into examples to enhance your understanding of optimizing queries effectively.
Download Presentation

Please find below an Image/Link to download the presentation.
The content on the website is provided AS IS for your information and personal use only. It may not be sold, licensed, or shared on other websites without obtaining consent from the author. If you encounter any issues during the download, it is possible that the publisher has removed the file from their server.
You are allowed to download the files provided on this website for personal or commercial use, subject to the condition that they are used lawfully. All files are the property of their respective owners.
The content on the website is provided AS IS for your information and personal use only. It may not be sold, licensed, or shared on other websites without obtaining consent from the author.
E N D
Presentation Transcript
This Lecture Query rewriting Cost estimation We have learned how atomic operations are implemented and their cost We ll complete the picture by estimating the cost query plans Including computing the size of the output (reduction factors) Join ordering - optimization 2
Query Optimization Process (simplified a bit) Parse the SQL query into a logical tree: identify distinct blocks (corresponding to nested sub- queries or views). Query rewrite phase: SQL-to-SQL transforms apply algebraic transformations to yield a cheaper plan. Merge blocks and move predicates between blocks. Optimize each block: join ordering. Complete the optimization: select scheduling (pipelining strategy). 3
Operations (revisited) Scan ([index], table, predicate): Either index scan or table scan. Try to push down sargable predicates. Selection (filter) Projection (always need to go to the data?) Joins: nested loop (indexed), sort-merge, hash, outer join. Grouping and aggregation (usually the last). 4
Algebraic Laws Commutative and Associative Laws R U S = S U R, R U (S U T) = (R U S) U T R S = S R, R (S T) = (R S) T R S = S R, R (S T) = (R S) T Distributive Laws R (S U T) = (R S) U (R T) 5
Algebraic Laws Laws involving selection: C AND C (R) = C( C (R)) = C(R) C (R) C OR C (R) = C(R) U C (R) C (R S) = C (R) S When C involves only attributes of R C (R S) = C (R) S C (R U S) = C (R) U C (S) C (R S) = C (R) S 6
Algebraic Laws Example: R(A, B, C, D), S(E, F, G) F=3 (R S) = ? A=5 AND G=9 (R S) = ? D=E D=E 7
Algebraic Laws Laws involving projections M(R S) = N( P(R) Q(S)) Where N, P, Q are appropriate subsets of attributes of M M( N(R)) = M N (R) Example: R(A,B,C,D), S(E, F, G) A,B,G(R S) = ? ( ?(R) ?(S)) D=E D=E 8
Query Rewrites: Sub-queries SELECT Emp.Name FROM Emp WHERE Emp.Age < 30 AND Emp.Dept# IN (SELECT Dept.Dept# FROM WHERE Dept.Loc = Seattle AND Dept Emp.Emp#=Dept.Mgr) 9
The Un-Nested Query SELECT Emp.Name FROM Emp, Dept WHERE Emp.Age < 30 AND Emp.Dept#=Dept.Dept# AND Dept.Loc = Seattle AND Emp.Emp#=Dept.Mgr 10
Converting Nested Queries Select distinct x.name, x.maker From product x Where x.color= blue AND x.price >= ALL (Select y.price From product y Where x.maker = y.maker AND y.color= blue ) 11
Converting Nested Queries Let s compute the complement first: Select distinct x.name, x.maker From product x Where x.color= blue AND x.price < SOME (Select y.price From product y Where x.maker = y.maker AND y.color= blue ) 12
Converting Nested Queries This one becomes a SFW query: Select distinct x.name, x.maker From product x, product y Where x.color= blue AND x.maker = y.maker AND y.color= blue AND x.price < y.price This returns exactly the products we DON T want, so 13
Converting Nested Queries (Select x.name, x.maker From product x Where x.color = blue ) EXCEPT (Select x.name, x.maker From product x, product y Where x.color= blue AND x.maker = y.maker AND y.color= blue AND x.price < y.price) 14
Rewrites: Group By and Join Schema: Product (pid, unitprice, ) Sales(tid, date, store, pid, units) Trees: groupBy(pid) Sum(units) Join groupBy(pid) Sum(units) Join Products Filter (price>100) Products Filter (price>100) Scan(Sales) Filter(date in Q2,2013) Scan(Sales) Filter(date in Q2,2013) 15
Query Rewrite Summary The optimizer can use any semantically correct rule to transform one query to another. Rules are used for: moving constraints between blocks (because each will be optimized separately) Un-nesting blocks In a few minutes of thought, you ll come up with your own rewrite. Some query, somewhere, will benefit from it. Theorems? 19
Cost Estimation For each plan considered, must estimate cost: Must estimate cost of each operation in plan tree. Depends on input cardinalities. Must estimate size of result for each operation in tree! Use information about the input relations. For selections and joins, assume independence of predicates. We ll discuss the System R cost estimation approach. Very inexact, but works ok in practice. More sophisticated techniques known now. 20
Statistics and Catalogs Need information about the relations and indexes involved. Catalogstypically contain at least: # tuples (T) and # pages (B) for each relation. # distinct key values (K) and #pages for each index. Index height, low/high key values (Low/High) for each tree index. Alternatively V(R, F) #distinct values in field F of relation R Catalogs updated periodically. Updating whenever data changes is too expensive; lots of approximation anyway, so slight inconsistency ok. More detailed information (e.g., histograms of the values in some field) are sometimes stored. 21
Size Estimation and Reduction Factors SELECT attribute list FROM relation list WHERE term1AND ... AND termk Consider a query block: Maximum # tuples in result is the product of the cardinalities of relations in the FROM clause. Reduction factor (RF) associated with each term reflects the impact of the term in reducing result size. Result cardinality = Max # tuples product of all RF s. Implicit assumption that terms are independent! Term col=value has RF 1/K(I), given index I on col Term col1=col2 has RF 1/MAX(K(I1), K(I2)) Term col>value has RF (High(I)-value)/(High(I)-Low(I)) 22
Histograms Key to obtaining good cost and size estimates. Come in several flavors: Equi-depth Equi-width Compressed histograms: special treatment of frequent values. 23
Histograms Statistics on data maintained by the RDBMS Makes size estimation much more accurate (hence, cost estimations are more accurate) V(R,F) number of distinct values in field F of relation R 24
Histograms Employee(ssn, name, salary, phone) Maintain a histogram on salary: Salary: 0..20k 20k..40k 40k..60k 60k..80k 80k..100k > 100k Tuples 200 800 5000 12000 6500 500 T(Employee) = 25000, but now we know the distribution 25
Histograms Ranks(rankName, salary) Estimate the size of Employee Ranks Salary Employee 0..20k 20k..40k 40k..60k 60k..80k 80k..100k > 100k 200 800 5000 12000 6500 500 Ranks 0..20k 20k..40k 40k..60k 60k..80k 80k..100k > 100k 8 20 40 80 100 2 26
Histograms Assume: V(Employee, Salary) = 200 V(Ranks, Salary) = 250 Then T(Employee Ranks) = = ( i=1,6 TiT i)/ 250 = (200x8 + 800x20 + 5000x40 + 12000x80 + 6500x100 + 500x2)/250 = . Salary 27
Schema for Some Examples Sailors (sid: integer, sname: string, rating: integer, age: real) Reserves (sid: integer, bid: integer, day: date, rname: string) Reserves: Each tuple is 40 bytes long, 100 tuples per page, 1000 pages Sailors: Each tuple is 50 bytes long, 80 tuples per page, 500 pages 28
Pipelining Assume that we want to find all the boats ever reserved by sailors with rank 8. SELECT R.bid FROM Sailors S, Reserves R WHERE S.rating=8 AND S.sid=R.sid Consider a plan that performs selection, hash join, and projection in this order Writing the result of each operation to the disk is redundant! In the best case, there is enough memory to perform all the operations simultaneously in memory 29
Pipelining Hash join step 1 for Sailors Hash join step 1 for Reserves Selection Hash join step 2 Projection 1 1 2 2 h2 rating=8 bid h h N N Sailors Output Reserves 30
Pipelining We ignored output cost for single operations For operations that get their input in a pipeline, we reduce the first read cost E.g., selection is for free! In the previous example we saved the full read in the first stage of hash join for sailor, and the full read for the projection Requires sufficient memory To perform succeeding operations in parallel We will usually assume that this is the case 31
Plan Optimimization Task: create a query execution plan Key idea: perform an estimation of the possible query plan costs and choose the cheapest one The query plan operations are carried out in some order, where the results of one operation are pipelined 32
SELECT S.sid FROM Sailors S WHERE S.rating=8 Example If we have an Index on rating: (1/K(I)) T(R) = (1/10) 40000 tuples retrieved. Clustered index: (1/K(I)) (B(I)+B(R)) = search cost + (1/10) (500) pages are read (= 51.2-54). Unclustered index: (1/K(I)) (B(I)+T(R)) = search cost + (1/10) (50+40000) pages are read. Doing a file scan: we retrieve all the pages (500). 33
Determining Join Ordering R1 R2 . Rn Join tree: R3 R1 R2 R4 A join tree represents a plan. An optimizer needs to inspect many (all ?) join trees 34
Types of Join Trees Left deep: R4 R2 R5 R3 R1 35
Types of Join Trees Bushy: R3 R2 R4 R5 R1 36
Types of Join Trees Right deep: R3 R1 R5 R2 R4 37
Problem Given: a query R1 R2 Rn Assume we have a function cost() that gives us the cost of every join tree Find the best join tree for the query 38
Dynamic Programming Idea: for each subset of {R1, , Rn}, compute the best plan for that subset In increasing order of set cardinality: Step 1: for {R1}, {R2}, , {Rn} Step 2: for {R1,R2}, {R1,R3}, , {Rn-1, Rn} Step n: for {R1, , Rn} A subset of {R1, , Rn} is also called a subquery 39
Dynamic Programming For each subquery Q {R1, , Rn} compute the following: Size(Q) A best plan for Q: Plan(Q) The cost of that plan: Cost(Q) 40
Dynamic Programming Step 1: For each {Ri} do: Size({Ri}) = B(Ri) Plan({Ri}) = Ri Cost({Ri}) = (cost of scanning Ri) 41
Dynamic Programming Step i: For each Q {R1, , Rn} of cardinality i do: Compute Size(Q) For every pair of subqueries Q , Q s.t. Q = Q U Q compute cost(Plan(Q ) Plan(Q )) Cost(Q) = the smallest such cost Plan(Q) = the corresponding plan 42
Dynamic Programming Return Plan({R1, , Rn}) 43
Dynamic Programming Summary: computes optimal plans for subqueries: Step 1: {R1}, {R2}, , {Rn} Step 2: {R1, R2}, {R1, R3}, , {Rn-1, Rn} Step n: {R1, , Rn} We used na ve size/cost estimations In practice: more realistic size/cost estimations (next) heuristics for Reducing the Search Space Restrict to left linear trees Restrict to trees without cartesian product need more than just one plan for each subquery: interesting orders 44
The Course in Perspective The relational data model, SQL Views, updates, transactions Conceptual design Expressing the constraints on the domain Using them to get good schema designs Building a database system: Storage and indexing Query execution (join algorithms) Query optimization 45