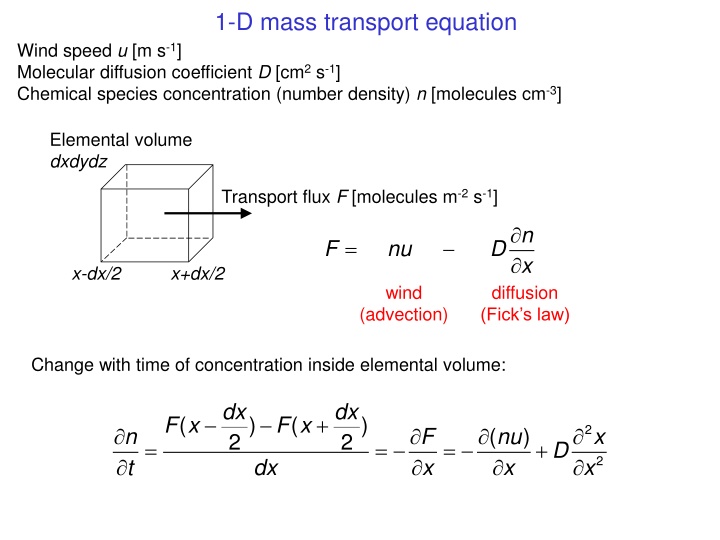
Mass Transport Equations in Atmospheric Sciences
The equations for mass transport in atmospheric sciences are crucial in understanding the dynamics of chemical species and their movement through different media. The interplay between advection, diffusion, and various forms of the advection equation shapes our comprehension of atmospheric processes. By incorporating source and sink terms, these equations depict the complex interrelations between wind speed, molecular diffusion, chemical concentration, and transport flux. Furthermore, the distinction between Eulerian and Lagrangian forms provides insights into how different formulations can be utilized based on the context of the study.
Download Presentation

Please find below an Image/Link to download the presentation.
The content on the website is provided AS IS for your information and personal use only. It may not be sold, licensed, or shared on other websites without obtaining consent from the author. If you encounter any issues during the download, it is possible that the publisher has removed the file from their server.
You are allowed to download the files provided on this website for personal or commercial use, subject to the condition that they are used lawfully. All files are the property of their respective owners.
The content on the website is provided AS IS for your information and personal use only. It may not be sold, licensed, or shared on other websites without obtaining consent from the author.
E N D
Presentation Transcript
1-D mass transport equation Wind speed u [m s-1] Molecular diffusion coefficient D [cm2 s-1] Chemical species concentration (number density) n [molecules cm-3] Elemental volume dxdydz Transport flux F [molecules m-2 s-1] n x = F nu D x-dx/2 x+dx/2 wind diffusion (Fick s law) (advection) Change with time of concentration inside elemental volume: dx dx + F x ( ) F x ( ) 2 n t F x ( nu x ) x 2 2 = = = + D 2 dx x
Generalize mass transport equation to 3-D Fy Wind vector U = (u, v, w)T Flux vector F = (Fx, Fy, Fz)T Fz F = D n n F U Divergence operator Fx = )T ( / , / , / x y z Change of concentration with time: F y F x F z n t y = = = + D 2 ( n ) n F U x z x y 2 2 2 = + + 2 Laplacian 2 2 2 z
Advection vs. diffusion in driving atmospheric transport Time t needed for transport over distance L: Advection: t = L/U where U is wind speed Diffusion: t = L2/2D >>1: transport dominated by advection << 1: transport dominated by diffusion Peclet number: Pe = LU/D Typical values: L ~ 1 km U ~ 10 m s-1 D ~ 1/p ~ 0.1 cm2 s-1 at sea level Pe ~ 109 Diffusion is negligible
Different forms of the advection equation Eulerian flux form: n t = ( n ) U n is number density [molecules cm-3] Eulerian advective form: C t = C U C = n/nais mixing ratio [mol mol-1] where nais number density of air Compressibility of air ( 0) U affects number density, not mixing ratio Lagrangian form: dC dt d dt = 0 = + U where is the absolute derivative (for elemental volume moving with the flow) t Brasseur and Jacob, chap. 4.2.1
Adding source and sink terms: the continuity equation elemental volume transport chemistry Flux Fi = niU X Y Pi, Li deposition emission Continuity equations for number densities n = (n1, nK) of ensemble of K species: t local trend in number density (flux divergence) System of K coupled 4-D partial differential equations n Eulerian flux form + n ( ) i P ( ) L ) U n n i = ( i i emissions, deposition, chemical and aerosol processes transport Eulerian advective form: C t = U + C P L ( ) C ( ) C i i i i Lagrangian form dC dt = P L ( ) C ( ) C i i i Brasseur and Jacob, chap. 4.2.1
Break down dimensionality of continuity equation by operator splitting Solve for transport (x, y, z) and chemistry separately over finite time steps t C t = U + C P L ( ) C ( ) C i i i i Chemistry (local processes): Advection, further split in 1-D: = t C C x (Eulerian) u i i dC dt = P L ( ) C ( ) C i i i = (Lagrangian) Cy dx u t dt ( , ) x Cx C(t+ t) Cz C(t) t t t t Chemical equations: K-dimensional ODE system Advection equations: no chemical coupling Operator splitting induces error by ignoring couplings between transport in different directions and chemistry over t can quantify error by trying t /2, t/4, etc. Brasseur and Jacob, chap. 4.14
On-line and off-line approaches to chemical modeling On-line: coupled to dynamics Off-line: decoupled from dynamics GCM conservation equations: air mass: a / t = momentum: U/ t = heat: / t = water: q/ t = chemicals: Ci/ t = GCM conservation equations: air mass: a / t = momentum: U/ t = heat: / t = water: q/ t = meteorological archive (averaging time ~ hours) PROs of off-line vs on-line approach: computational cost simplicity stability (no chaos) compute sensitivities back in time CONs: no fast chemical-dynamics coupling need for meteorological archive transport errors Chemical transport model: Ci / t = Chemical data assimilation, forecasts best done on-line Chemical sensitivity studies may best be done off-line Brasseur and Jacob, chap. 1.7
Finite differencing in space: Eulerian models partition atmospheric domain into gridboxes This discretizes the continuity equation in space Solve continuity equation for individual gridboxes, like in a box model Present computational limit ~ 108 gridboxes In global models, this implies a grid resolution x of ~ 10-100 km in horizontal and 0.1-1 km in vertical Finite differencing in time and space are coupled by the Courant number condition = u t / x 1; in global models, t ~102-103 s The convenient orthogonal latitude-longitude grid suffers from Courant number singularity at poles Brasseur and Jacob, chap. 4.8
Eulerian models often use equal-area or zoomed grids Equal-area grids: avoid singularities at poles icosahedral triangular cubed-sphere Zoomed grids: increase resolution where you need it (or when, in an adaptive grid) nested stretched Brasseur and Jacob, chap. 4.8
Regional models: limited domain, boundary conditions at edges WRF domain with successive nests 2-way nesting 1-way nesting
Lagrangian models track points in model domain (no grid) Transport large number of points with trajectories from input meteorological data base (U) over time steps t Points have mixing ratio or mass but no volume Determine local concentrations in a given volume by the statistics of points within that volume or by interpolation PROS over Eulerian models: stable for any wind speed no error from spatial averaging easy to parallelize easily track air parcel histories efficient for receptor-oriented problems CONS: need very large # points for statistics inhomogeneous representation of domain individual trajectories do not mix cannot do nonlinear chemistry or aerosol physics cannot be conducted on-line with meteorology position to+ t U t position to Brasseur and Jacob, chap. 4.11
Lagrangian receptor-oriented modeling Run Lagrangian model backward from receptor location, with points released at receptor location only flow backward in time Efficient quantification of source influence distribution on receptor ( footprint )
Representing non-linear chemistry Consider two chemicals A and B emitted in different locations, and reacting by A + B products Eulerian model Lagrangian model gridboxes A B A B A and B never react A and B react following the mixing of gridboxes