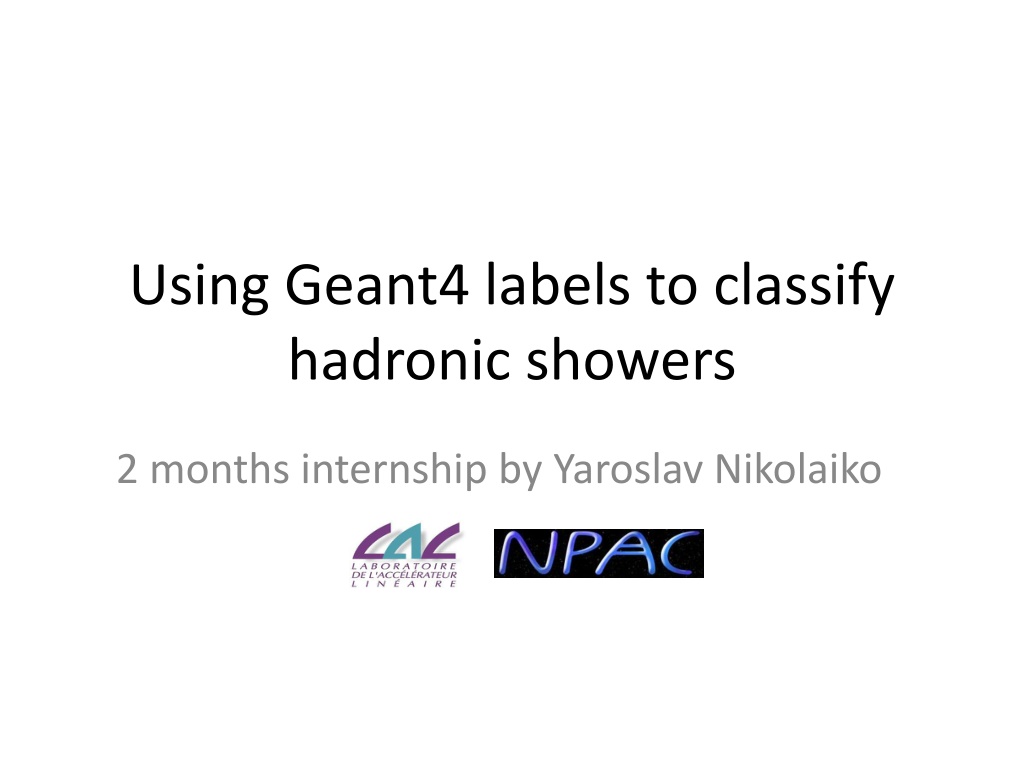
Exploring Hadronic Showers Using Geant4 Labels
In a 2-month internship by Yaroslav Nikolaiko, machine learning techniques were employed to classify hadronic showers in the ECAL using Geant4 labels. The study investigated physics labels and observables, including data set analysis, Geant4 setup, observable parameters, and supervised learning for interaction classification. The collaboration focused on deep learning with input features to continue the research.
Download Presentation

Please find below an Image/Link to download the presentation.
The content on the website is provided AS IS for your information and personal use only. It may not be sold, licensed, or shared on other websites without obtaining consent from the author. If you encounter any issues during the download, it is possible that the publisher has removed the file from their server.
You are allowed to download the files provided on this website for personal or commercial use, subject to the condition that they are used lawfully. All files are the property of their respective owners.
The content on the website is provided AS IS for your information and personal use only. It may not be sold, licensed, or shared on other websites without obtaining consent from the author.
E N D
Presentation Transcript
Using Geant4 labels to classify hadronic showers 2 months internship by Yaroslav Nikolaiko
Introduction In the context of using machine learning techniques to characterize hadronic interactions in the ECAL, investigate the information available in Geant4 and supply useful physics labels and observables 9/9/2013 CALICE Collaboration meeting 2
Data set Testbeam Monte Carlo pi- at 10 GeV, QGSP_BERT 9/9/2013 CALICE Collaboration meeting 3
Geant4 setup and observables Pair Ionization (MIP) Elastic Inelastic Production Number of secondaries Hadronic Model # of secondaries with E>1GeV G4LEPionMinu sInelastic Bertini Cascade Gamma and Nucleus presence Evaporation and gamma de- exitation Pre- Nucleus explosion fission equilibrium 9/9/2013 CALICE Collaboration meeting 4
Results with Multiboost Supervised learning setup Simple single stump learner Input features: combination of energy per layer, energy difference, radius per layer etc. Input labels: MIP, elastic, pair production and inelastic 9/9/2013 CALICE Collaboration meeting 5
Results with Multiboost Similar test with different labels: model, gamma and nucleus presence, number of secondaries, number of high energy secondaries Number of high energy secondaries Number of secondaries Gamma and Nucleus presence Bertini vs Parameterized model 9/9/2013 CALICE Collaboration meeting 6
Summary The architecture of the Geant4 kernel was explored and the basic labels extracted. Supervised learning setup was tested for hadronic interaction classification and for details of inelastic interactions. Collaboration with AppStat group on deep learning (provide input features) To be continued 9/9/2013 CALICE Collaboration meeting 7