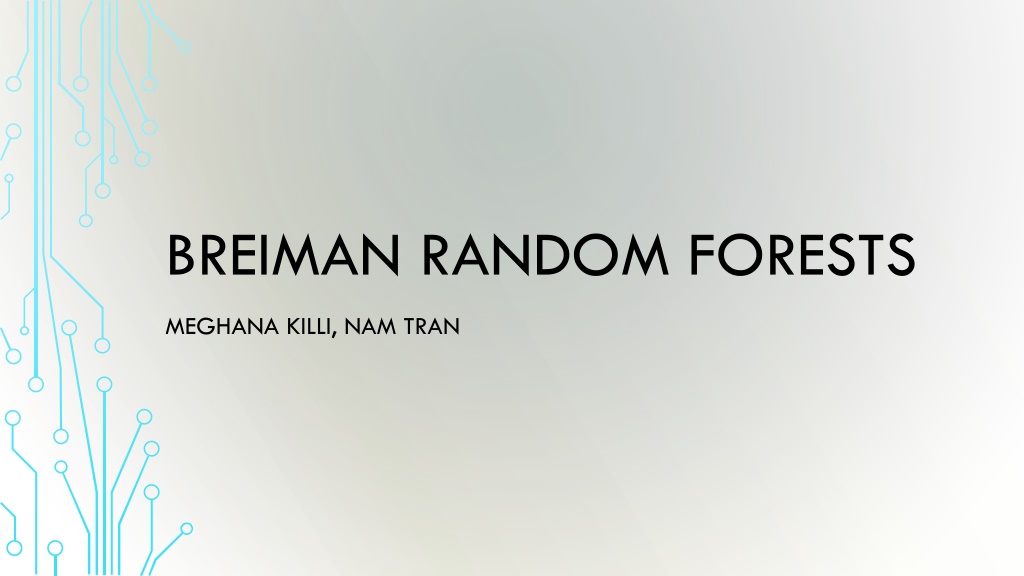
Breiman Random Forests Overview
"Explore the principles and techniques behind Breiman Random Forests, including bootstrapping, bagging, and the random subspace method. Learn how BRFs offer robust classification and regression while avoiding overfitting, making them faster than other methods like Adaboost. Dive into decision trees, random subspaces, and the strengths of BRFs in handling outliers and noise."
Download Presentation

Please find below an Image/Link to download the presentation.
The content on the website is provided AS IS for your information and personal use only. It may not be sold, licensed, or shared on other websites without obtaining consent from the author. If you encounter any issues during the download, it is possible that the publisher has removed the file from their server.
You are allowed to download the files provided on this website for personal or commercial use, subject to the condition that they are used lawfully. All files are the property of their respective owners.
The content on the website is provided AS IS for your information and personal use only. It may not be sold, licensed, or shared on other websites without obtaining consent from the author.
E N D
Presentation Transcript
BREIMAN RANDOM FORESTS MEGHANA KILLI, NAM TRAN
Introduction Machine Learning Deterministic ~ Random Strong Law of Large Numbers Eg: AdaBoost Eg: Random Forests 1 2 3 2
Decision Trees 3 Image source: Elements of Statistical Learning by Hastie et.al.
Random Forests Bootstrapping - Random Sampling Technique with Replacement Random Forests Bagging (B)ootstrap (Agg)regat(ing) {1 2 3 4 5 6} {1 1 2 2 3 3}, {1 1 1 2 2 2}, {1 2 3 4 5 5}, {5 5 6 6 6 6} Decision Trees 4
Random Subspace Method Total number of features = N Generate a subspace at random with m (<N) features Select features from subspace to best split data 5
Decision Trees in a Subspace 6 Image source: Elements of Statistical Learning by Hastie et.al.
Breiman Random Forests Bootstrapping - Random Sampling Technique with Replacement Breiman Random Forests Random Forests Random Subspace Method Bagging (B)ootstrap (Agg)regat(ing) Decision Trees 7
Features of BRFs Classification and Regression Avoid overfitting Insensitive to number of features sampled Robust to outliers and noise - more than Adaboost Faster than bagging and boosting 8