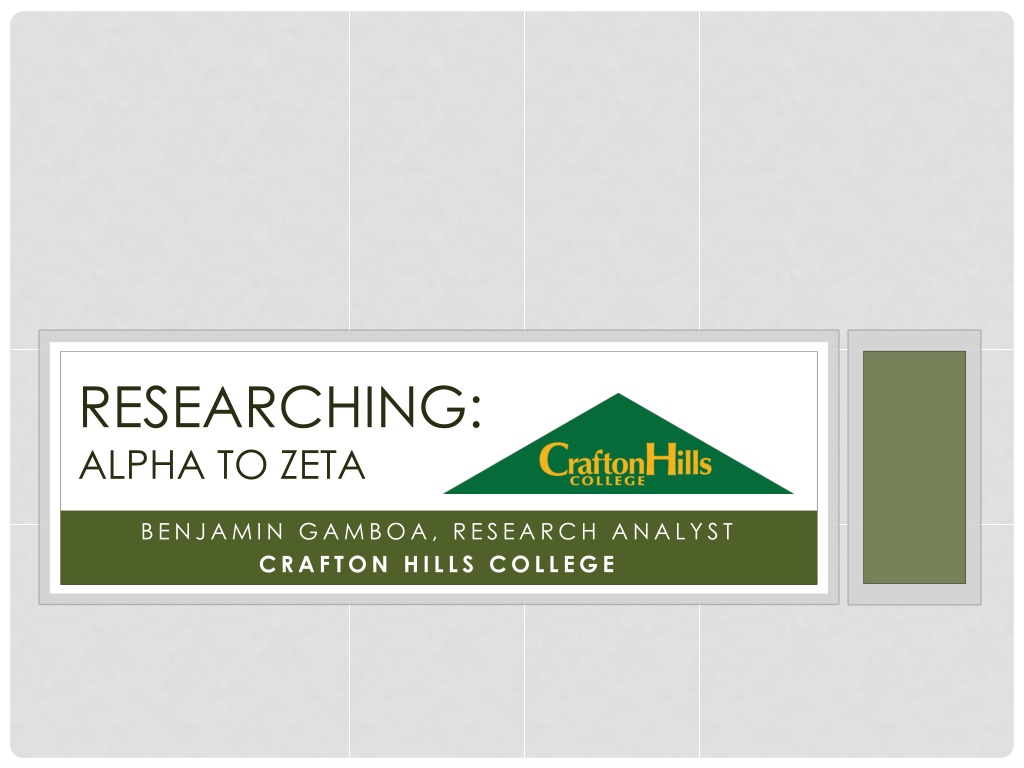
Researching Statistical Techniques for Effective Data Analysis
Explore the journey from creating a dataset to applying statistical techniques like logistic regression and segmentation analytics for impactful research findings. Understand the significance of controls, variables, and practical implications in evaluating research outcomes.
Download Presentation

Please find below an Image/Link to download the presentation.
The content on the website is provided AS IS for your information and personal use only. It may not be sold, licensed, or shared on other websites without obtaining consent from the author. Download presentation by click this link. If you encounter any issues during the download, it is possible that the publisher has removed the file from their server.
E N D
Presentation Transcript
RESEARCHING: ALPHA TO ZETA BENJAMI N GA MBOA, RESEARCH ANALYST CRAFTON HILLS COLLEGE
SESSION OBJECTIVES Participants will be able to: Apply proper controls and create a dataset for a research study Evaluate multiple statistical analyses, such as statistical and practical significance, logistic regression, and segmentation modeling, for appropriateness to the study Facilitate conversations around findings to effect change at their college
MOTIVATION Communications professor observed 100% success rates in short-term courses Considerable literature on accelerated and compressed coursework Title III STEM Grant was considering compressed sequencing
CONTROLS Reviewed literature for methods Determined 8-week control would provide most data and usable results Other controls: college, course, course length, faculty, course type, and term (fall and spring only) Disaggregation: age, gender, and ethnicity
CREATING DATASET A total of 4,592 records (over 5 years) were identified for the treatment group Applying the controls, 11,002 records were identified for the control group Variables created: Course success, cumulative prior GPA (continuous), cumulative prior GPA (normalized), student s age in term, first-time students
STATISTICAL TECHNIQUES Statistical significance: p-value Practical significance: effect size (Cohen s d) Predictive analytics: logistic regression & segmentation analytics
STATISTICAL TECHNIQUES Statistical significance: p-value Practical significance: effect size (Cohen s d) Predictive analytics: logistic regression & segmentation analytics
STATISTICAL SIGNIFICANCE P-value is a well known statistic that faculty and decision-makers understand (or at least think they do) Remember, the p-value is impacted by the sample size! ?1 ?2 ? = 2 2 ?1 ?1+?2 ?2 Use responsibly
STATISTICAL TECHNIQUES Statistical significance: p-value Practical significance: effect size (Cohen s d) Predictive analytics: logistic regression & segmentation analytics
PRACTICAL SIGNIFICANCE Effect size (Cohen s d): difference of the two means divided by the pooled standard deviation ?1 ?2 ? = 2+ ?2 1 ?2 ?1+ ?2 2 2 ?1 1 ?1 Using Cohen as a guide: Small effect 0.20 Medium effect 0.50 Large effect 0.80
STATISTICAL TECHNIQUES Statistical significance: p-value Practical significance: effect size (Cohen s d) Predictive analytics: logistic regression & segmentation modeling
PREDICTIVE ANALYTICS Logistic Regression Predicts binary outcome (i.e. success, no success) Standard package in statistical software Supports (A LOT) of continuous and dichotomous predictor variables Assumes lack of relationship between predictor variables
PREDICTIVE ANALYTICS Logistic Regression Dummy coding Multicollinearity Missing values Overfitting the model Selecting the cut off value Choosing the best model Goodness-of-fit test Interpretation of results
PREDICTIVE ANALYTICS Segmentation Modeling Classification tree (CRT) algorithm Standard in some packages, add-on for others Partitions cases along predictor variables where similar outcomes are grouped together Visual output that is easy to describe and understand
RESULTS Success Rates by Course Length Traditional courses 69% Compressed courses 75% 0% 25% 50% 75% 100%
RESULTS Success Rates by Course Length for Students with Higher and Lower than Average Prior GPAs 57% Lower GPA Traditional courses 77% Condensed courses 70% Higher GPA 85% 0% 25% 50% 75% 100%
RESULTS Success Rates by Course Length and Subject 71% English Traditional courses 88% Compressed courses 85% Reading 92% 0% 25% 50% 75% 100%
RESULTS Course length has an odds ratio of 1.553 and a positive coefficient meaning a student enrolled in a compressed course is one and a half times more likely to succeed than a student enrolled in a traditional-length course. Prior cumulative GPA has an odds ratio of 2.083 and a positive coefficient meaning as a student is two times more likely to succeed than a student with a GPA .73 points lower. Predictor p Exp( ) Course Length .44 <.001 1.553 Prior GPA .73 <.001 2.083
RESULTS Segmentation model best predicted success for: 1) continuing students with GPAs greater than 3.028 and 2) students with GPAs between 2.457 and 3.028 enrolled in condensed courses 1 2
CLOSING THE RESEARCH LOOP Publish and distribute a full report and dashboard
CLOSING THE RESEARCH LOOP Presentation to faculty chairs committee Left the statistical language out Focused on: Correlated relationships Variables that best predicted course success Implications on teaching and learning
CLOSING THE RESEARCH LOOP Fall 2014 Spring 2015 Fall 2015 Spring 2016 Transferable Math Math 090 Math 095 Math 095 Math 942 Math 952 Math 090 Math 090 Math 095 Transferable Math Transferable Math Math 942 Math 952 Math 090 Math 095
CLOSING THE RESEARCH LOOP Provided impetus to grant program to identify interested faculty for a pilot program Completed first term of Fast Track Math Non-Fast Track MATH-942 Fast Track MATH-942 Effect Size (d) Outcome P-value # N % # N % Success in MATH-942 22 50 44.0 41 61 67.2 0.47 0.014 Retain to MATH-952 7 22 31.2 41 41 100.0 1.59 <0.001 Success in MATH-952 5 7 71.4 24 41 58.5 -0.26 0.523 Success in Sequence 5 50 10.0 24 61 39.3 0.66 <0.001
RESOURCES DesJardins, S.L. (2001). A comment on interpreting odds-ratios when logistic regression coefficients are negative. The Association for Institutional Research, 81, 1-10. Retrieved October, 15, 2006 from http://airweb3.org/airpubs/81.pdf George, D., & Mallery, P. (2006). SPSS for windows step by step: A simple guide and reference (6th ed.). Boston: Allyn and Bacon. Harrell, F.E. (2001). Regression Modeling Strategies: With Applications to Linear Models, Logistic Regression, and Survival Analysis. New York: Springer Science+Business Media, Inc. RP Group (2013). Suggestions for California Community College Institutional Researchers Conducting Prerequisite Research. Retrieved January 29, 2014 from http://www.rpgroup.org/sites/default/files/RPGroupPreqreqGuidelinesFNL.pdf Tabachnick, B.G. & Fidell, L.S. (2007). Using Multivariate Statistics (5th ed.). Boston: Pearson Education. Wetstein, M. (2009, April). Multivariate Models of Success. PowerPoint presentation at the RP/CISOA Conference, Tahoe City, CA. Retrieved January 28, 2014 from http://www.rpgroup.org/sites/default/files/Multivariate%20Models%20of%20Succe ss.pdf Wurtz, K. A. (2008). A methodology for generating placement rules that utilizes logistic regression. Journal of Applied Research in the Community College, 16, 52-58.