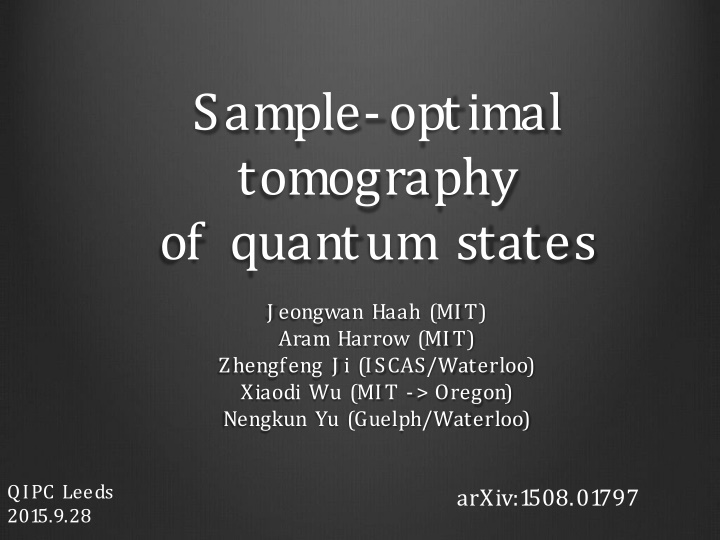
Quantum State Tomography: Optimal Methods and Bounds
Explore sample-optimal tomography of quantum states, minimizing loss and maximizing classical descriptions. Learn about the necessary/sufficient number of copies, boundary cases, and local asymptotic normality. Dive into optimal measurements, symmetry determinations, related work, and lower bounds in quantum state estimation.
Download Presentation

Please find below an Image/Link to download the presentation.
The content on the website is provided AS IS for your information and personal use only. It may not be sold, licensed, or shared on other websites without obtaining consent from the author. If you encounter any issues during the download, it is possible that the publisher has removed the file from their server.
You are allowed to download the files provided on this website for personal or commercial use, subject to the condition that they are used lawfully. All files are the property of their respective owners.
The content on the website is provided AS IS for your information and personal use only. It may not be sold, licensed, or shared on other websites without obtaining consent from the author.
E N D
Presentation Transcript
Sample-optimal tomography of quantum states J eongwan Haah (MIT) Aram Harrow (MIT) Zhengfeng J i (ISCAS/Waterloo) Xiaodi Wu (MIT -> Oregon) Nengkun Yu (Guelph/Waterloo) QIPC Leeds 2015.9.28 arXiv:1508.01797
state tomography Goal: minimize loss, i.e. max E dist( , ) M classical description quantum states
how many copies? Distance measures Trace distance: = || - ||1 / 2 Infidelity: = 1 F( , ) = 1 || 1 /2 1 /2 ||1 2 n = f( ,d, r) n = g( ,d, r) copies necessary/sufficient. States d dimensions Assume rank r. How does n scale with d, r, , ?
boundary case: d=2 Estimation protocol Measure in {|0 , |1 } basis Let q := (#0 s) / n. output suppose distribution of q p n 1 / 2 1 /
Local Asymptotic Normality Kahn-Guta 0804.3876 -dependent covariance matrix implies optimal n f(d) / = g(d) / 2 for unknown f, g Bloch ball
boundary case: constant error Intuition has d2 - 1 real parameters n d2 bounded rank: rd parameters n rd # of copies # of parameters? plausible, but not a proof.
boundary case: r=1 Symmetry determines optimal measurement Fidelity and Trace distance are equivalent: F2+ 2 = 1 Explicit formula for all moments of F [Chiribella, 1010.1875]
our results related work [O Donnell-Wright, 1508.01907] ongoing work (speculative) product measurements [Kueng, Rauhut, Terstiege 1410.6913] product measurements
lower bound rank-r d-dim states form a manifold of dimension rd distance =0.1 packing net has size exp(rd)[Szarek 81] state estimation can transmit O(rd) bits
Lower bound (for r=d) An ensemble {pi, i} can transmit at most = S( i pi i) - i pi S( i) bits per copy [Holevo 73] Given an -net 1, ..., M, choose pi=1 /M, i= i n Choose an /10 net of states of the form S( i) =log(d)-O( 2) O(n 2) O(log M) d2 (from last slide)
Upper bound inspiration 1. Use symmetry, cf. spectrum estimation [Keyl-Werner 01] and rank-1 case [Holevo 79] 2. Use pretty-good measurement (PGM) [Belavkin 75] [Hausladen-Wootters 94]
symmetries of (Cd)n U2Ud! U U U U (Cd)4 = Cd Cd Cd Cd (1324)2S4! Schur-Weyl duality P
spectrum estimation n= q ( ) Im q irrep of GL(d) For d=2, analogous to J (total angular momentum). In general, spec( ) Measuring causes no disturbance. Thm: [Keyl-Werner, quant-ph/0102027] m tr q ( ) exp(-n D( || spec( )) nd2 n O(d2log(d/ ) / 2) for spectrum estimation substantial improvements by O Donnell-Wright, 1501.05028
pretty-good measurement [Belavkin 75] [Hausladen-Wootters 94] Given an ensemble {pi, i}, define Mi = -1 /2 pi i -1 /2 with = i pi i Classical analogue Given underlying distribution p(i), and observed j p(j|i), guess i with probability p(i |j)using Bayes rule. Thm: [Barnum-Knill, quant-ph/0004088] Pr[PGM correct] Pr[optimal measurement is correct]2 Thm: [Harrow-Winter, quant-ph/0606131] Given a set of M states with pairwise infidelity , PGM requires O(log(M)/ ) copies to distinguish w.h.p.
putting it together 1. First estimate spectrum using Keyl-Werner. Measurement yields estimate . Do PGM with { = U U : U uniform} lemma: m 2 tr q (U U ) F( ,U U )2n nrd 2. ...a little more algebra... thm: Pr[guess | ] F( , )2n nO(rd)
things we dont know Efficiency? Not even known for pure states. Process tomography Other prior distributions / assumptions about Adaptive measurements Continuous-variable tomography