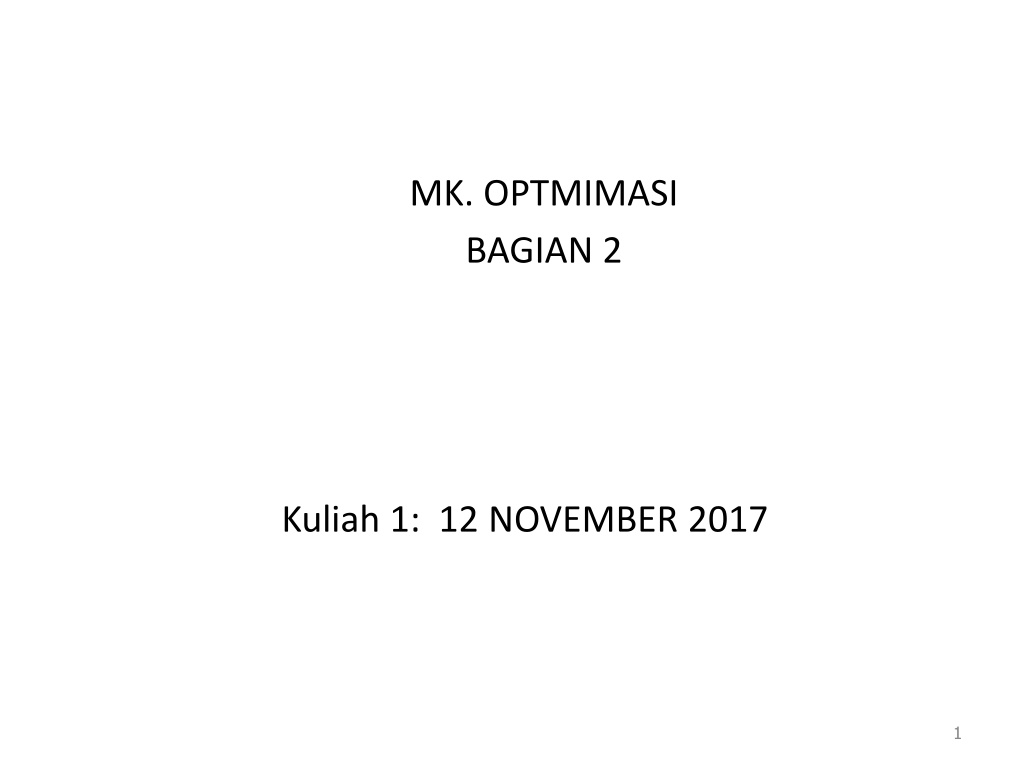
Non-Linear Optimization in Decision Making
Enhance logical and analytical problem-solving skills in non-linear optimization for decision making. Explore optimization terminology, classification of optimization problems, and various techniques for tackling complex decision-making scenarios.
Download Presentation

Please find below an Image/Link to download the presentation.
The content on the website is provided AS IS for your information and personal use only. It may not be sold, licensed, or shared on other websites without obtaining consent from the author. If you encounter any issues during the download, it is possible that the publisher has removed the file from their server.
You are allowed to download the files provided on this website for personal or commercial use, subject to the condition that they are used lawfully. All files are the property of their respective owners.
The content on the website is provided AS IS for your information and personal use only. It may not be sold, licensed, or shared on other websites without obtaining consent from the author.
E N D
Presentation Transcript
MK. OPTMIMASI BAGIAN 2 Kuliah 1: 12 NOVEMBER 2017 1
TUJUAN Meningkatkan kemampuan mahasiswa dalam berfikir secara logis analitis dalam penyelesaian masalah optimasi yang bersifat non linear. Setelah mengikuti mata kuliah ini mahasiswa diharapkan mempunyai kompetensi menyelesaian masalah optimasi non linear dan dapat merapkanya pada masalah nyata 2
DESKRIPSI ISI Masalah pengambilan keputusan selalu melibatkan penentuan pilihan terbaik dari semua alternative pilihan yang mungkin. Sehingga dalam pemilihan keputusan terbaik (optimal) diperlukan teknik2 khusus. Dalam perkuliahan ini dibahas metoda optimasi non linear dengan dan tanpa batasan. 3
Optimization Terminology An optimization problem or a mathematical program or a mathematical programming problem. Minimize f(x) subject to h(x)=c g(x)> b f(x): Objective function x: Decision variables h(x)=c: Equality constraint g(x)> b: Inequality constraint x*: solution 4
Classification of Optimization Problems Continuous Optimization Unconstrained Optimization Bound Constrained Optimization Derivative-Free Optimization Global Optimization Linear Programming Network Flow Problems Nondifferentiable Optimization Nonlinear Programming Optimization of Dynamic Systems Quadratic Constrained Quadratic Programming Quadratic Programming Second Order Cone Programming Semidefinite Programming Semiinfinite Programming Discrete and Integer Optimization Combinatorial Optimization Traveling Salesman Problem Integer Programming Mixed Integer Linear Programming Mixed Integer Nonlinear Programming Optimization Under Uncertainty Robust Optimization Stochastic Programming Simulation/Noisy Optimization Stochastic Algorithms Complementarity Constraints and Variational Inequalities Complementarity Constraints Game Theory Linear Complementarity Problems Mathematical Programs with Complementarity Constraints Nonlinear Complementarity Problems Systems of Equations Data Fitting/Robust Estimation Nonlinear Equations Nonlinear Least Squares Systems of Inequalities Multiobjective Optimization 5
Outline Unconstrained Optimization Functions of One Variable oGeneral Ideas of Optimization oFirst and Second Order Conditions oLocal v.s. Global Extremum Functions of Several Variables oFirst and Second Order Conditions oLocal v.s. Global Extremum Constrained Optimization Kuhn-Tucker Conditions Sensitivity Analysis Second Order Conditions Covex Programming 6 6
Unconstrained Optimization An unconstrained optimization problem is one where you only have to be concerned with the objective function you are trying to optimize. An objective function is a function that you are trying to optimize. None of the variables in the objective function are constrained. 7
General Ideas of Optimization There are two ways of examining optimization. Maximization (example: maximize profit) In this case you are looking for the highest point on the function. Minimization (example: minimize cost) In this case you are looking for the lowest point on the function. 8
Maximization f(x) is equivalent to minimization f(x) Fin500J Topic 4 Fall 2010 Olin Business School 9
Graphical Representation of a Maximum y 16 y = f(x) = -x2 + 8x x 4 8 10
Questions Regarding the Maximum What is the sign of f '(x) when x < x*? Note: x* denotes the point where the function is at a maximum. What is the sign of f '(x) when x > x*? What is f '(x) when x = x*? Definition: A point x* on a function is said to be a critical point if f ' (x*) = 0. This is the first order condition for x* to be a maximum/minimum. 11
Second Order Conditions If x* is a critical point of function f(x), can we decide whether it is a max, a min or neither? Yes! Examine the second derivative of f(x) at x*, f ' '(x*); x* is a maximum of f(x) if f ' '(x*) < 0; x* is a minimum of f(x) if f ' '(x*) > 0; x* can be a maximum, a minimum or neither if f ' '(x*) = 0; 12
An Example of f''(x*)=0 Suppose y = f(x) = x3, then f '(x) = 3x2 and f ''(x) =6x, This implies that x* = 0 and f ''(x*=0) = 0. y=f(x)=x3 y x x*=0 is a saddle point where the point is neither a maximum nor a minimum 13
Example of Using First and Second Order Conditions Suppose you have the following function: f(x) = x3 6x2 + 9x Then the first order condition to find the critical points is: f (x) = 3x2 - 12x + 9 = 0 This implies that the critical points are at x = 1 and x = 3. 4 2 0 -2 -4 -6 -8 14 -0.5 0 0.5 1 1.5 2 2.5 3 3.5 4
Example of Using First and Second Order Conditions (Cont.) The next step is to determine whether the critical points are maximums or minimums. These can be found by using the second order condition. f ''(x) = 6x 12 = 6(x-2) Testing x = 1 implies: f ''(1) = 6(1-2) = -6 < 0. Hence at x =1, we have a maximum. Testing x = 3 implies: f ''(3) = 6(3-2) = 6 > 0. Hence at x =3, we have a minimum. 15