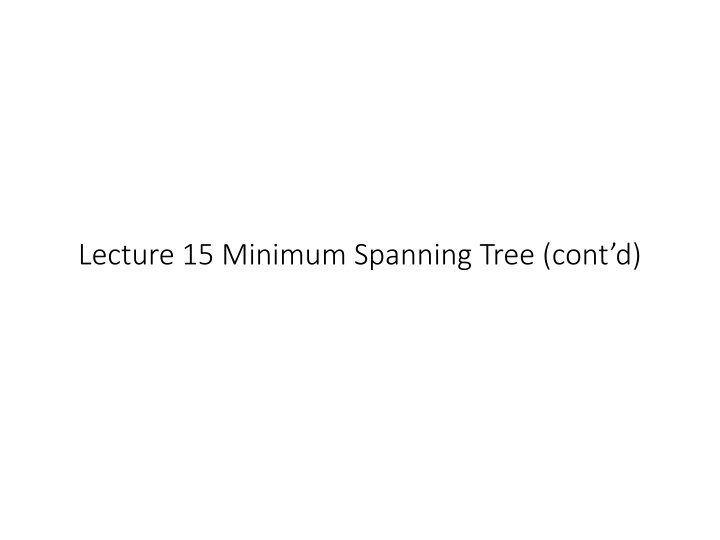
Minimum Spanning Tree Algorithms Explained
Learn about Minimum Spanning Trees (MST) and key concepts, such as cycles, key lemma, and algorithms like Prim's and Dijkstra's. Understand the process of designing an MST algorithm efficiently. Explore how to find the minimum cost edge, identify cuts, and implement algorithms step by step.
Download Presentation

Please find below an Image/Link to download the presentation.
The content on the website is provided AS IS for your information and personal use only. It may not be sold, licensed, or shared on other websites without obtaining consent from the author. If you encounter any issues during the download, it is possible that the publisher has removed the file from their server.
You are allowed to download the files provided on this website for personal or commercial use, subject to the condition that they are used lawfully. All files are the property of their respective owners.
The content on the website is provided AS IS for your information and personal use only. It may not be sold, licensed, or shared on other websites without obtaining consent from the author.
E N D
Presentation Transcript
Trees and cycles Adding any non-tree edge to a tree will introduce a cycle For a tree with a cycle, removing any edge in the cycle results in a tree. Basic operation: add an edge and remove another edge in the cycle created (swap).
Key Property Key Lemma: Suppose F is a set of edges that is inside some MST T, if ?, ? is a cut that does not contain any edge in F and e is the minimum cost edge in the cut, then it is safe to add e to F. ? ? ? for some MST T
General Algorithm for MST Initialize the tree to be empty. (initially a subset of some MST) Repeat Find a cut that does not have any edge in the current tree Add the min cost edge of the cut to the tree (by Key Lemma: still a subset of some MST) Until the tree has n-1 edges (Now we already have a tree, so it must be a MST)
Designing a MST algorithm Goal: do the two operations efficiently 1. How to find a cut that does not go through any edges we have chosen 2. How to find a minimum cost edge in the cut.
Prims algorithm Starting from an arbitrary vertex s. Make sure that the edges we select are always connected to s. Choosing the cut: The set of vertices connected to s. Finding the minimum cost edge: Use a data-structure similar to Dijkstra.
Prims algorithm Prim(s) initialize dis[u] to be all infinity, prev[u] to be NULL For neighbors of s, initialize dis[u] = w[s,u], prev[u] = s Mark s as visited FOR i = 2 to n Among all vertices that are not visited, find the one with smallest distance, call it u. Mark u as visited FOR all edges (u,v) IF w[u,v] < dis[v] THEN dis[v] = w[u,v] prev[v] = u.
Dijkstras algorithm Dijkstra(s) initialize dis[u] to be all infinity, prev[u] to be NULL For neighbors of s, initialize dis[u] = w[s,u], prev[u] = s Mark s as visited FOR i = 2 to n Among all vertices that are not visited, find the one with smallest distance, call it u. Mark u as visited FOR all edges (u,v) IF dis[u]+w[u,v] < dis[v] THEN dis[v] = dis[u]+w[u,v] prev[v] = u.
Kruskals algorithm Idea: don t try to find a cut, directly find the shortest edge. Algorithm: Sort the edges in ascending order of weight. For each edge, if adding it does not create a cycle, then add the edge to the tree. Checking the cycle needs a special data structure that we will talk about later.
Running time of Kruskal Sorting takes O(mlog m) time. For each edge, we need to check whether adding the edge creates a cycle. This can be done very efficiently (o(log m)). Total running time O(m log m)
Prim vs. Kruskal Running Time: Prim seems to be always faster O(m+nlog n) vs. O(m log m) However, the O(m+nlog n) version of Prim is not very easy to implement, and has a large hidden constant If you use a regular binary heap, the running time are the same, and Kruskal is usually faster in practice/easier to implement. If the graph is dense, O(n2) version of Prim is easy to implement and faster than Kruskal.