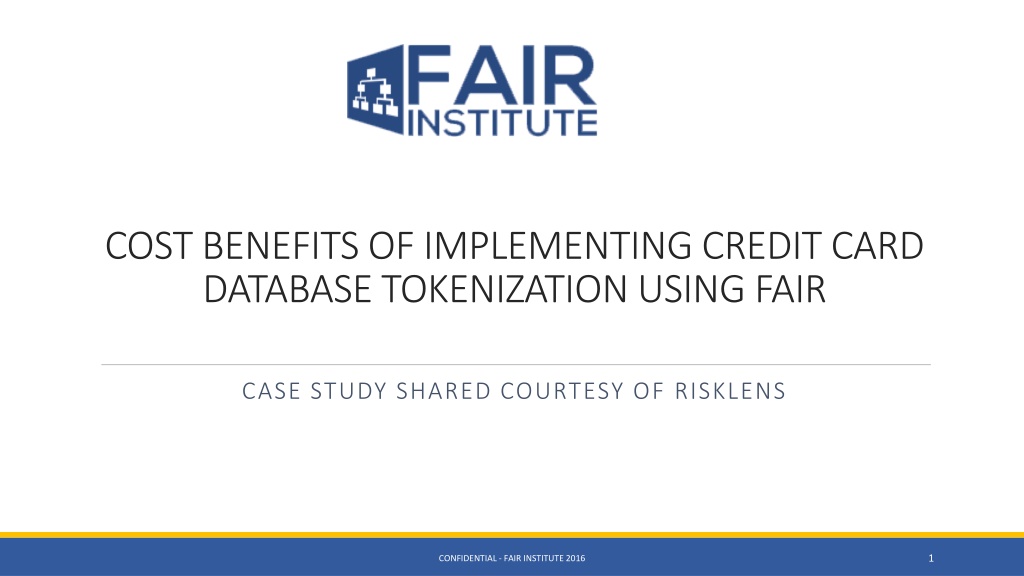
Implementing Credit Card Database Tokenization for Risk Reduction
This case study explores the cost benefits of implementing credit card database tokenization to reduce the risk associated with storing credit card data. By assessing the level of risk in different scenarios and interpreting the results, the study highlights the potential reduction in total loss exposure and per scenario magnitude through tokenization. The analysis focuses on mitigating threats to confidentiality posed by organized cyber criminals seeking to exploit stolen card data.
Download Presentation

Please find below an Image/Link to download the presentation.
The content on the website is provided AS IS for your information and personal use only. It may not be sold, licensed, or shared on other websites without obtaining consent from the author. Download presentation by click this link. If you encounter any issues during the download, it is possible that the publisher has removed the file from their server.
E N D
Presentation Transcript
COST BENEFITS OF IMPLEMENTING CREDIT CARD DATABASE TOKENIZATION USING FAIR CASE STUDY SHARED COURTESY OF RISKLENS 1 CONFIDENTIAL - FAIR INSTITUTE 2016
ANALYSIS SCOPING RISK SCENARIO DESCRIPTION Understand how much credit card number tokenization can reduce the risk associated with the card datastore ASSET(S) DESCRIPTION Credit card data in the Card Data Environment (CDE) LOSS TYPE Confidentiality THREAT(S) DESCRIPTION Organized cyber criminals motivated to monetize stolen card data through underground markets 2 CONFIDENTIAL - FAIR INSTITUTE 2016
ANALYSIS SCOPING Assessing Risk Reduction By Comparison of Scenarios: Assessed how much risk a critical datastore has when storing full credit card numbers Assessed level of risk for applications using the primary datastore Assessed how much risk the datastore will have using a tokenized form* *Assumption: There is still a low probability that tokenized data can be compromised by: Theft of the data in transit (potential Point-of-Sale attack) Compromised tokenization data source and/or process Both were considered in the analysis. 3 CONFIDENTIAL - FAIR INSTITUTE 2016
ANALYSIS SCOPING INTERPRET RESULTS: Look at the risk reduction from two perspectives: 1. Reduction in total loss exposure (annualized risk) 2. Reduction in per scenario magnitude 4 CONFIDENTIAL - FAIR INSTITUTE 2016
ANALYSIS RESULTS RISK = Frequency x Magnitude of future loss. We express risk in terms of loss exposure. ANNUALIZED REDUCTION IN LOSS EXPOSURE (RISK) 5 CONFIDENTIAL - FAIR INSTITUTE 2016
ANALYSIS RESULTS Change in Average Loss Exposure Range for Each Component FUTURE CURRENT 6 CONFIDENTIAL - FAIR INSTITUTE 2016
ANALYSIS RESULTS Change in Average Loss Exposure By Scenario CURRENT FUTURE 7 7 CONFIDENTIAL - FAIR INSTITUTE 2016
ANALYSIS RESULTS Change in Loss Exposure Category By Scenario Current Future 8 CONFIDENTIAL - FAIR INSTITUTE 2016
ANALYSIS LEVERAGED THE FAIR MODEL Risk Loss Event Frequency Loss Magnitude Threat Event Frequency Vulnerability Primary Loss Secondary Loss Contact Frequency Probability of Action Threat Capability Resistance Strength Loss Event Frequency Loss Magnitude 9 CONFIDENTIAL - FAIR INSTITUTE 2016
THE FAIR MODEL Risk Loss Event Frequency Loss Magnitude Threat Event Frequency Vulnerability Primary Loss Secondary Loss Contact Frequency Probability of Action Threat Capability Resistance Strength Loss Event Frequency Loss Magnitude 10 CONFIDENTIAL - FAIR INSTITUTE 2016
ANALYSIS CONSIDERATIONS Frequency of attacks by each threat community Estimating the capability (skills & resources) of the scoped threat community How vulnerable a given system is by evaluating the following factors: Authentication Access Privileges Patching / Structural Integrity 11 CONFIDENTIAL - FAIR INSTITUTE 2016
THE FAIR MODEL Risk Loss Event Frequency Loss Magnitude Threat Event Frequency Vulnerability Primary Loss Secondary Loss Contact Frequency Probability of Action Threat Capability Resistance Strength Loss Event Frequency Loss Magnitude 12 CONFIDENTIAL - FAIR INSTITUTE 2016
ANALYSIS INPUT PRIMARY LOSSES Incident response Investigation SECONDARY LOSSES Notification / credit monitoring Regulatory notification Possible fines / judgments Customer service requests Potential litigation Loss of current/future customers (reputation) Card replacement 13 CONFIDENTIAL - FAIR INSTITUTE 2016
DECISION SUPPORT / ROI THE ORGANIZATION WAS ABLE TO Quantify and compare the current and future risk exposure Fully justify and fund stalled tokenization projects across all credit card databases 14 CONFIDENTIAL - FAIR INSTITUTE 2016