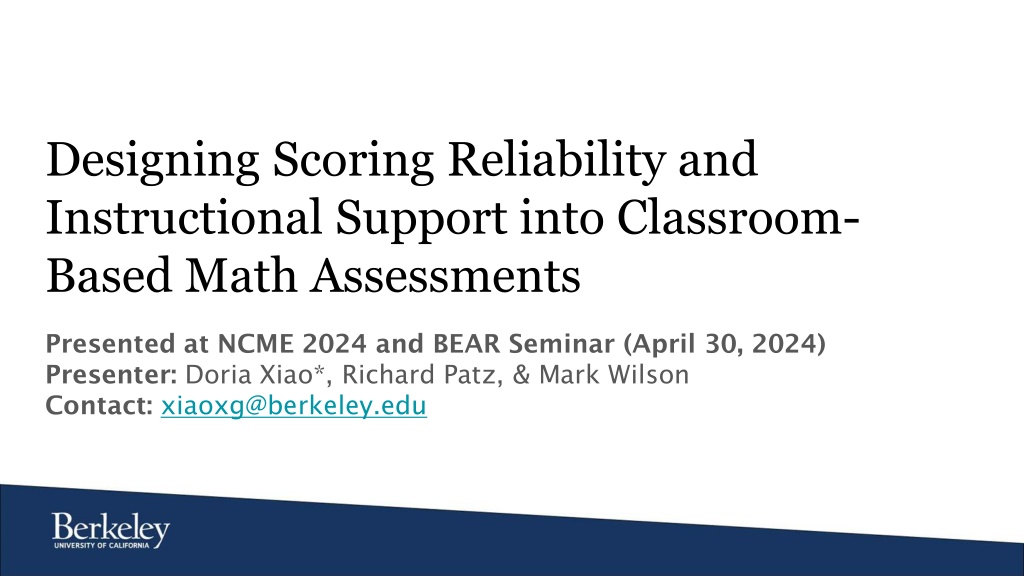
Enhancing Math Assessment Efficiency and Reliability
Explore the integration of scoring reliability and instructional support in classroom-based math assessments presented at NCME 2024 and BEAR Seminar. Topics include research questions, sample overview, scoring guide alignment with construct maps, and model results using Many-Facet Rasch Models. Learn about key objectives to improve student understanding and assessment accuracy, along with strategic response-rater assignment design. Discover insights into rating reliability and rater behavior in classroom settings.
Download Presentation

Please find below an Image/Link to download the presentation.
The content on the website is provided AS IS for your information and personal use only. It may not be sold, licensed, or shared on other websites without obtaining consent from the author. If you encounter any issues during the download, it is possible that the publisher has removed the file from their server.
You are allowed to download the files provided on this website for personal or commercial use, subject to the condition that they are used lawfully. All files are the property of their respective owners.
The content on the website is provided AS IS for your information and personal use only. It may not be sold, licensed, or shared on other websites without obtaining consent from the author.
E N D
Presentation Transcript
Designing Scoring Reliability and Instructional Support into Classroom- Based Math Assessments Presented at NCME 2024 and BEAR Seminar (April 30, 2024) Presenter: Doria Xiao*, Richard Patz, & Mark Wilson Contact: xiaoxg@berkeley.edu
Outline 1. Background & Research Questions 2. Overview of Sample, Items, and Activities 3. Scoring Guide Aligned with Construct Map 4. Response-Rater Assignment Design 5. Model & Results: Many-Facet Rasch Models (MFRM) for Polytomous Ratings 6. Summary 2
1. Exploring Key Research Objectives Enhancing alignment between the learning progression (construct map) and the items Improving accuracy in assessing students' mathematical understanding Implementing a strategic response-rater assignment design: Maximizing utilization of response data and human scoring for efficiency Statistical approach: Providing a methodological framework (MFRM) for classroom settings to gain deeper insights into rating reliability and rater behavior 3
2. Overview of Sample, Items, and Activities Items: Scored Activities: Two open-ended (OE) high-school items (scored by human raters) Wildlife Word 24 multiple-choice (MC) items (scored by machine) Activities conducted in Fall 2022 & Fall 2021 Total responses collected: 1304 Human-Scoring Activity: Date of human scoring: March 2023 Number of raters involved: 4 raters 4
3. Scoring Guide Aligned with Construct Map Utilization of construct maps for each sub-construct within the Interpreting Mathematical Results (IMR) construct Mapping each sub-construct to delineate levels of mathematical understanding (waypoints) Designing items to engage respondents at specific waypoints on the learning progression Providing raters with descriptors for waypoints to ensure consistent scoring 5
Sample Item: Wildlife 6
Construct Map | Scoring Guide No. The morning peak occurs at about 8am, the probability of wildlife-vehicle collisions increases as traffic volume increases before the morning peak. Then after the rush hours, the probability of wildlife-vehicle collisions decreases as traffic volume increases. IMR (Interpreting Mathematical Results) Her claim is incorrect because after a peak point before the middle of the X-axis the vehicle collision starts going down. No, it is not correct because the collisions drop as the volume increases Yes, because they intersect and have opposite slopes 7 no; Yes; umm
4. Response-Rater Assignment Design Randomized order of responses assigned to raters Data Collection Design: Fully crossed subsets: 100 responses scored by all 4 raters Overlapping block design: Each block consists of 100 responses and is scored by two different raters 8
Result: Percentage Agreement and Cohens Quadratic Weighted Kappa (QWK) Item 1: Wildlife Item 2: Word Human rater pair Number of responses Percentage agreement QWK/Kapp a Human rater pair Number of responses Percentage agreement QWK/Kapp a 2 100 58.0% 0.73 2 99 45.5% 0.35 1 3 100 68.0% 0.77 1 3 99 51.5% 0.28 4 148 76.4% 0.87 4 148 56.1% 0.36 3 150 58.0% 0.67 3 150 37.3% 0.17 2 2 4 99 59.6% 0.74 4 99 39.4% 0.36 3 4 149 63.1% 0.77 3 4 149 74.5% 0.45 QWK .70 is a widely adopted criterion in recent studies (McGraw-Hill Education CTB, 2014; Pearson and ETS, 2015; Williamson et al., 2012), including its application in math assessment (Lottridge, Wood, & Shaw, 2020; etc.) 10
5. Model : Many-Facet Rasch Models (MFRM) for Polytomous Ratings 11
Conclusion: The severity of raters appears to vary by item. 12
6. Discussion and Summary 1. Importance of Rubric Alignment with Learning Progressions a. Keeps measurement grounded in the construct b. Teacher involvement in scoring reinforces their understanding of learning progression 2. Response-Rater Assignment Design a. Efficiently allocate responses to raters to accurately assess inter-rater reliability and identify any discrepancies in ratings 3. Statistical Analysis Approach a. MFRM Model: Combining OE and MC items, aligning raters, item difficulties, and categories on a common scale to evaluate each rater's impact on item difficulties. 13