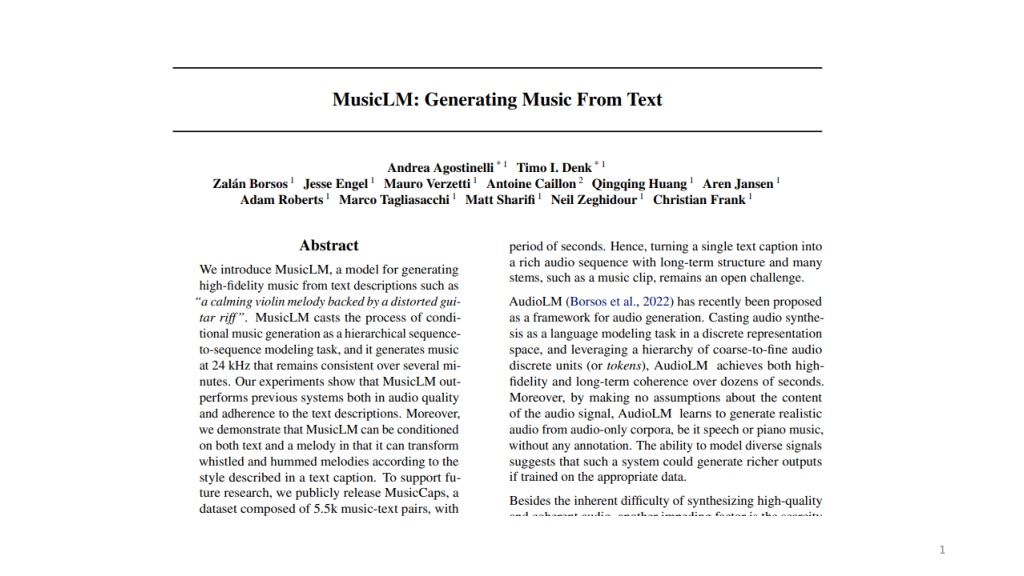
Cutting-Edge Technology in Audio-Visual Innovation
Explore the forefront of text-to-image and text-to-music generation, neural audio codecs, and joint embedding technologies for music and text. Discover how soundstream, w2v-BERT, and MuLan redefine audio processing through AI advancements. Understand the training steps and inference processes using state-of-the-art models for creating high-quality audio outputs from text prompts. Dive into the significance of acoustic and semantic stages in capturing intricate audio details and semantic information.
Download Presentation

Please find below an Image/Link to download the presentation.
The content on the website is provided AS IS for your information and personal use only. It may not be sold, licensed, or shared on other websites without obtaining consent from the author. If you encounter any issues during the download, it is possible that the publisher has removed the file from their server.
You are allowed to download the files provided on this website for personal or commercial use, subject to the condition that they are used lawfully. All files are the property of their respective owners.
The content on the website is provided AS IS for your information and personal use only. It may not be sold, licensed, or shared on other websites without obtaining consent from the author.
E N D
Presentation Transcript
SoundStream(Zeghidour et al., 2022) Neural audio codec Compress audio at lower bit rate Maintain high reconstruction quality(24 kHz) Residual Vector Quantization(RVQ) 6
w2v-BERT(Chung et al., 2021) BERT for audio(originally trained on speech) Take care of semantic information Model long-term structure 7
MuLan(Huang et al., 2022) Music-text joint embedding -> link music to free-form description Two embedding towers (audio + text) Text-embedding network is BERT pre-trained on text Audio-embedding network is ResNet-50 Trained on pairs of music clips and their corresponding text annotation 8
Training steps 1. Extract tokens from input a. acousitc tokens (A) with SoundStream b. semantic tokens(S) with w2v-BERT c. audio tokens(??) with MuLan 2. Predict semantic tokens conditioned on MuLan audio tokens:??->S 3. Predict acoustic tokens conditioned on MuLan audio tokens and semantic tokens:(??,S)->A 9
More on training Each stage is modelled autoregressively as a sequence-to- sequence task using decoder-only Tranformers 10
Inference 1. Provide text prompt 2. Extract MuLan text tokens?? 3. Predict semantic tokens conditioned on MuLan text tokens 4. Predict acoustic tokens conditioned on MuLan text tokens and semantic tokens 5. Reconstruct audio passing the acoustic tokens to the SoundStream decoder 11
Why acoustic and semantic stages? Acoustic model captures fine-grained acoustic details Semantic model improves long-term music structure+adherence to text descriptions 12
Clever tricks SoundStream made it possible to generate tokens instead of waveforms -> lower dimensionality MuLan made it possible to use audio dataset without text descriptions 13
Experiments Training data a. Pretrained and frozen MuLan model b. SoundStream and w2v-BERT trained on the Free Music Archive dataset c. Autoregressive models trained on 280k hours of music at 24 kHz d. Trained with 30- and 10-second audio clips a. Evaluation a. Quantitative and expert-based evaluations b. New dataset (MusicCaps)-> 5.5k music clips from AudioSet with text 14
Results MusicLM outperforms Riffusion and Mubert AI Better audio-fidelity Better adherence to text input 15
Limitations Long-term structure isn't convincing -> not evaluated Audio fidelity is OK but not ready for production Minimal creative control A tool for TikTokers not for high-end creators and musicians 16
THANKS! 17