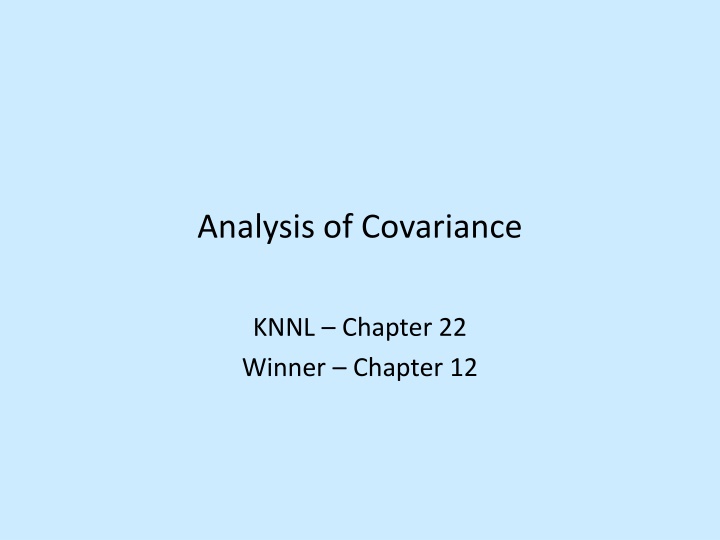
Advanced Analysis of Covariance Methods Explained
Explore the nuances of Analysis of Covariance (ANCOVA) in this comprehensive guide. Learn how to compare treatments while controlling for numeric predictors, understand single and multiple factor models, delve into additive and interaction models, and discover generalizations for handling random covariates in regression models.
Download Presentation

Please find below an Image/Link to download the presentation.
The content on the website is provided AS IS for your information and personal use only. It may not be sold, licensed, or shared on other websites without obtaining consent from the author. If you encounter any issues during the download, it is possible that the publisher has removed the file from their server.
You are allowed to download the files provided on this website for personal or commercial use, subject to the condition that they are used lawfully. All files are the property of their respective owners.
The content on the website is provided AS IS for your information and personal use only. It may not be sold, licensed, or shared on other websites without obtaining consent from the author.
E N D
Presentation Transcript
Analysis of Covariance KNNL Chapter 22 Winner Chapter 12
Analysis of Covariance Goal: To Compare treatments (1-Factor or Multiple Factors) after Controlling for Numeric Predictor(s) that is (are) related to response Makes use of Multiple Linear Regression Model with numeric and categorical predictors Covariates (aka Concomitant Variables) can not be effected by the treatments assigned to units (often covariate is pre-treatment or baseline score) Purpose is to reduce experimental error when it is large Alternative to blocking: uses fewer degrees of freedom, and can be measured after trt assignment
Single Factor Model with 1 Covariate = + + = = 1-Way ANOVA Model: 1,..., ; 1,..., Y i r j n ij i ij i r ( ) = 2 0 ~ 0, independent N i ij = 1 i Model with Centered Covariate: ( ) = + + + = = 1,..., ; 1,..., Y X X i r j n ij i ij ij i Fixed Constants (often pre-treatme nt measurements) X ij r ( ) = 2 0 ~ 0, independent N i ij = 1 i ( ) E Y Y = + + = 2 2 are independent X X Y ij i ij ij ij Effect of Treatment after controlling for i X i ( ) ( ) + + 2 ~ , independent Y N X X ij i ij
Additive Model Homogeneity of Slopes Analysis of Covariance - Additive Model 160 140 120 100 mu_1 80 Y mu_2 mu_3 60 mu_4 40 20 0 0 2 4 6 8 10 12 14 16 18 20 X = = = = = = = 100 10 10 20 20 2 10 X 1 + 2 3 4 ( ) E Y = + X X ij i ij
Interaction Model Heterogeneity of Slopes Analysis of Covariance - Interaction Model 140 120 100 80 mu_1 Y mu_2 60 mu_3 mu_4 40 20 0 0 2 4 6 8 10 12 14 16 18 20 X = = = = = = = = = = 100 10 10 20 20 2 4 0 1 10 X 1 + 2 3 4 1 2 3 4 ( ) E Y = + X X ij i i ij
Model Generalizations Random Xij - Model is treated as conditional of observed values of X Nonlinear relation between Response and Covariate Include linear and quadratic centered X values More than one covariate No problem extending to multiple covariates More than one treatment factor No problem having multiple factors
Regression Model for 1-Way ANCOVA Create Dummy Variables for Treatments and Centered Covariate: 1 if Treatment = 1 1 if Treatment = -1 1 if Treatment = ... 1 if Treatment = 0 otherwise 0 otherwise r = = = I r I r x X X 1 1 r ij ij Testing for Treatment Effects after Controlling for Covariate (Additive Model): = + + + + + Full Model: ... Y I I x 1 1 1 , 1 ij ij r ij r ij ij 2 n r ^ ^ ^ ^ i ( ) ( ) = + + + + = + ... ( ) F 1 SSE F Y I I x df n r 1 1 r 1 , 1 ij ij ij r ij E T = = 1 1 i j = + + Reduced Model: Y x ij ij ij 2 n r ^ ^ i ( ) = + = ( ) R 2 SSE R Y x df n ij ij E T = = 1 1 i j ( ) ( ) R SSE F df ( ) ( ) F SSE R df SSE F df ( ) ( ) R ( ) F ( ) F E E = * * Test Statistic: Conclude Treatment Means Differ if 1 ; , F F F df df df ( ) ( ) F E E E E
Comparing (Adjusted) Treatment Means Adjusted Mean for Treatment where line crosses at = =0: i X X x ij ij ^ ^ ^ ^ ^ = + = 1,..., 1: : ... i r i r 1 1 i r Comparing Pairs of Adjusted Means: ^ ^ , ' i i 1 Parameter: Estimator: r ' i i ' i i ^ ^ ^ ^ ^ ^ = + 2 2 Estima ted Standard Error: 2 , s s s s ' ' ' i i i i i i 1 r ^ ^ ^ ^ ^ ^ ^ = = = + ' Parameter: Estimator: ... 2 i r 1 1 ' i r i r i i i r = ' 1 ' i i i 1 1 2 1 r r r r ^ ^ ^ ^ ^ ^ ^ ^ = + + + 2 2 Estimated Standard Error: 4 4 , 2 , s s s s s ' ' ' '' i r i i i i i i = = = = + ' 1 ' ' 1 ' ' 1 '' ' i i ' 1 i i i i i i i i i '' i i Where the variances and covariances are obtained from: ( ) -1 X'X obtained from statistical packages MSE
Testing for Common Slopes Create Dummy Variables for Treatments and Centered Covariate: 1 if Treatment = 1 1 if Treatment = ... 0 otherwise 1 if Treatment = -1 1 if Treatment = 0 otherwise r = = = I r I r x X X 1 1 r ij ij Testing for Common Slopes: = + + + + + + + + Full Model: ... ... Y I I x I x I x 1 1 1 , 1 1 1 1 , 1 ij ij r ij r ij ij ij r ij r ij ij 2 n r ^ ^ ^ ^ ^ ^ i ( ) = + + + + + + + = ... ... ( ) F 2 SSE F Y I I x I x I x df n r 1 1 r 1 1 r 1 , 1 1 , 1 ij ij ij r ij ij ij ij r ij E T = = 1 1 i j = = + + + + + Reduced Model: ... Y Y I I x 1 1 1 , 1 ij ij ij r ij r ij ij 2 n r ^ ^ ^ ^ i ( ) ( ) = + + + + = + ... ( ) R 1 SSE R Y I I x df n r 1 1 r 1 , 1 ij ij ij r ij E T = = 1 1 i j ( ) ( ) R SSE F df ( ) ( ) F SSE R df SSE F df ( ) ( ) F ( ) ( ) R ( ) F ( ) F E E = * * Test Statistic: Conclude Slopes Differ if 1 ; , F F F df df df E E E E